NCBI Bookshelf. A service of the National Library of Medicine, National Institutes of Health.
Tipton K, Leas BF, Flores E, et al. Impact of Healthcare Algorithms on Racial and Ethnic Disparities in Health and Healthcare [Internet]. Rockville (MD): Agency for Healthcare Research and Quality (US); 2023 Dec. (Comparative Effectiveness Review, No. 268.)
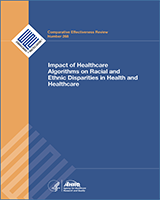
Impact of Healthcare Algorithms on Racial and Ethnic Disparities in Health and Healthcare [Internet].
Show detailsMain Points
- We examined two Key Questions (KQs). KQ 1 explored the effect of healthcare algorithms on racial and ethnic disparities in access to care, quality of care, and health outcomes. KQ 2 identified strategies to mitigate racial and ethnic bias associated with algorithms.
- For KQ 1, we identified 17 studies examining the effect of 18 algorithms on racial and ethnic disparities in health and healthcare. Four of the 18 algorithms included race or ethnicity as an input variable. The most frequently examined algorithms are used (or, in a few instances, are suggested for use) to inform resource allocation in a crisis setting (e.g., crisis standards of care) (4 studies), guide emergency department (ED) care decisions (3 studies), determine eligibility for lung cancer screening (3 studies), and determine eligibility for prostate cancer screening (2 studies). None was a randomized controlled trial (RCT).
- KQ 1 studies found that algorithms may reduce racial and ethnic disparities (5 studies), perpetuate or exacerbate disparities (11 studies; 3 of 11 included an examination of methods to mitigate these disparities and thus addressed both KQ 1 and 2), or have no effect on disparities (1 study). Three of the four studies examining algorithms that included race and ethnicity as an input variable found that these algorithms actually or potentially reduced disparities. In some algorithms (e.g., revised Kidney Allocation System), race and ethnicity input variables were included specifically to address existing racial and ethnic disparities.
- Studies addressing KQ 2 examined strategies for mitigating racial and ethnic disparities associated with algorithms. We included 44 studies across a range of clinical applications, including measurement of kidney function and lung function; risk assessment for cardiovascular disease, stroke, lung cancer, opioid misuse, and postpartum depression; suitability for kidney and liver transplant; and anticoagulation titration.
- Six types of mitigation strategies were identified: removing a race or ethnicity input variable from the algorithm (24 studies); replacing race or another input variable with a different measure (5 studies); adding an input variable (9 studies); recalibrating the algorithm with a more representative patient population (4 studies); stratifying algorithms to assess Black and White patients separately (2 studies); and using different statistical techniques within algorithms (3 studies).
- Most studies that examined the impact of removing a race coefficient from a common kidney function measure (eGFR) found an increase in diagnoses of chronic and severe kidney disease in Black patients. This may lead to a decrease in disparities in both early nephrology referrals for chronic kidney disease and referrals for kidney transplant. Conversely, some studies demonstrated a potentially negative impact of removing the race coefficient on health and healthcare outcomes other than transplant eligibility among Black patients when removing the race coefficient (e.g., patients reclassified as having more severe kidney disease could be deemed ineligible, possibly inappropriately, for medication or enrollment in clinical trials).
- Algorithms are often developed by electronic health record vendors, payers, and health systems. Due to the propriety nature of these algorithms, little is known about the development approach and potential impact on racial and ethnic disparities.
- Awareness is low among patients, healthcare providers, payers, and policymakers of the potential for algorithms to affect racial and ethnic disparities.
Background and Purpose
Healthcare algorithms are frequently used to guide clinical decision making at the point of care and as part of resource allocation and healthcare management. Race and ethnicity are often used as input variables in these algorithms.1–3 However, because race and ethnicity are socially constructed and thereby poor proxies for biological markers or genetic predisposition, when used in algorithms to guide clinical care their inclusion may introduce or exacerbate inappropriate, unequal treatment (healthcare disparities) and thereby contribute to or exacerbate unequal health outcomes (health disparities).4–6 In September 2020, the Agency for Healthcare Research and Quality received a request from Congress to review the evidence on the use of race and ethnicity in clinical algorithms and the potential of algorithms to contribute to disparities in healthcare. This review responds to that request by exploring two KQs addressing how healthcare algorithms affect racial and ethnic disparities in access to care, quality of care, and health outcomes. KQ 1 asks: What is the effect of healthcare algorithms on racial and ethnic differences in access to care, quality of care, and health outcomes? KQ 2 focuses on potential solutions: What is the effect of interventions, models of interventions, or other approaches to mitigate racial and ethnic bias in the development, validation, dissemination, and implementation of healthcare algorithms?
