NCBI Bookshelf. A service of the National Library of Medicine, National Institutes of Health.
Tipton K, Leas BF, Flores E, et al. Impact of Healthcare Algorithms on Racial and Ethnic Disparities in Health and Healthcare [Internet]. Rockville (MD): Agency for Healthcare Research and Quality (US); 2023 Dec. (Comparative Effectiveness Review, No. 268.)
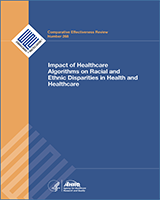
Impact of Healthcare Algorithms on Racial and Ethnic Disparities in Health and Healthcare [Internet].
Show details3.1. Overview
To address the Key Questions (KQs), electronic searches for published scientific studies identified 11,500 citations. After we screened titles and abstracts, 336 articles were deemed eligible for full-text review and evaluated for KQ 1 and/or KQ 2 eligibility. After full-text review, 58 articles met inclusion criteria:
Figure 4 presents a PRISMA (Preferred Reporting Items for Systematic Reviews and Meta-Analyses) flow diagram of study eligibility. The primary reasons for exclusion included the following: did not examine an algorithm (KQ 1), did not examine an intervention’s ability to mitigate racial and ethnic bias of an algorithm (KQ 2), and did not report an outcome of interest (both KQs). Detailed results of the literature searches and excluded studies are in Appendixes A and B. Two submissions were received through the Agency for Healthcare Research and Quality (AHRQ) Supplemental Evidence and Data submission process but did not meet eligibility criteria. Detailed characteristics and outcomes of included studies are described in Appendixes C and D.
To address the Contextual Questions (CQs), we synthesized insights gathered during semi-structured interviews with 14 Key Informants (KIs), 10 members of our Technical Expert Panel (TEP), and 5 Subject Matter Experts (SMEs). We also summarized key points from 10 white papers, commentaries, and technical documents that our searches identified; these resources describe guidelines, standards, and best practices to reduce potential racial and ethnic bias related to algorithm development. For CQ 4, we examined six algorithms in depth.
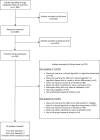
Figure 4
Study flow diagram.
Figure 5 and Figure 6 present an evidence map that summarizes the algorithms identified for KQ 1 and KQ 2. We include information about the type of clinical assessment and each study’s design and categorized outcomes as defined a priori in our protocol. For each algorithm, we display the primary outcome(s) and directionality of impact on disparities as identified by the study. The direction of effect on the outcome of interest is represented by an arrow pointing up (an increase), down (a decrease), or a horizontal arrow (no effect). Further details on findings presented in the evidence map can be found in the KQ 1 and KQ 2 Summary of Findings.
3.2. Key Question 1. What is the effect of healthcare algorithms on racial and ethnic differences in access to care, quality of care, and health outcomes?
3.2.1. Description of Included Evidence
For KQ 1, we included 17 studies: 11 modeling studies using real-world datasets,5,54–57,60,65–67,107,108 3 pre-post studies,58,61,62 2 retrospective cohort studies,63,64 and 1 modeling study using synthetic datasets.59 Most research was recent: 12 of 17 studies (71 percent) were published in 2021 or later.54,56–58,63–67,107–109
Studies in our evidence base examined algorithms that inform decisions about emergency department (ED) care,58,63,64 measure severity of illness for crisis standard-of-care scenarios,54,57,65,107 predict future healthcare needs,5 allocate organs for transplant,61,62 assess risk of lung cancer,59,67,109 predict opioid misuse,108 predict risk of prostate cancer,56,60 and predict risk of stroke66. Some studies analyzed data from patients who were managed using an algorithm and examined differences in outcomes across racial and ethnic groups in real-world settings. Most studies employed a modeling approach, using patients who were not managed using the algorithm but who have data on all the input variables included in the algorithm, making it possible to determine what the algorithm’s predictions and clinical recommendations would have been if applied to those patients. Eleven studies compared two or more algorithms,5,54,56,57,59,60,66,67,107–109 three studies compared an algorithm with no algorithm (e.g., pre-implementation of algorithm),58,61,62 and three studies examined algorithms in isolation, with no comparators.63–65 Detailed information about the included studies is provided in Table C-1 in Appendix C and Table D-1 in Appendix D.
For KQ 1, studies had to report outcome data separately for more than one racial and ethnic group. Studies usually identified and selected patients from electronic health records (EHRs) or national databases (e.g., transplant registries); therefore, the reliability of race and ethnicity classifications depended on respective database collection methods. In 11 studies, participants self-reported race and ethnicity,5,57,58,60,62–67,108 and the remaining studies did not specify how race or ethnicity was determined.54,56,59,61,107,109 In five studies, analyses were restricted to patients categorized as African-American/Black or White,5,54,62,63,107 and nine reported data for these two groups in addition to patients categorized as Asian or Hispanic;56,57,59,61,65–67,108,109 One study reported only analyses comparing non-Hispanic White patients with non-White patients.64 Eight studies included a heterogeneous non-White or Other patient group.56,58,60,64,65,67,108,109 In some studies, either the database itself used an “Other” category or the authors chose to create this category for patients whose race and ethnicity was unknown (e.g., patients declined to respond) or who belonged to racial and ethnic groups with small sample sizes.
Overall risk of bias (ROB) ratings were Moderate in 12 studies5,54,56,59,60,62–65,67,107,108 and High in 5 studies.57,58,61,66,109 Equity-based signaling questions changed domain-specific ROB in only one instance (from Low to Moderate for bias due to selection of participants in one study)64 and did not change overall ROB for any KQ 1 studies (Appendix Table D-3). In most studies, the algorithms examined were applied retrospectively to a single cohort of patients to model the effect of using the algorithm. In studies of this design, estimates of overall differences in the effects of one algorithm versus another are, by definition, not subject to ROB arising from confounding or selection of participants (because the same patients simultaneously “receive” both algorithms) or to ROB due to deviations from intended interventions (because both algorithms are “applied” without deviation). However, other estimates – notably, estimates of differences in outcome between racial and ethnic groups – may be subject to ROB arising from confounding or selection of participants; thus, conclusions about the effect of different algorithms on such racial and ethnic differences may be subject to increased ROB. Furthermore, estimates of effects in modeling studies may have limited generalizability to actual clinical settings due to the inability to estimate the impact of real-world deviations from intended interventions (i.e., use of algorithm at the point of care). Studies varied in procedures used to gather race and ethnicity of enrolled patients, such as being self-reported or captured by an administrator. For most studies, it was unclear whether a consistent definition of race and ethnicity was used or if adequate response options were available. In addition, some studies reported outcomes for aggregate groups, such as those that identify as BIPOC (Black, Indigenous, or People of Color), likely resulting in missing data for some racial and ethnic groups. Other ROB concerns centered on problems related to missing data for algorithm score generation or outcomes (e.g., safety events) and variation in methods to measure outcomes (e.g., across different time periods).
Eighteen different clinical algorithms were examined across the studies included for KQ 1. Four algorithms included race and ethnicity as an input variable,56,60,61,67 and 14 did not.5,54,57–59,62–66,107–109 Five studies described 4 algorithms that were associated with reduced racial and ethnic disparities,56,60–62,66 eleven studies found that 13 algorithms were associated with perpetuating or exacerbating racial and ethnic disparities (3 of 11 studies included an examination of methods to mitigate these disparities and thus addressed both KQ 1 and 2),5,54,57,59,63–65,67,107–109 and 1 study reported no racial and ethnic disparities with or without the algorithm.58 Three studies of algorithms that included race and ethnicity as an input variable found that disparities were reduced.56,60,61 It is important to note that, in one of the three algorithms (the revised Kidney Allocation System), race and ethnicity input variables were included specifically to address existing racial and ethnic disparities. Lastly, other algorithms that included race and ethnicity input variables and perpetuated and exacerbated disparities (i.e., eGFR [estimated glomerular filtration rate], GLI [Global Lung Function Initiative] calculator for spirometry) are described below in the KQ 2 results rather than here, because the studies examining them focused primarily on reporting mitigation strategies.
Table 4
Effect of clinical algorithms on racial and ethnic disparities.
3.2.2. Key Points
3.2.2.1. Emergency Department Assessment
- One study58 assessed the impact of implementing the HEART (History, Electrocardiogram, Age, Risk Factors, Troponin) Pathway risk assessment. In this algorithm, which does not include race or ethnicity, high scores indicate the patient is at high risk for adverse outcomes resulting from acute coronary syndrome and should receive further testing. After implementation, early discharge rates increased, and hospitalization and objective cardiac testing rates decreased for BIPOC (i.e., Black or African American, Asian, American Indian, and Hawaiian or Pacific Islander, and other/unknown patients) and White patients. BIPOC patients were more likely to be classified as low risk than White patients. The difference in 30-day death and myocardial infarction rates between low-risk BIPOC and White patients was nonsignificant; therefore, authors concluded that the pathway did not impact health disparities.