Four Contextual Questions (CQs) designed to capture insights on practical aspects of potential racial and ethnic bias were also examined. CQ 1 examines the scope of healthcare algorithms that explicitly include race and ethnicity as an input variable; CQ 2 summarizes recently emerging standards and guidance on how racial and ethnic bias can be prevented or mitigated during algorithm development and deployment; and CQ 3 explores various stakeholders’ awareness of and their perspectives on associations between algorithms, race and ethnicity, and healthcare. To respond to CQ 4, we conducted an in-depth evaluation of a sample of six healthcare algorithms, not previously evaluated in the published literature, to better understand how their design and implementation might contribute to racial and ethnic disparities.
Methods
We searched electronic databases (Embase®, MEDLINE®, PubMed®, and the Cochrane Library) from January 1, 2011, to February 7, 2023. Using predefined criteria and dual review, we screened all records for KQ 1 and KQ 2 and selected eligible studies that assessed one or both KQs. We included studies that examined actual outcomes among patients managed using algorithms as well as those that modeled potential effects of the use of algorithms in both real-world and synthetic datasets. Eligible studies were required to report on at least one of the following outcome categories: access to healthcare, quality of care, and health outcomes. We assessed studies’ methodologic risk of bias (ROB) using the Risk Of Bias in Non-randomized Studies of Interventions (ROBINS-I) tool and piloted an appraisal supplement with signaling questions (e.g., was a transparent rationale provided for including or removing race and ethnicity?) to assess racial and ethnic equity-related ROB. Using this tool, ROB ratings on each of seven domains are combined to generate an overall rating of Low, Moderate, or High ROB for each study. CQs 1–3 were addressed through supplemental literature reviews and conversations with Subject Matter Experts, Key Informants, and Technical Expert Panel members. For CQ 4, we evaluated, in-depth, the development approach, performance, and implementation of six algorithms not previously widely evaluated in the published literature for potential racial or ethnic bias.
Results
Fifty-eight studies met eligibility criteria. Fourteen studies addressed KQ 1, 41 studies addressed KQ 2, and 3 studies addressed both KQs and are presented in the results section for each KQ. For KQ 1, 17 studies examined 18 algorithms that inform decisions about ED care (3 studies), predict mortality to inform resource allocation in a crisis setting (e.g. crisis standards of care) (4 studies), predict future healthcare needs (1 study), allocate organs for transplant (2 studies), assess risk of lung cancer (3 studies), predict opioid misuse (1 study), predict risk of prostate cancer (2 studies), and predict risk of stroke (1 study). Four of the 18 algorithms included race or ethnicity as an input variable. All studies addressing KQ 1 were non-RCTs and were rated as moderate or high ROB due to concerns such as confounding, deviations from intended interventions, and missing data. Equity-based signaling questions changed domain-specific ROB in one instance (from Low to Moderate for the domain of bias due to selection of participants in one study) but did not change overall ROB for any KQ 1 studies. Most study designs employed a modeling approach to determine outcomes using either real-world or synthetic datasets for inputting into the algorithm. The studies found that algorithms may reduce racial and ethnic disparities (5 studies), perpetuate or exacerbate disparities (11 studies; 3 of these included an examination of methods to mitigate these disparities and thus addressed both KQ 1 and 2), or have no effect on disparities (1 study). Three studies examining algorithms that included race and ethnicity as an input variable found that these algorithms actually or potentially reduced racial and ethnic disparities. It is important to note that, in one of the three algorithms (the revised Kidney Allocation System), race and ethnicity input variables were included specifically to address existing racial and ethnic disparities.