- Two studies63,64 examined the Emergency Severity Index (ESI), an algorithm triage providers use to assess a patient’s level of acuity and to prioritize care. An assigned ESI score is based on an assessment of a patient’s vital signs, primary reason for ED visit, and immediate needs. Race and ethnicity are not included as input variables. In a cohort of BIPOC and non-Hispanic (NH) White pediatric patients, one study examined the association between assigned ESI scores and the patient’s race.64 The second study assessed the effect of a rapid triage fast track (FT) model, an algorithm-informed care pathway based on ESI scores, on outcomes in NH Black and NH White adult patients.63 Results of both studies indicated that BIPOC pediatric and adult patients were more likely than NH White patients to receive a lower acuity score indicating a less urgent need for care. Using the ESI alone or to inform a care pathway, may lead to placing BIPOC patients in a lower acuity care category compared with NH White patients at the same level of need, which may potentially exacerbate racial and ethnic disparities in access to care. Authors noted that BIPOC patients might present to the ED with less acute conditions than NH White patients. Thus, lower ESI scores may reflect true differences in illness severity between patients upon presentation to the ED as opposed to the impact of provider assessment to determine acuity level and care needs.
3.2.2.2. High-Risk Care Management
- One study5 analyzed a widely used EHR-based algorithm (which does not include race or ethnicity as an input variable) designed to help determine whether patients should be placed in high-risk care management programs. The algorithm predicts future healthcare costs based on prior utilization; patients with high predicted costs are prioritized for placement in the programs. At any given level of actual healthcare need, the algorithm predicted lower costs for Black patients than for White patients, which could result in a race disparity in access to care.
3.2.2.3. Kidney Transplant Allocation
- One study61 examined how implementing the revised Kidney Allocation System (KAS), which includes ethnicity as an input variable, affected the rate of waitlisting (i.e., placement on the national deceased donor waiting list). After implementation, the overall rate of waitlisting declined for all racial and ethnic groups, due largely to a reduction in inactive waitlisting (i.e., placing patients on the waitlist who are not in fact eligible for transplant for various reasons). The difference in waitlisting rates between Black and White patients was reduced after implementation, due partly to declines in inactive waitlisting and increases in active waitlisting among BIPOC (Black, Hispanic, and Asian) patients, but a difference in rates remained. Therefore, implementing the revised KAS reduced racial and ethnic disparities.
3.2.2.4. Lung Transplant Allocation
- One study62 examined how implementing the Lung Allocation Score, which does not include race or ethnicity as input variables, affected outcomes for patients on the waitlist for lung transplantation. Before implementation, Black patients were more likely than White patients to die while on the waitlist or become too sick for transplantation within 3 years of listing (43.8 percent vs. 30.8 percent); after implementation, the rate of this outcome was reduced for both groups, and the difference between them became negligible (14.0 percent vs. 13.3 percent).
3.2.2.5. Lung Cancer Screening
- Two studies59,109 examined the U.S. Preventive Services Task Force (USPSTF) lung cancer screening guidelines from 2013 and compared them with an algorithm (PLCOm2012) for determining eligibility for lung cancer screening. The algorithm includes race as an input variable; USPSTF guidelines include only age and smoking history. Results indicated that race differences in eligibility based on the USPSTF guidelines would be greatly reduced by expanding eligibility criteria to include individuals categorized as eligible by PLCOm2012.
- One study67 examined racial and ethnic differences in the percentages eligible for lung cancer screening based on: (a) 2013 USPSTF guidelines; (b) the expanded 2021 USPSTF guidelines; (c) lung cancer risk as calculated by the PLCOm2012(Race3L) model (similar to the original PLCOm2012 but using 3-level race), using a value of 1.5 percent 6-year risk as the threshold for eligibility; and (d) PLCOm2012(Race3L) risk using a 1 percent threshold value. All four sets of criteria resulted in differences across racial and ethnic groups in percentages identified as eligible, with lower percentages among Black and Hispanic individuals than among White individuals and those of other races. Authors suggest that closing the gap between the proportions eligible by race might require inclusion of additional risk factors in risk-based lung cancer screening tools.
3.2.2.6. Opioid Misuse Risk
- One study108 of a natural-language-processing classifier designed to identify individuals needing services to help them overcome opioid abuse found a higher false-negative rate (i.e., patients who were misusing opioids but were not identified as such by the classifier) for Black patients (32 percent) and “Other” race and ethnicities (33 percent) than White or Hispanic/LatinX patients (17 percent), which could result in a racial and ethnic disparity in access to care for opioid misuse.
3.2.2.7. Prostate Cancer Risk
- Two studies56,60 modeled the use of prostate cancer algorithms (both including race as an input variable) to inform the decision about whether to perform a biopsy. Under some model parameters, the net benefit of algorithms (defined in terms of unnecessary biopsies that would have been avoided versus clinically significant cancers that would have been missed) was higher for White patients than for Black patients, but this depended on which algorithm was used as well as the numerical threshold for recommending biopsies; other parameters led to a slightly higher net benefit for Black patients than for White patients.
3.2.2.8. Severity of Illness Measurement for Crisis Standards of Care
- Three studies54,57,107 evaluated racial and ethnic differences in the performance of four illness-severity prediction models: Acute Physiology and Chronic Health Evaluation (APACHE IVa), Laboratory-based Acute Physiology Score version 2 (LAPS2), Oxford Acute Severity of Illness Score (OASIS), and Sequential Organ Failure Assessment (SOFA). In resource-constrained settings, these algorithms (none of which contain race or ethnicity as input variables) were proposed to be used to inform Crisis Standards of Care, which allocate resources preferentially to patients with better estimated chances of survival. In all three studies, the prediction models overestimated mortality in Black patients compared with White patients (i.e., at any given level of algorithm-predicted risk, Black patients had lower actual mortality than White patients). Using these prediction models therefore has the potential to lead to inappropriate deprioritization of Black patients.
- One study65 examined a Crisis Standards of Care algorithm based on short-term mortality risk estimated by SOFA and long-term mortality risk estimated either by comorbidities or physician assessment and estimated excess deaths that might occur through use of this algorithm to allocate ventilators under conditions of resource shortage, by race. At certain risk-threshold values, the estimated excess mortality among Black patients was significantly higher than that among non-Black patients.
3.2.2.9. Stroke Risk
- One study66 examined potential racial and ethnic disparities in health outcomes that could occur as a result of using an algorithm-informed decision tool, the American College of Cardiology/American Heart Association atrial fibrillation treatment guideline. The guideline recommendation for anticoagulant therapy is based on the CHA2DS2-VASc score, which predicts stroke risk in patients with atrial fibrillation. Race and ethnicity are not included as input variables. The study compared two versions of the guideline that use different CHA2DS2-VASc score thresholds to guide decision making. Using the 2014 guideline (CHA2DS2-VASc score > 1), among patients who would not have been offered anticoagulant therapy, 3.3 percent of Hispanic patients had a stroke. Using the 2020 guideline (CHA2DS2-VASc score > 2 for males and > 3 for females), 1.78 percent of Hispanic patients had a stroke. Authors suggest that when using the 2020 guideline, the disparity in negative event frequency (stroke) in Hispanic patients was reduced.
3.2.3. Summary of Findings
Below, we present the findings in the following clinical categories:
- High-risk care management5
- Kidney transplant allocation61
- Lung transplant allocation62
- Opioid misuse risk108
- Stroke risk66
Tables 5–15 describe the algorithms within each clinical category.
3.2.3.1. Emergency Department Assessment
Table 5
Description of the HEART Pathway.
One study assessed the impact of implementing the HEART Pathway risk assessment over 24 months in 3 North Carolina EDs among White patients and non-White (BIPOC) patients (Table C-1 in Appendix C).58 The BIPOC group included Black or African American, Asian, American Indian, and Hawaiian or Pacific Islander patients and a group of patients categorized as other/unknown (e.g., refused to provide information). Using an EHR database, the study examined data from 8474 White and BIPOC patients (n=3713 pre-implementation and n=4761 post-implementation). For several risk factors (e.g., cardiovascular disease), BIPOC patients already had lower rates than White patients before implementation.
In interpreting this study’s findings, it should first be noted that the HEART Pathway identified significantly more BIPOC patients as low risk than White patients (35.6 percent vs. 28.0 percent; p<0.0001). But clinical outcomes, including death, were not higher among low-risk BIPOC patients; therefore, the authors agreed that patients were accurately classified as low risk. Before HEART Pathway implementation, 30-day death or myocardial infarction rates were higher for White patients than for BIPOC patients, as were 30-day rates of hospitalization and objective cardiac testing. (Reduction in objective cardiac testing was a goal of the HEART Pathway, due to a high rate of unnecessary testing.) Post-implementation, hospitalization and objective cardiac testing rates decreased for both racial and ethnic groups, but the decrease was greater among BIPOC patients, while 30-day death or myocardial infarction rates decreased for BIPOC patients and increased for White patients. Thus, for these three outcomes, race differences increased post-implementation, with the difference-of-differences ranging from 1 to 5 percentage points (Table D-1 in Appendix D). For several adverse outcomes, BIPOC patients already had lower rates than White patients before implementation, and the disparities increased post-implementation. The rate of early discharge (proportion of patients discharged from the ED without objective cardiac testing, an outcome that the authors considered clinically appropriate) before implementation was lower for White patients than for BIPOC patients (36 percent vs. 40 percent). After HEART Pathway implementation, these rates were 39 percent for White patients and 49 percent for BIPOC patients. Although race differences increased from pre- to post-implementation for all four outcomes, the increase was statistically significant only for early discharge. Therefore, the authors concluded that implementing the HEART pathway did not worsen disparities for clinical outcomes, specifically 30-day death and myocardial infarction rates, and can be safely implemented. Authors suggested that pathway users should be cognizant that BIPOC patients are more likely to be classified as low-risk and therefore discharged early. This study did not capture long-term outcomes.
Table 6
Description of the Emergency Severity Index.