For KQ 2, 21 of the 44 included studies focused on kidney function and evaluated efforts to mitigate potential harms associated with using the race correction in the estimation of glomerular filtration rate (eGFR). Seven studies examined algorithms that predict cardiovascular risk, four studies addressed kidney or liver donation or transplant, and three studies assessed algorithms that guide dosing of the anticoagulant warfarin. The remaining studies addressed the need for intensive or high-risk care management, assessment of lung function, and risk of stroke, lung cancer, postpartum depression, or opioid misuse. One study was a randomized controlled trial, 17 studies used cohort or pre-post designs, and 26 studies used modeling approaches. ROB was rated as Low for 8 studies, Moderate for 31 studies, and High for 5. In six studies, ROB ratings for individual domains of bias changed because of the equity-based signaling questions we added, but the overall ROB rating was changed in only one of these studies. The most common domain to receive a rating change (mostly from Low to Moderate) was bias in selection of study participants due to inconsistent reporting of racial and ethnic groups and inconsistent definitions and categories for race and ethnicity.
We identified six broad categories that describe the mitigation strategies used to address potential harms resulting from algorithms: removing an input variable (usually race and ethnicity) was used in 24 studies; replacing a variable with one or more different variables (5 studies); adding one or more input variables (9 studies); diversifying the racial and ethnic composition of the patient population used to train or validate an algorithm (4 studies); creating separate algorithms or thresholds for different populations (2 studies); and modifying the statistical or analytic techniques used for algorithm development (3 studies). Some studies compared more than one mitigation strategy. Evidence suggests that removing a race coefficient from eGFR may result in significantly more diagnoses of chronic and severe kidney disease among Black patients, which can then lead to increased eligibility for kidney transplant; however, this may also result in underuse or underdosing of other treatments. Further research is needed to better understand these implications across the wide range of outcomes and medical decisions that eGFR influences.
Although studies reported that mitigation approaches can improve algorithm calibration and may reduce disparities, they often relied on simulation and inference to estimate the effects of such strategies on patient outcomes. This may not adequately model potential biases occurring in algorithm translation, dissemination, and implementation, and further research is needed to quantify the real-world effects of using and modifying algorithms. Finally, we found the effectiveness of mitigation strategies is context-specific and may largely depend on the unique combination of algorithm, clinical condition, population, setting, and outcomes.
Findings from the CQs suggest the scope of algorithmic bias is difficult to quantify, but it clearly extends across the entire spectrum of medicine. Public awareness of healthcare algorithms and their potential effects on health and healthcare is very limited. We identified numerous efforts by regulatory, professional, and corporate stakeholders to develop standards for algorithms, often emphasizing the need for transparency, accountability, and representativeness.
Limitations
Our multipronged and multidisciplinary approach to conduct a comprehensive review of the use of algorithms and efforts to mitigate their potential contribution to racial and ethnic disparities enabled us to synthesize a broad array of evidence. Due to heterogeneity of included studies, conclusions about the effect of algorithms on exacerbating racial and ethnic disparities in health and healthcare outcomes varied across different clinical assessment areas. We included algorithms for many different clinical settings, and results in one setting do not necessarily apply to those in other settings. Furthermore, attempts to mitigate race disparities caused by algorithms are also highly context-specific. Included studies frequently used national datasets that typically provide a more representative distribution of races, yet some studies used an overly broad race categorization, such as White/Non-White, when presenting findings. While this may allow investigators the ability to study systemic racism, broadly speaking, there is often inadequate representation of specific racial and ethnic groups to identify subgroup-specific issues such as differences in effects across different populations. This is because virtually all “Non-White” people self-identify using more specific race designation(s); furthermore, most electronic health records and scoring systems use more specific designations. Other studies focused only on two races (e.g., Black/White); their results may be less relevant to those of other races, and often ethnicity was not specified by study authors (i.e., whether these categories include patients identifying as Hispanic/Latino).
The lack of studies evaluating the real-world effects of an algorithm or mitigation strategy is a limitation of the current evidence base. Only 7 of 58 studies (3 for KQ 1 and 4 for KQ 2) actually managed patients with an algorithm or reported real outcomes experienced by patients. The rest of the studies used outcome simulation, whereby the authors estimated an algorithm’s (or mitigation strategy’s) hypothetical influence. The applicability of such studies depends heavily on assumptions made, representativeness of the data sources analyzed, and whether the algorithm would actually be used in the manner hypothesized. Simulation may, however, provide the basis for future hypothesis-driven clinical research into the effects of algorithms on racial and ethnic differences.