One study examined the association between assigned ESI scores and the patient’s race.64 The study used an EHR to identify 8928 pediatric patients (3086 NH White; 5842 Non-White) with 10,815 ED visits. Authors categorized patients as non-White if they reported any race other than White/Caucasian (American Indian/Alaska Native, Asian, Black, Native Hawaiian/Pacific Islander, other, and patients with more than one race).
In analyses adjusting for illness severity (i.e., abnormal vital signs), non-White pediatric patients were significantly less likely than NH White pediatric patients to receive an ESI score of 2 (emergency; adjusted odds ratio [aOR] 0.40, 95 percent confidence interval [CI]: 0.33 to 0.49, p<0.001) or 3 (urgent; aOR 0.50, 95 percent CI: 0.45 to 0.56, p<0.001), but significantly more likely to receive an ESI score of 5 (minor; aOR 1.34, 95 percent CI 1.07 to 1.69, p=0.012). That is, non-White patients were more likely than NH White patients to be assigned a lower acuity score (e.g., ESI 5), indicating a less urgent need for care. Subgroup analyses of the symptoms that caused patients to seek care (e.g., fever, headache) demonstrated similar results. For the outcome of ED length of stay, non-White patients had a higher discharge rate than NH White patients (adjusted hazard ratio [aHR] 1.08, 95 percent CI 1.03 to 1.14, p=0.002); the differences between racial and ethnic groups in time to provider (p=0.352) and hospital admission rates (p=0.094) were not significant. The authors stated that the observed pattern of results is consistent with the possibility that illness severity was inadequately controlled for – that is, there may have been a tendency, not fully adjusted for in the analyses, for non-White patients to present to the ED with less acute conditions than NH White patients. This explanation, if true, would lead one to expect that non-White patients would have lower ESI scores on average than NH White patients (as a reflection of objective differences in illness severity rather than algorithmic bias), but would not necessarily be undertreated compared with NH White patients (thus accounting for the non-White patients’ shorter length of stay and the lack of racial differences in time to provider and hospital admission rates).
Table 7
Description of a Rapid Triage Fast Track model.
One study assessed the impact of a rapid triage FT model, which is an algorithm-informed care pathway based on ESI. The authors studied this model’s effect on outcomes in Black NH and White NH patients presenting to the ED of a tertiary care hospital in Minnesota.63 Using EHR data, the study examined 9704 patients with 12,330 unique encounters (5151 Black NH and 7179 White NH, exact-matched on potential confounders, including presence of abnormal vital signs) during a 1-year period after implementation of the FT model. Race and ethnicity were self-reported. (Table C-1 in Appendix C).
Compared with White NH patients, Black NH patients were significantly more likely to be assigned to FT, a lower acuity area (22.6 percent vs. 18.5 percent; odds ratio [OR] 1.28, 95 percent CI 1.12 to 1.46, p<0.001), and significantly less likely to be categorized as a high-acuity patient (59.8 percent vs. 67 percent; OR 0.73, 95 percent CI 0.66 to 0.81, p<0.001). Among patients designated as high acuity, Black NH patients were also significantly more likely than White NH patients to be assigned to the FT area (3.4 percent vs. 2.5 percent; OR 1.40, 95 percent CI 1.05 to 1.87, p=0.024). The difference between Black NH and White NH low-acuity patients was not significant (p=0.934). In a subgroup analysis, Black NH patients with abdominal pain, shortness of breath, chest pain, or headache had an increased likelihood of being assigned to the FT area than White NH patients. The difference between racial and ethnic groups was significant for the chief issue of headache (OR: 2.10; 95 percent CI 1.01 to 4.39, p=0.048).
Black NH patients also had a significantly shorter wait time between ED arrival and being placed in a room than White NH patients (MD -3.47 minutes, 95 percent CI -6.56 to -0.37, p=0.028). Subgroup analyses also demonstrated significantly shorter wait times for Black NH patients than White NH patients with a chief issue of abdominal pain (mean difference [MD] -9.52 minutes, 95 percent CI -20.02 to -0.03, p=0.028) and chest pain (MD -18.82 minutes, 95 percent CI -28.93 to -8.72, p<0.001). Authors suggest that the shorter average wait time for Black NH patients may be associated with Black NH patients being more likely to be triaged to the FT area.
The rapid triage FT model, an algorithm-informed care pathway, involves ESI score assignment by a triage provider (e.g., nurse) that assesses a patient’s acuity level. Study authors suggest that triage provider assessment might introduce implicit bias and potentially affect triage decisions.63 Using the FT model, Black NH patients presenting to the ED were less likely than White NH patients to be categorized as needing immediate or urgent care and were more likely to be triaged to the FT area, which was designed to evaluate and manage lower-acuity patients. Authors concluded the FT model led to Black NH patients receiving lower-acuity scores compared with White NH patients at the same level of need, which may potentially exacerbate racial and ethnic disparities in access to care.
3.2.3.2. High-Risk Chronic Disease Care Management
Table 8
Description of a high-risk care management prediction algorithm.
One study examined racial differences in healthcare resource allocation produced by what the authors termed a “widely used” commercial risk prediction algorithm and examined strategies to reduce those differences.5 This study’s data came from a health system in which patients with scores on the algorithm above the 97th percentile are automatically identified for enrollment into the system’s care management program. The study sample consisted of all primary care patients enrolled in risk-based contracts at a large academic hospital from 2013 to 2015 and self-identifying as either Black or as White without another race (n=49,618). For each patient, algorithmic risk scores generated annually by the health system were obtained, as well as actual costs per year. Also, the total number of chronic conditions was calculated for each patient as a measure of overall illness burden to examine the extent to which the algorithm had allocated additional resources to the patients with the greatest need.
At every level of algorithm-predicted risk, Black and White patients had similar actual costs in the following year. However, at a given level of health (measured, as described above, by number of chronic conditions), Black patients generated lower costs than White patients – on average, $1801 less per year. Thus, although the algorithm predicted costs equally well for Black and White patients, costs cannot be assumed to be a valid proxy for healthcare needs because the association between costs and health differs across racial and ethnic groups. At the cutoff score for automatic identification for enrollment into the care management program (97th percentile), Black patients had 26.3 percent more chronic conditions than White patients (p<0.001). Thus, use of the algorithm to determine program eligibility hypothetically leads to acceptance of White patients who have a lower level of actual need than Black patients (i.e., greater access to healthcare for Whites than Blacks). Further discussion of this study’s subsequent mitigation strategy is described in KQ 2.
3.2.3.3. Kidney Transplant Allocation
Table 9
Description of the Kidney Allocation System.
One study examined how the 2014 revised Kidney Allocation System (KAS) affected racial and ethnic differences in the waitlisting rate (i.e., placement on the national deceased donor waiting list).61 More information on the revised KAS is in Table C-1 in Appendix C. Authors selected data from the U.S. Renal Data System of 1,253,100 new (n=1,120,655 pre-KAS and n=132,445 post-KAS) and 1,556,954 existing patients on dialysis between 2005 and 2015.
Of note, the analyses reported in this study do not examine how implementing KAS affected transplantation rates (which is the clinical outcome that the KAS directly determines), but rather how the policy’s existence affected the waitlisting rate, which is an “upstream” clinical outcome for a patient before the KAS comes into play. The rationale for examining this outcome is that awareness of the policy change, and thus the change in the anticipated likelihood of individual patients receiving transplants once on the waitlist, could have affected clinicians’ decisions about whether to initiate the requisite screening process.
After implementing KAS, small to moderate declines occurred in the waitlisting rate for Black (4 percent), Asian (8 percent), Hispanic (10 percent), and White (11 percent) patients. The interaction of race and ethnicity with KAS implementation was significant (p<0.0001). Authors further examined the difference in waitlisting rates among incident and prevalent end-stage renal disease (ESRD) patients. Compared with White incident ESRD patients, Black incident ESRD patients had a 19 percent lower waitlisting rate before implementation of KAS (adjusted hazard ratio [aHR]: 0.81; 95 percent CI 0.80 to 0.82). Post-KAS, the difference between White and Black patients declined significantly to 12 percent (aHR: 0.88; 95 percent CI 0.85 to 0.90; p<0.001), partially due to more Black patients with incident ESRD placed on the waitlist. However, a difference in waitlisting rates remained. Before and after KAS implementation, Asian and Hispanic incident ESRD patients had higher waitlisting rates than White incident ESRD patients. The differences from pre- to post-KAS were not significant (Asian vs. White p=0.27; Hispanic vs. White p=0.62). Monthly waitlisting rates for prevalent dialysis patients decreased from pre- to post-implementation for all racial and ethnic groups, with a statistically significant decrease for White (p=0.017), Black (p=0.011), and Hispanic (p=0.03) patients.
Another analysis in this study examined active and inactive waitlisting rates before and after KAS implementation.61 Actively listed patients can be called to receive a kidney transplant at any time, while patients listed as inactive are not eligible to be called for a transplant due to reasons such as health concerns. Active waitlisting rates were similar before and after KAS implementation (p=0.601), while inactive waitlisting rates declined significantly (p <0.001). The proportion of new actively waitlisted candidates (i.e., eligible to be called for transplantation) increased from pre- to post-KAS for Black (71.3 percent vs. 76.3 percent), Hispanic (72.2 percent vs. 78 percent), and Asian (72.7 percent vs. 73.5 percent) patients, while declining slightly for White patients (72.3 percent vs. 71.4 percent). Results also demonstrated a greater decline in inactive waitlisting counts (i.e., patients on the list but not eligible to be called for transplantation) among Black and Hispanic patients following KAS implementation (p<0.0001). For more information, see Table D-1 in Appendix D.
Study findings indicate that, post-KAS implementation, the overall waitlisting rate declined for all racial and ethnic groups, and the difference in rates between Black and White incident ESRD patients declined significantly but was not eliminated. Results suggest that the overall decline in waitlisting rates was due to a decline in inactive waitlisting, while rates of active waitlisting (i.e., patients actually eligible for transplant being placed on the waitlist, remained relatively stable). Similarly, the reduction in the difference between Black and White incident ESRD patients was due to both a decrease in inactive waitlisting and an increase in active waitlisting among BIPOC (Black, Hispanic, and Asian) patients.61 That is, post-KAS, fewer BIPOC patients were listed as ineligible for transplantation and a greater proportion listed as eligible. Authors also speculate that the decline in waitlisting rates might reflect a reduction in transplant referrals, which could negatively affect patients in need of resources and treatment.
3.2.3.4. Lung Transplant Allocation
Table 10
Description of the Lung Allocation Score.
One study analyzed data from all White and Black non-Hispanic adults who were listed for lung transplantation during two time periods: pre-LAS (2000–2005; n=8765) and LAS (2005–2010; n=8806).62 In the pre-LAS period, Black patients were far more likely than White patients to die or become too sick for transplantation within 3 years of listing (43.8 percent vs. 30.8 percent, adjusted OR 1.84; p <0.001); the difference became negligible in the LAS period (14.0 percent vs. 13.3 percent, adjusted OR 0.93; p = 0.74). Black patients were 18 percent more likely than White patients to die while on the waitlist in the pre-LAS period (adjusted hazard ratio [HR] 1.18; 95 percent CI 0.99 to 1.40; p=0.06); in the LAS period, Black patients were 17 percent less likely than White patients to die (adjusted HR 0.83; 95 percent CI 0.62 to 1.10; p=0.18).
3.2.3.5. Lung Cancer Screening
Table 11
Description of lung cancer screening prediction models.
Two studies59,109 examined racial and ethnic differences in lung cancer screening recommendations between the U.S. Preventive Services Task Force (USPSTF) guidelines (2013 version) and a risk prediction algorithm, the PLCOm2012 Model. (Both studies were conducted before the 2020 revision of the guidelines, limiting the applicability of results to current clinical practice.)
In one study, patients (n=883) enrolled in a lung cancer cohort between 2010 and 2019 were selected for analysis.109 Findings demonstrated that the PLCOm2012 prediction model (threshold: >1.7 percent/6-year risk) reduced the difference between Black and White patients in the percentages ineligible for screening based on the USPSTF-2013 criteria. The percentage of patients who were ineligible by USPSTF-2013 criteria was 35.3 percent among White patients and 48.3 percent among Black patients, expanding the eligibility criteria to include patients classified as being at risk by PLCOm2012 reduced the percentages to 26.0 percent and 26.3 percent, respectively.
The second study used a simulated dataset (n=100,000) representing the 1950 U.S. birth cohort and containing smoking history data generated by the CISNET (Cancer Intervention and Surveillance Modeling Network) Smoking History Generator and risk factor data generated by the Lung Cancer Risk Factor Generator.59 For the PLCOm2012, a risk of >1.51 percent was used as the threshold for eligibility for screening.
Among individuals aged 50–54, 4.8 percent of White individuals and 15.6 percent of Black individuals were eligible for screening by PLCOm2012 but ineligible by USPSTF criteria. Among individuals aged 55–70, the percentages were 3.3 percent and 7 percent, respectively; among those aged 71–80, the percentages were 10.8 percent and 14.2 percent, respectively. In the youngest and oldest of the three age groups, the difference in percentages was significant, at p <0.001; the p-value for the middle group was not reported. Results at varying risk thresholds are presented graphically; the authors described the proportion as “consistently higher in Black individuals compared with White individuals independently of risk threshold.”
A sensitivity analysis was performed on a similar dataset representing the 1960 U.S. birth cohort. Differences persisted but were generally smaller than those in the 1950 cohort. Across all age groups in the 1960 cohort, 2.3 percent of White individuals and 5.8 percent of Black individuals were eligible for screening by PLCOm2012 but ineligible by USPSTF (p<0.001).
A third study examined racial and ethnic differences in the percentages of individuals eligible for screening under four different sets of criteria: (a) the 2013 USPSTF eligibility criteria; (b) the 2021 expanded USPSTF criteria; (c) lung cancer risk as calculated by the PLCOm2012(Race3L) model, using a value of 1.5 percent 6-year risk as the threshold for eligibility; and (d) PLCOm2012(Race3L) risk using a 1 percent threshold value.67 Data came from the 2019 Centers for Disease Control Behavioral Risk Factor Surveillance System; the analysis sample included respondents who were 50+ years old and were current or former smokers. The sample included 41,544 individuals (88.5 percent non-Hispanic White, 5 percent non-Hispanic Black, 2 percent Hispanic, 4.5 percent other). Overall, the 2013 USPSTF criteria identified the lowest percentage of individuals as eligible for screening (21 percent), and the PLCOm2012(Race 3L) model using the 1 percent threshold identified the highest (45 percent), with the 2021 USPSTF criteria and the PLCOm2012(Race 3L) model using the 1.5 percent threshold identifying similar, intermediate percentages (34.7 percent and 35.3 percent, respectively). All four sets of criteria, however, resulted in differences across racial and ethnic groups in percentages identified as eligible, with lower percentages among Black and Hispanic individuals than among White individuals and those of other races. Using the 2013 USPSTF criteria, the percentages identified as eligible for screening among White, Black, Hispanic, and other individuals were 21.9, 16.0, 9.8, and 22.1, respectively; using the 2021 USPSTF criteria, the percentages were 35.8, 28.5, 18.0, and 39.3, respectively; using the PLCOm2012(Race 3L) model with a 1.5 percent threshold, the percentages were 36.2, 31.1, 15.0, and 43.4, respectively; and using the PLCOm2012(Race 3L) model with a 1 percent threshold, the percentages were 46.3, 39.3, 20.3, and 51.4, respectively.
3.2.3.6. Opioid Misuse Risk
Table 12
Description of a natural-language processing classifier.
One study108 examined a natural-language-processing classifier. The study analyzed an external validation dataset of adult inpatient encounters (n=53,974). Patients’ actual opioid misuse was assessed by screening questions administered at admission.
The key outcome was the false-negative rate (FNR): the percentage of actual opioid misusers whom the classifier missed. The FNR was considerably higher among Black patients (32 percent) and “Other” racial and ethnic groups (33 percent) than among White patients (17 percent) and Hispanic/LatinX patients (17 percent). This suggests a race disparity in resource allocation: while 83 percent of White and Hispanic/LatinX patients would receive needed resources based on the classifier, only 67 percent of Blacks/Others would.
3.2.3.7. Prostate Cancer Risk
Table 13
Description of prostate cancer screening algorithms.
Two studies applied algorithms retrospectively to cohorts of men who had received biopsies based on abnormal digital rectal exams and/or elevated levels of prostate-specific antigen (PSA).56,60 The studies calculated, if the biopsy decisions had been based solely on the algorithms, how many negative biopsies would have been avoided, how many total biopsies would have been avoided, and how many cancers would have been missed. A “net benefit” calculation illustrates the key tradeoff, as it provides the number of negative biopsies avoided for each missed high-grade cancer.
In one study, a newly developed algorithm, the Kaiser Permanente Prostate Cancer Risk Calculator, was externally validated.56 Results were presented by racial and ethnic category for two versions of the model (versions A and B).
The net benefit for members of each racial and ethnic category differed substantially depending on the model version and threshold value used. For example, using version A and a risk threshold of ≥10 percent, 9 percent of negative biopsies would have been avoided among White patients while missing 1 percent of high-grade cancers (i.e., 9:1 ratio); among Black patients, 25 percent of negative biopsies would have been avoided, but 6 percent of high-grade cancers would have been missed, yielding a ratio of only about 4:1. By contrast, using version B and a ≥10 percent cutoff, the percentages of unnecessary biopsies avoided and high-grade cancers missed would be 39 percent and 4 percent, respectively, for White patients (i.e., about a 10:1 ratio), and 61 percent and 5 percent, respectively, for Black patients (i.e., about a 12:1 ratio).
For Hispanic patients, the effect of using the algorithm would have been relatively low; using version B with a ≥10 percent cutoff, 24 percent of negative biopsies would have been avoided and 1 percent of high-grade cancers would have been missed. For Asian patients, the net benefit of using the algorithm would have been, in general, less positive than for the other groups. Using version B with a ≥10 percent cutoff, 51 percent of negative biopsies would have been avoided, but 9 percent of high-grade cancers would have been missed (i.e., 5.7:1 ratio).