Implications and Conclusions
Algorithms have been shown to potentially exacerbate, perpetuate, or reduce racial and ethnic disparities in health and healthcare outcomes. When race or ethnicity were incorporated into an algorithm to intentionally tackle known disparities in resource allocation (e.g., kidney transplant allocation) or healthcare delivery (e.g., prostate cancer screening historically led to Black men receiving more low-yield biopsies), disparities were reduced. However, when race or ethnicity were included without a clear and appropriate rationale, incorrect notions of race as biological may be reinforced; moreover, algorithms that inappropriately used race and ethnicity as a proxy for biological mechanisms have been shown to potentially perpetuate and exacerbate disparities (e.g., eGFR for kidney function measurement). Furthermore, some algorithms do not contain race or ethnicity as an input but could also affect disparities. Several modeling studies showed that applying algorithms out of context of original development (e.g., illness severity scores used for crisis standards of care) would exacerbate disparities. Conversely, algorithms may also reduce disparities by standardizing care (e.g., Lung Allocation Score for lung transplantation). In terms of strategies to mitigate racial and ethnic disparities associated with algorithms, many studies presented proximal outcomes, such as improvements of algorithmic accuracy within a single racial group, resulting in the need to infer or extrapolate effects on differences between racial and ethnic groups. No single strategy led to the greatest success, but several have been shown to successfully mitigate disparities. Results may be highly context-specific, relating to unique combinations of algorithm, clinical condition, population, setting, and outcomes.
Finally, we emphasize the challenge of determining cause and effect in this literature. Disparities in health and healthcare are well documented for BIPOC (Black, Indigenous, or People of Color) people, but assessing how, and how much, a particular algorithm may contribute to or redress a disparity needs to be assessed. Distal health outcomes are also influenced by multiple contributing clinical, health system and social factors. Important future steps include increasing transparency in algorithm development and implementation, increasing diversity of research and leadership teams, engaging diverse patient and community groups in the development to implementation lifecycle, promoting awareness by stakeholders (including patients) of potential algorithmic risk, and investing in real-world experiments to assess the effect of algorithms on racial and ethnic disparities before widespread implementation.
References
- 1.
- Vyas DA, Eisenstein LG, Jones DS. Hidden in plain sight - reconsidering the use of race correction in clinical algorithms. N Engl J Med. 2020;383(9):874–82. doi: 10.1056/NEJMms2004740. PMID: 32853499. [PubMed: 32853499] [CrossRef]
- 2.
- Cerdeña JP, Plaisime MV, Tsai J. From race-based to race-conscious medicine: how anti-racist uprisings call us to act. Lancet. 2020;396(10257):1125–8. doi: 10.1016/s0140-6736(20)32076-6. PMID: 33038972. [PMC free article: PMC7544456] [PubMed: 33038972] [CrossRef]
- 3.
- Schmidt IM, Waikar SS. Separate and unequal: race-based algorithms and implications for nephrology. J Am Soc Nephrol. 2021;32(3):529–33. doi: 10.1681/asn.2020081175. PMID: 33510038. [PMC free article: PMC7920170] [PubMed: 33510038] [CrossRef]
- 4.
- Eneanya ND, Yang W, Reese PP. Reconsidering the consequences of using race to estimate kidney function. JAMA. 2019;322(2): 113–4. doi: 10.1001/jama.2019.5774. PMID: 31169890. [PubMed: 31169890] [CrossRef]
- 5.
- Obermeyer Z, Powers B, Vogeli C, et al Dissecting racial bias in an algorithm used to manage the health of populations. Science. 2019;366(6464):447–53. doi: 10.1126/science.aax2342. PMID: 31649194. [PubMed: 31649194] [CrossRef]
- 6.
- Amutah C, Greenidge K, Mante A, et al Misrepresenting race - the role of medical schools in propagating physician bias. N Engl J Med. 2021;384(9):872–8. doi: 10.1056/NEJMms2025768. PMID: 33406326. [PubMed: 33406326] [CrossRef]
- Executive Summary - Impact of Healthcare Algorithms on Racial and Ethnic Dispari...Executive Summary - Impact of Healthcare Algorithms on Racial and Ethnic Disparities in Health and Healthcare
Your browsing activity is empty.
Activity recording is turned off.
See more...