The second study compared the Prostate Cancer Prevention Trial (PCPT) 2.0 and the Prostate Biopsy Collaborative Group (PBCG) algorithms.60 Decision curve analysis was used to calculate the net benefit that would have accrued to the men in the sample if each algorithm had been used to determine whether they should have a biopsy, as well as the net benefit of conducting biopsies on all men. While the article does not define “net benefit,” the term has a formal definition in decision curve analysis; it is a function of the true-positive rate, the false-positive rate, and the threshold risk value. Each strategy’s net benefit was calculated, using a range of threshold risk values from 5 percent to 40 percent (described by the authors as the range that “patients and providers usually have”), separately for White men, Black men, and others (which were 75 percent Hispanic and 25 percent Asian). The authors depicted the results graphically in their Figure 3 (see original article). In general, the net benefit of all three strategies declined, or at best remained constant, as the threshold probability used increased. For both Black and White men, neither algorithm had a net benefit superior to that of the strategy of conducting a biopsy of all men at any risk threshold below 30 percent. For Black men, there was little difference in net benefit for any of the three strategies, except at the 40 percent risk threshold, at which the net benefit of performing a biopsy on all men became slightly negative while that of the two algorithms remained positive; PCPT’s net benefit was slightly higher than that of PBCG at values above 30 percent. For White men, by contrast, PCPT’s net benefit was lower than that of PBCG at all threshold probabilities, while the net benefit of performing a biopsy on all men was similar to that of PBCG except at threshold values over 30 percent, where it dropped below the two algorithms. The net benefit of all three strategies was higher for Black men than for White men at all threshold values.
For the men in the sample belonging to other racial and ethnic groups, the net benefit of two of the strategies (PBCG and biopsying all men) was lower than that for Black or White men at all threshold values. The net benefit of performing biopsy on all men was lower than that of PBCG for threshold values of 20 percent or above but was negative for both. At threshold values above 10 percent, PCPT’s net benefit was higher than that of the other two (remaining slightly positive) and was comparable to PCPT’s net benefit among White men.
3.2.3.8. Severity of Illness Measurements for Crisis Standards of Care
Table 14
Description of severity of illness measurements.
Three studies54,57,107 retrospectively evaluated racial and ethnic differences in the performance of four models used to predict risk of mortality:APACHE IVa, LAPS2, OASIS, and SOFA. None of the models use race or ethnicity as an input.
All three studies selected patients from an EHR107 or intensive care unit (ICU) database such as the eICU Collaborative Research database (eICU-CRD)54,57 or the Medical Information Mart for Intensive Care-III (MIMIC-III) database57. Two studies limited analyses to Black and White patients,54,107 and the third study also included Hispanic and Asian patients.57 For more information, see Table C-1 in Appendix C.
One study compared the performance of SOFA and LAPS2 in Black and White patients (n=113,158) admitted to 27 hospital EDs between 2013 and 2018 with acute respiratory failure or sepsis (Table C-1 in Appendix C).107 Most patients were White (75.6 percent), and White patients were older than Black patients (mean age: 67.1 vs. 61.7, p<0.001). Black patients had higher overall mean SOFA scores (3.1 [standard deviation (SD) 2.1] vs. 2.9 [SD 1.8], p<0.001) than White patients, indicating a lower predicted likelihood of survival, but lower mean LAPS2 scores (102.2 [SD 38.4] vs. 103.1 [SD 36.7], p<0.001), indicating a higher predicted likelihood of survival. However, authors found that at a given SOFA or LAPS2 score, Black patients had lower in-hospital mortality than White patients in almost every category, suggesting that both prediction models overestimated in-hospital mortality for Black patients and underestimated this outcome for White patients (Table D-1 in Appendix D). Use of these models to prioritize resource allocation in ICUs (with priority given to patients most likely to survive) would thus tend to lead to inappropriate deprioritization of Black patients.
This study also examined the subset of patients in the highest-priority category (i.e., SOFA <6), indicating a higher predicted likelihood of survival, and again found that Black patients had lower in-hospital mortality than White patients (5.3 percent vs. 6.9 percent).107 To illustrate the extent of inappropriate deprioritization associated with this discrepancy, the authors performed a simulation analysis in which Black patients with SOFA scores ≥6 were sequentially reclassified into the highest-priority category until rates of in-hospital mortality for Black and White patients in that category were similar (6.7 percent vs. 6.9 percent). The Black patients thus reclassified were those with SOFA scores of 6 to 8 (n=2611), representing 9.4 percent of all Black patients and 81.6 percent of Black patients with SOFA >5 (Table D-1 in Appendix D). Overall, authors found the use of illness severity models in crisis standards of care, in particular SOFA, “may divert critical care resources away from Black patients and lead to racial disparities in resource allocation.”107
One study examined the APACHE IVa, OASIS, and SOFA illness severity models in patients admitted to the ICU.57 Participants were selected from two ICU databases: the eICU-CRD (n=122,919) between 2014 and 2015, which contains APACHE IVa scores, or the MIMIC-III (n=43,823) between 2001 and 2012, which includes OASIS scores. Authors calculated SOFA scores for participants in both databases. Race and ethnicity data captured in each database were self-reported (Table C-1 in Appendix C). Both APACHE IVa and OASIS overestimated mortality for all racial and ethnic groups, and overestimates were worse for Black and Hispanic patients. Standardized mortality ratios of observed to predicted death rates for both prediction models were lower (i.e., overestimated mortality to a greater extent) for Black (0.67 for both models) and Hispanic (0.73 and 0.64) patients than for White (0.76 and 0.81) and Asian (0.77 and 0.95) patients, respectively. Among patients with SOFA scores 0 to 7, the ratio of the observed ethnicity-specific mortality rate to the mortality rate in the overall population was lower for Black (0.86 and 0.74) and Hispanic (0.96 and 0.62) patients than for White (1.02 and 1.04) and Asian (1.12 and 1.06) patients when calculated for both databases, eICU-CRD and MIMIC-III, respectively. Thus, to be placed in the lowest category of predicted risk (and therefore be assigned the highest priority), Black and Hispanic patients had to have lower true risk than White and Asian patients. See Table D-1 in Appendix D.
A third study examined whether use of the SOFA score is associated with inappropriate deprioritization of Black patients in currently adopted crisis standards of care.54 For use in allocating resources, SOFA scores are collapsed into tiers; depending on the severity of the shortage, resources may be allocated only to patients in the highest-priority tier (i.e., those with the lowest scores and lowest risk of mortality), the two highest-priority tiers, etc. Authors evaluated three widely used tier systems, termed A (4 tiers, with scores <6 forming the highest-priority tier and scores ≥12 forming the lowest), B (3 tiers, scores <8 highest priority, ≥12 lowest), and C (4 tiers, scores <9 highest priority, ≥15 lowest). SOFA scores were retrospectively calculated for 111,885 patient encounters involving 95,549 unique patients in the eICU-CRD occurring between 2014 and 2015. The sample included 16,688 encounters with Black patients (14.9 percent) and 95,197 encounters with White patients (85.1 percent) (Table C-1 in Appendix C).
One analysis modeled actual in-hospital mortality using the continuous version of the SOFA score, race, and the interaction of race by SOFA score.54 The interaction was significant (OR for Black vs. White, 0.98; 95 percent CI, 0.97 to 0.99; p<0.001). This indicated a small but statistically significant tendency for the SOFA score to overestimate the true risk of death among Black patients compared with White patients, thus hypothetically lowering their eligibility for resources compared with White patients. See Table D-1 in Appendix D.
Another analysis in this study examined the tier systems.54 For each system, this analysis focused on the subset of patients in the highest-priority tier (i.e., those who would be prioritized for resources under conditions of severe shortage) and compared the adjusted odds of in-hospital mortality among Black versus White patients (Table D-1 in Appendix D). Black patients had significantly lower odds of in-hospital mortality than White patients in the highest tier of system A (OR, 0.65; 95 percent CI, 0.58 to 0.74; p <0.001), system B (OR, 0.70; 95 percent CI, 0.64 to 0.78; p <0.001), and system C (OR, 0.73; 95 percent CI, 0.67 to 0.80; p <0.001), indicating that, under each system, Black patients had to have a lower true risk of death to qualify for resources than White patients. The percentage of Black patients who would have been inappropriately deprioritized (i.e., assigned to a lower-priority tier even though their true risk of death was lower than some patients in the highest-priority tier) was 15.6 percent for system A, 9.0 percent for system B, and 6.5 percent for system C. Across all systems and all levels of shortage, increasing the SOFA threshold by 2 points for Black patients would be necessary to equalize the adjusted odds of death for Black and White individuals who qualify for the high-priority tier.
A fourth study examined differences across racial groups in estimated rates of excess deaths that would have been caused by using a crisis standards of care algorithm to ration mechanical ventilators.65 The sample consisted of patients who were admitted to the ICUs of 6 hospitals in a Boston-area hospital system in April and May of 2020 and received mechanical ventilation (n=244). The distribution of self-reported race in this sample was 16.8 percent Black, 49.1 percent White, 2.8 percent Asian, 10.6 percent of any other reported race, and 20.4 percent of unknown race. Priority scores were preemptively calculated for these patients in anticipation of resource shortages due to COVID-19 (which did not materialize), using state-issued guidelines. Scores were based on estimated likelihood of acute and long-term survival. Acute survival was estimated using the SOFA score grouped into four categories (1 point for best prognosis, 4 points for worst). Long-term survival was estimated using a 3-level score (0 points for best prognosis, 2 points for intermediate, 4 points for worst) based either on comorbidity data from the electronic medical record (through April 27) or on a clinical assessment by the attending physician (after April 27). The total score was the sum of the acute and long-term scores and was grouped into three tiers: highest priority (scores of 1 or 2), intermediate (3 to 5), and lowest (6 to 8). If this system had been used to allocate ventilators to the patients in the sample, 140 would not have received ventilation if ventilators had been allocated only to patients in the highest-priority tier, and 30 would not have received ventilation if ventilators had been allocated to patients in the highest and intermediate tiers. The analysis assumed that all patients who lived, but would not have received ventilators under these scenarios, would have died (i.e., excess deaths). At the cutoff of ≤2, the estimated number of excess deaths among Black patients was 18 (i.e., there were 18 patients who lived, but would not have received ventilation because they had scores >2). This represented 43.9 percent of all Black patients in the sample compared with a rate of 28.6 percent among the other 203 patients (p = 0.05). At a cutoff of ≤3, the estimated excess mortality among Black patients was 26.8 percent and 14.3 percent among all other patients (p = 0.05). There were no statistically significant differences in excess mortality between Black patients and all other patients at any other cutoff (p’s ≥0.08) or between Black and White patients at any cutoff (p’s ≥0.22).
In summary, findings from these four studies54,57,65,107 indicate that illness severity models consistently overestimated mortality in Black patients compared with White patients. That is, at any given level of algorithm-predicted risk, Black patients had lower actual mortality than White patients. Using prediction models that overestimate mortality in Black patients can lead to inappropriate deprioritization and divert resources away from Black patients.
3.2.3.9. Stroke Risk
Table 15
Description of the CHA2DS2-VASc.
One study examined potential racial and ethnic disparities in health outcomes that could occur as a result of using the CHA2DS2-VASc, which predicts stroke risk in patients with atrial fibrillation and is used to guide recommendations for anticoagulation treatment.66
The study data came from the Stanford Medicine Research Data Repository, which is composed of records from Stanford Health Care and the Lucile Packard Children’s Hospital. Race and ethnicity were self-reported; the racial and ethnic groups included were White, Black, Hispanic, and Asian. Potential disparities in health outcomes were quantified by identifying individuals who would have been denied treatment based on CHA2DS2-VASc and ascertaining the frequency of negative events (stroke) among these individuals.
The sample consisted of 233,129 patients (176,278 White, 33,927 Asian, 13,578 Hispanic, 7323 Black, and 2023 other). The 2014 American College of Cardiology/American Heart Association atrial fibrillation treatment guideline, an algorithm-informed decision tool, uses a threshold of a CHA2DS2-VASc score > 1 to recommend anticoagulant therapy. The negative event frequency was 3.30 for Hispanic patients (i.e., 3.3 percent of Hispanic patients who would not have received a recommendation for anticoagulant therapy had a stroke), 2.26 for Asian patients, 2.21 for White patients, and 2.19 for Black patients. The 2020 guideline uses a higher threshold (CHA2DS2-VASc score >2 for males and > 3 for females) for recommending anticoagulant therapy. The negative event frequency when using the 2020 guideline was 2.21 for Black patients (i.e., 2.21 percent of Black patients who would not have received a recommendation had a stroke), 2.15 for Asian patients, 2.14 for White patients, and 1.78 for Hispanic patients. Authors suggest that when using the 2020 guideline, the disparity in negative event frequency (stroke) in Hispanic patients was reduced.
This study also examined potential racial and ethnic disparities in health outcomes that could occur as a result of using the Model for End-Stage Liver Disease (MELD) calculator and simplified Pulmonary Embolism Severity Index (sPESI). However, authors reported that the algorithms performed poorly for different racial and ethnic groups. Due to limitations in subgroup performance, there was insufficient information about the potential impact of the MELD calculator and sPESI on racial and ethnic health outcomes; therefore, we present study findings only for the CHA2DS2-VASc scor
3.3. Key Question 2. What is the effect of interventions, models of interventions, or other approaches to mitigate racial and ethnic bias in the development, validation, dissemination, and implementation of healthcare algorithms?
- Datasets: What is the effect of interventions, models of interventions, or approaches to mitigate racial and ethnic bias in datasets used for development and validation of algorithms?
- Algorithms: What is the effect of interventions, models of interventions, or approaches to mitigate racial and ethnic bias produced by algorithms or their dissemination and implementation?
3.3.1. Description of Included Evidence
Our searches identified 44 studies (Table 16) published between 2011 and 2023 that met our inclusion criteria and evaluated strategies to mitigate racial and ethnic disparities associated with healthcare algorithms.5,21,23,68–108 The evidence base included 1 randomized controlled trial,93 17 studies that used cohort, pre-post, or cross-sectional designs,5,21,23,71,72,74–77,83,86–88,92,95,107,108 and 26 studies involving comparison models or simulated effects.68–70,73,78–82,84,85,89–91,94,96–106 Detailed information about the included studies is provided in Table C-2 in Appendix C and Table D-2 in Appendix D. 21,23,69,70,72,73,75–77,79,80,82,83,86,99–105
Twenty-one studies measuring kidney function21,23,69,70,72,73,75–77,79,80,82,83,86,99–105 and seven studies predicting cardiovascular risk81,84,87,88,90,95,96 composed the majority of the research on mitigation strategies, but numerous other clinical issues were addressed as well. Four studies addressed organ donation,89,94,97,98 three studies examined algorithms for appropriate warfarin dosing,92,93,106 and the remaining studies addressed lung function,68,71 stroke risk,91 intensive care needs,107 lung cancer screening,74 postpartum depression,78 opioid misuse,108 and healthcare costs and utilization.5,85
Our searches identified numerous strategies used to mitigate bias in healthcare algorithms. Broadly, these strategies fall into six categories: removing an input variable (usually race and ethnicity) without changing an algorithm’s other features; replacing an input variable with one or more different variables; adding one or more input variables without removing any; changing the racial and ethnic composition of the patient population used to train or validate a model; stratifying algorithms by race and ethnicity; or modifying the statistical or analytic techniques used by an algorithm. Three studies73,78,88 used more than one strategy. The most common approach, used in 24 of 44 studies, was to remove race. Not surprisingly, this strategy was used predominantly in studies of eGFR, but removal of race-based variables was also evaluated in studies of lung function,68,71 kidney donor suitability,97,98 and postpartum depression.78 Five studies replaced an input variable with something different; three of these replaced race in eGFR with biological indicators, such as cystatin-C or metabolic markers,23,73,86 while a study of the Kidney Donor Risk Index (KDRI) replaced race with a genetic marker.89 Lastly, in Obermeyer’s landmark study of a healthcare needs algorithm that did not include race, the utilization variable that was identified as causing disparities was replaced with three other measures of patient needs that were not associated with outcome disparities.5
Eight studies added an input variable to improve algorithm performance: three of these added race to address disparities associated with initially race-free algorithms for risk of cardiovascular disease87,95 or stroke;91 four studies added genetic or other biological variables to cardiovascular risk prediction algorithms81,90 or warfarin dosing algorithms;93,106 one study added a measure of life-years gained to a screening algorithm for lung cancer;74 and one study incorporated social determinants of health (SDOH) measures into an algorithm that predicted healthcare use, costs, and mortality.85
Four studies recalibrated models using datasets derived from a different mix of patients than those used for initial model development. These studies focused on cardiovascular risk for Black patients,84,88 postpartum depression for women who receive Medicaid,78 and Black liver donors with hepatitis C.94 In two studies, the authors sought to address concerns about algorithms for warfarin dosing92 and opioid misuse108 by developing separate algorithms or thresholds for Black and White patients. Finally, in three studies that focused on postpartum depression78 and cardiovascular risk,88,96 the statistical methods used for model calibration were modified with innovative techniques designed to mitigate potential algorithmic bias.
As with KQ 1, studies addressing KQ 2 usually included patients from EHRs, clinical trials, or national databases such as the National Health and Nutrition Examination Survey. In 14 studies, race and ethnicity were self-reported,5,68–70,81,85,87,99,102–106,108 while 27 studies did not describe how race and ethnicity were collected. Two studies82,101 included a combination of self-reported and administratively designated classifications for race and ethnicity, and one study91 employed an algorithm developed by the Research Triangle Institute that assigns race and ethnicity based on first and last name. In 29 studies, analyses were restricted to only 2 race and ethnicity groups (African American/Black and White/Non-Black), while 5 other studies reported data for these 2 groups in addition to patients categorized as Asian or Hispanic.71,74,79,85,105 Because KQ 2 focused on mitigation strategies, we also identified 10 studies that reported outcomes only for Black patients.69,70,75,77,80,82,83,89,90,104
Overall, ROB was rated as Low for 8 studies, Moderate for 31 studies, and High for 5 studies. The strengths and limitations affecting ROB for the KQ 2 studies were similar to those for the KQ 1 studies, as described above. The equity-based signaling questions changed domain ROB in six studies, but only changed overall ROB in one study.91 The most common domain to receive a change in ROB rating was for bias in selection of study participants due to inconsistent reporting of racial and ethnic groups (i.e., self-reported as ideal) and with inconsistent definitions and categories. Complete ROB ratings are in Appendix Table D-3.
Table 16
Mitigation strategies.
3.3.2. Key Points
- We included 44 studies addressing a broad range of clinical assessment. The most frequently examined algorithms evaluated kidney function and cardiovascular risk.
- Six types of mitigation strategies were examined, with some studies testing multiple strategies. The most common approach was removal of race, which occurred in 24 studies. Five studies replaced race or another input variable with a different measure, while nine studies added an input variable to an algorithm. In four studies, an algorithm was recalibrated with a more representative patient population. Two studies developed stratified algorithms that assessed Black and White patients separately, and three studies evaluated the effect of different statistical techniques within algorithms.
- The evidence base featured considerable heterogeneity across patient populations, clinical conditions, healthcare settings, and primary outcomes.
- Removing race from eGFR may increase the likelihood of diagnosis of chronic kidney disease and severe kidney disease in Black patients. This could result in broader and earlier eligibility for kidney transplant. Conversely, removing race from eGFR might reduce access to other types of treatment, affect medication dosing for a broad range of conditions, and reduce enrollment of Black patients in clinical trials.
- Most published studies found that mitigation strategies may reduce racial and ethnic disparities and could improve outcomes for BIPOC patients. However, strategies that improve one outcome (e.g., eligibility for kidney transplant) may have undesired effects on other outcomes (e.g., medication dosing or eligibility for enrollment in clinical trials).
- A mitigation strategy’s effectiveness may depend critically on a unique combination of algorithm, clinical condition, population, setting, and outcomes. It is unclear from the current evidence base if certain types of strategies are generally more effective than others, or what the implications are for both existing and future algorithms.
3.3.3. Summary of Findings
The sections below discuss six categories of mitigation strategies:
- Removing Input Variables
- Replacing Input or Outcome Variables
- Adding Input Variables
- Changing the Patient Mix Used for Development and Validation
- Developing Separate Algorithms by Race
- Refining Statistical and Analytic Techniques
3.3.3.1. Removing Input Variables
Our review identified 24 studies that examined the effect of removing race from an algorithm. Nineteen of these studies focused on kidney function as estimated by eGFR, two evaluated kidney donor suitability in the KDRI and Kidney Donor Profile Index (KDPI), two examined lung function, and one study addressed postpartum depression.
The 19 studies of eGFR were heterogeneous in their overall objectives and in the type and number of outcomes assessed. Ten studies examined the effect of removing race from eGFR on diagnosing kidney disease or classifying disease severity.21,69,70,73,77,79,80,82,99,105 Seven studies evaluated prediction of mortality,102 kidney failure,70,102 end-stage kidney disease,103 progression of kidney disease in patients with human immunodeficiency virus,101 and acute kidney injury in patients with cirrhosis75 or following percutaneous coronary intervention.100 Finally, several studies explored possible downstream effects of removing race, including changes to medication dosing,76,105 appropriateness of drug therapy or other treatments,69,105 potential enrollment in clinical trials,104 and eligibility for kidney21,72,80,83,105 or joint liver-kidney transplant.77
The effects of removing race from eGFR were consistent across most studies, although some variation in outcomes emerged. One analysis70 of a national healthcare database found that the proportion of Black patients qualifying for a diagnosis of chronic kidney disease more than doubled when the race coefficient was removed. In a separate analysis of Veterans Administration patients in that same study, diagnosis in Black patients rose by 74 percent. A study by Shi et al.79 demonstrated that after removing the race coefficient, between 16 percent and 38 percent of Black patients in every severity class (stages 1 through 4) were reclassified to a higher stage, and no Black patients were reclassified to a lower stage. Conversely, when patients of all races were combined, between 1 percent and 30 percent of patients moved to a lower stage while just 1 percent to 5 percent moved to a higher stage.
A study77 examining data from a national transplant registry found that removing the race coefficient from eGFR led to a 26 percent increase in eligibility of Black patients for kidney transplant waitlists. Hoenig et al.72 reported a more modest but still meaningful increase in transplant eligibility; the authors found that 15 percent of patients who were added to a transplant list after the race coefficient was removed would not have been eligible when the race coefficient was in use. Diao et al.105 found that Black patients would have expanded access to nephrology referral and preemptive arteriovenous fistula, while Medicare coverage of kidney disease education and medical nutrition therapy would increase by 45 and 48 percent, respectively.
Two studies examined the effect of removing race in eGFR in patients with cirrhosis. As background, patients with cirrhosis are assessed using the Model for End-Stage Liver Disease score, which incorporates the race-based eGFR equation and can drive clinical decision making, including liver transplant eligibility. Mahmud et al.75 found that inclusion of race in eGFR in a Veterans Administration dataset did not improve prediction of acute kidney injury events. Panchal et al.77 used data from a national transplant registry and found that removing the race coefficient from eGFR could lead to a 26 percent increase in eligibility of Black patients for simultaneous liver-kidney transplantation.
Not all consequences are necessarily positive. A multivariate model21 found that removing race from eGFR would result in no Black patients referred to transplant waitlists; the reason for that is unclear. Casal et al.69 reported that, although 26 percent of Black patients who were undergoing cancer treatment were reclassified as having more severe kidney disease, 5 percent were newly deemed ineligible to receive cisplatin because their revised renal function estimate exceeded standard medication safety thresholds. Diao et al.,105 using 18 years of National Health and Nutrition Examination Survey (NHANES) data, determined that 38 percent of Black patients would see a reduction in their dose of common medications (e.g., beta blockers, statins, opioids), with unknown implications. Finally, Schmeusser et al.104 reported that eligibility of Black patients for participation in cancer clinical trials could decrease significantly when eGFR is estimated without a race coefficient.
Five studies removed race from algorithms other than eGFR. Baugh et al.68 compared spirometry measures with and without race-based equations in patients with chronic obstructive pulmonary disease. The authors found that race-specific algorithms slightly overestimated healthy lung function in Black patients compared with that of non-Hispanic White patients; predicted mean forced expiratory volume (FEV1) was 5 percent higher and predicted mean forced vital capacity (FVC) was 2.3 percent higher in Black patients. Removing race led to more accurate assessment of lung function, with Black patients demonstrating a mean FEV1 that was 7.9 percent lower and FVC 16.3 percent lower, than non-Hispanic White patients. Another study examined the impact of spirometry equations with or without race and ethnicity on predicting chronic lower respiratory disease (CLRD) events and all-cause mortality in Asian, Black, Hispanic, and White patients.71 Findings suggest that percentage predicted FEV1 and FVC with race-specific spirometry equations did not appear to improve the prediction of CLRD events or all-cause mortality compared with race-neutral equations.71 The C-statistic for the standard race-specific spirometry equation predicting CLRD-events was 0.71 for FEV1 and 0.61 for FVC. Authors found very similar C-statistics (0.72 and 0.62) for the race-neutral equation. Findings were similar for all-cause mortality.
Kidney donation was the subject of two studies that examined the KDRI and KDPI.97,98 These are interrelated indices that predict graft failure following transplantation, using donor characteristics including race. Published in 2022 by separate research teams analyzing data from the Scientific Registry for Transplant Recipients, both studies had similar findings. They reported that removing race as a variable did not affect the predictive accuracy of the algorithms but did result in a small increase of approximately 5098 to 7097 kidneys from Black donors that might become available annually. Finally, a 2021 study78 evaluated an algorithm designed to predict diagnosis and treatment needs associated with postpartum depression. Using data on Medicaid beneficiaries, the authors compared three mitigation strategies to improve the model and yield more accurate prediction. The study modeled three alternative versions of the algorithm: without race; with addition of a statistical adjustment designed to limit race-based effects, and following recalibration based on a reweighing of key population subgroups. The latter two strategies are described below in the respective sections addressing those approaches. The authors found that removing race improved the algorithm’s accuracy, and this approach was more effective than adding a statistical adjustment but less effective than recalibrating the model with more diverse patient data.
3.3.3.2. Replacing Input or Outcome Variables
Five studies evaluated the impact of replacing initial algorithmic variables (either inputs or outcomes) with alternative variables. Three studies replaced race in eGFR with biological measures, and one replaced race with a biologically relevant genotype in the KDRI. The fifth study, by Obermeyer et al., demonstrated how variables other than race could unintentionally affect healthcare disparities.
Substantial interest in alternatives to race-based GFR estimation has led to much recent research. We identified three studies meeting our review criteria that also represent the current research addressing eGFR. A 2019 study86 identified a panel of metabolites, excluding creatinine (and thereby the race-based coefficient), that estimated GFR as effectively as using either creatinine or cystatin C alone (although it was less effective than a combination of creatinine and cystatin C). This algorithm was developed using data only from Black patients and validated using a diverse population data set. In 2021, the Chronic Kidney Disease Epidemiology Collaboration examined the effect of removing race from eGFR calculations while adding cystatin C and creatinine.73 They found that, when both cystatin C and creatinine were used, the new algorithm was more accurate than the previous race-based eGFR equation using only creatinine. The new version with creatinine (but not cystatin C) increased the estimates of population-level chronic kidney disease for Black people, with similar or lower estimates among other racial and ethnic populations. Also in 2021, the Chronic Kidney Disease Epidemiology Collaboration (CKD-EPI) published a study23 examining the effect of replacing race in eGFR with measures of cystatin C, beta-trace protein, and beta2-microglobulin, with or without creatinine. They discovered that replacing race with this combination of input variables resulted in GFR estimation that is equivalent to that derived from the race-based eGFR algorithm.
On a related topic but addressing a different algorithm, a 2017 study sought to replace race in the KDRI.89 The authors removed race and added a measure for the apolipoprotein L1 genotype, which is associated with kidney disease in Black patients. They found that replacing race with the genotype marker improved the index’s ability to predict graft failure (area under the curve improved from 0.59 to 0.60 at 1–5 years after transplantation). Because the study enrolled only Black patients, it did not provide data on the effect on race differences.
Finally, the landmark study by Obermeyer et al.5 is addressed above in KQ 1 because it found racial differences in access to a care management program, resulting from unintentional bias in model design that used cost as a proxy for clinical need. After identifying the problem, the model developers sought to mitigate the issue by replacing the previous model with three new algorithms that better predict clinical need: degree of chronic conditions, avoidable costs, and total costs. None of the algorithms used input variables based on race and ethnicity. The new algorithms significantly increased access to disease management resources for Black patients.
3.3.3.3. Adding Input Variables
Eight studies added input variables to mitigate or avoid potential bias resulting from clinical algorithms. Two of these studies added race and ethnicity to the Framingham Risk Score (FRS) equations to address concerns about underestimating cardiovascular risk in Black patients. In one study,87 the authors found that the atherosclerotic cardiovascular disease equations (ASCVD) algorithm, which added race and other factors to FRS, resulted in a general reduction of differences between Black and White patients on two measures of subclinical vascular disease. The reductions in race differences were generally greater for low-risk patients than high-risk patients. In contrast, a study of patients with hypertension95 found that adding race did not lead to improvements in cardiovascular risk classification for either Black or White patients. Another study91 added an input variable labeled “African American ethnicity” to a stroke risk prediction tool that included age, gender, and morbidity. The authors found that the new model was slightly better (1.2 percent closer) at predicting true stroke risk in Black patients, while the algorithm’s predictive ability for White patients was unchanged (<0.1 percent closer).
Race is not the only input variable that researchers have added to algorithms to address disparities. A 2021 study added polygenic risk scores to the ASCVD algorithm and evaluated patients in multiple racial and ethnic populations.81 The authors found integrating polygenic risk resulted in significant net classification improvement for patients who self-reported as White, Black/African American/Black Caribbean/Black African, South Asian, or Hispanic. They suggest that incorporating genetic risk markers into ASCVD could lead to more accurate risk prediction for patients of all racial, ethnic, and ancestral backgrounds. Another study simulated the effect of adding up to 10 different biomarkers (measuring factors such as adiposity, inflammation, glycemic control, and more) to the ASCVD and FRS algorithms.90 Using data based only on Black patients, the study found that incorporating the biomarkers provided no substantial benefit over the original algorithms for classifying cardiovascular risk.
Our review identified only one randomized controlled trial addressing the KQs. This 2013 multicenter study assessed incorporating genotype variables into warfarin dosing algorithms during the first 4 weeks of anticoagulation therapy.93 Overall, the study found that algorithms informed by genotype information performed no better than traditional clinical algorithms. However, the genotype-informed algorithms led to poorer management in Black patients than in White patients. Thus, adding genotype variables would actually exacerbate disparities. Conversely, Lindley et al.106 reported that standard warfarin dosing algorithms may overestimate dosing by 30 percent in patients of African ancestry with a specific allele variant. Incorporating this single-nucleotide variation could improve the safety of warfarin dosing and potentially reduce health disparities.
One study modified a lung cancer screening tool by adding a measure of life-years gained from screening.74 The authors reported that the revised algorithm reclassified 3.5 million people as eligible for lung cancer screening. Importantly, 22 percent of the newly eligible were Black, and differences between Black and White patients would be greatly reduced by implementing this modified algorithm (from 13 percent difference to 0 percent in preventable lung cancer deaths, and from 16 percent difference to 1 percent in life-years gainable). Slight reductions occurred in the White-Hispanic difference (by 3–4 percent), but no change occurred in the White-Asian difference.
Finally, a study based on Medicare beneficiary data examined the effect of using SDOH to predict healthcare use, costs, and mortality.85 The authors compared models that used four different sets of input variables: 1) sex and age only; 2) sex, age, and morbidity; 3) sex, age, morbidity, and seven SDOH measures; and 4) only the SDOH measures (which included education, economic status, financial strain, marital status, access to healthcare, rural or urban location, and alcohol abuse). The model that included SDOH in addition to the other input variables performed best at predicting risk of hospitalization and death for both Black and White patients. Moreover, the models without SDOH tended to underestimate risk of hospitalization and overestimate risk of death for Black patients, while overestimating the likelihood of hospitalization and underestimating risk of death for White patients.
3.3.3.4. Changing the Patient Mix Used for Development and Validation
An algorithm’s components and construct are substantially affected by the characteristics of the patients used for derivation and validation. When relevant populations are not adequately represented during development, an algorithm may reflect and contribute to racial differences. Our review identified four studies that attempted to mitigate bias by recalibrating algorithms based on a different patient mix than initially used. Two of these studies focused on ASCVD equations for cardiovascular risk. In 2018, a seminal study by Yadlowsky et al.88 revised the pooled cohort equations using more heterogeneous patient data. The authors reported that the original algorithm overestimated risk for most patients, leading to unnecessary treatment, while the new version was significantly better at predicting risk, especially for Black people. These findings were supported two years later by a study that found the revised algorithm eliminated significant differences in risk assessment and recommendations for statin use between Black and White patients.84
We briefly described Park et al.’s algorithm for predicting postpartum depression78 in the section above on removing race-based input variables. The authors tested two additional mitigation strategies in their study: they incorporated a statistical technique to adjust the algorithm (described below), and they reweighed key population groups to better calibrate their model. Reweighting the algorithm with diverse patient data proved the most effective of their three strategies, leading to more accurate predictions that were less likely to produce disparities.
Finally, we identified a study that modified a Donor Risk Index for liver transplant.94 In this case, the initial algorithm had been developed using a diverse population and included Black race among seven input variables predicting risk of graft failure. The authors sought to create an algorithm that would better predict risk specifically in Black patients with a diagnosis of hepatitis C. They revised the algorithm using data drawn solely from that subpopulation and reported that the new strategy resulted in more accurate risk assessment, including reclassification of more than a quarter of patients.
3.3.3.5. Developing Separate Algorithms by Race
Two studies went a step beyond recalibration with representative data and developed different algorithms for Black and White patients. Limdi et al. compared two models for developing warfarin dosing algorithms based on multiple clinical and genetic factors.92 The authors found that separate algorithms for Black and White patients were better at predicting correct dosing levels than traditional, combined algorithms that adjust for but are not stratified by race. A study of opioid misuse that was also described in KQ 1108 took a related approach. The authors tested two mitigation strategies: develop separate thresholds for Black and White patients and recalibrate the model for each racial subgroup. They found that both approaches eliminated differences in false-negative predictions between Black and White patients. The first mitigation technique involved creating separate thresholds for each racial and ethnic group. Reducing the threshold from 0.3 to 0.2 for Black patients reduced the FNR to 0.25 (95 percent CI: 0.20 to 0.30) and “in closer approximation to the White subgroup with overlapping confidence intervals.”108 (Data for other racial and ethnic groups were presented only in graphical form, so exact values cannot be determined.) The second technique, which involved recalibration by racial and ethnic group, produced results virtually identical to those of the first: the FNR was 0.24 (95 percent CI: 0.19 to 0.29) among Black patients and 0.21 (95 percent CI: 0.15 to 0.27) among White patients.
3.3.3.6. Refining Statistical and Analytic Techniques
Modifying the technical aspects of algorithms, including statistical methodologies and analytic approaches, composes the final mitigation strategy described in two studies we reviewed. As described above, an algorithm to predict postpartum depression was tested against three mitigation approaches.78 Removing race improved the algorithm’s accuracy and may have reduced the likelihood of contributing to disparities, while recalibrating the model with diverse patient data was even more effective. The third strategy involved adding an adjustment term to the model intended to limit the impact of including a race-based input variable (what the authors termed “Prejudice Remover”). This modification had no significant effect on outcomes.
We also discussed above the work by Yadlowsky et al.88 to update the ASCVD algorithm with more diverse patient data. The authors also adjusted the statistical methodology used in the equations, employing elastic net regularization to avoid overfitting the data and to address concerns about proportional hazards assumptions. They found that these adjustments improved accuracy but to a lesser degree than recalibration with diverse patient data. Foryciarz et al.96 also addressed the ASCVD algorithm, adjusting estimation of risk through group calibration and equalized odds. They found that recalibrating by subgroups could increase accuracy for a given group while increasing disparities between groups, and use of an equalized odds constraint led to poorer calibration for the overall model.
3.4. Contextual Question 1. How widespread is the inclusion of input variables based on race and ethnicity in healthcare algorithms?
The evidence base presented throughout this report offers an insightful but limited view of the landscape of race and ethnicity in healthcare algorithms. The 31 distinct algorithms (and their various iterations) examined in KQ 1 and KQ 2 and the 6 described in CQ4 affect cardiology, nephrology, oncology, hematology, neurology, hepatology, endocrinology, infectious disease, obstetrics, pulmonary medicine, transplant medicine, urology, addiction medicine, surgery, and mental health. They are used in primary care settings, hospital medicine, emergency medicine, and intensive care and address screening, diagnosis, treatment, prognosis, and the use and allocation of healthcare resources. Seventeen of the algorithms include race and ethnicity as an input variable, and five include measures such as SDOH, healthcare costs, or healthcare utilization that may correlate with or serve as proxies for race and ethnicity.
However, this is just the tip of the iceberg, because our review was limited to studies that met specific inclusion criteria (especially related to study design and reported health outcomes). To gain a wider perspective, we briefly examined excluded studies. Of the 278 studies excluded during full-text review, 156 were not included due to study design (these were usually derivation studies without external validation, indicating clinical algorithm development but not necessarily use) or because they did not report outcomes related to access to care, quality of care, or health. Similarly, the 6 algorithms examined in CQ 4 were selected from a final pool of 55 algorithms that were initially identified after reviewing hundreds of potential resources. Although a comprehensive analysis of the excluded studies and examples was beyond the scope of this report, a cursory review revealed that hundreds of them included clinical algorithms that were similar or identical to those that were included in the KQ 1 and 2 results. Also, several studies were conducted in specialties that were not included in the evidence for KQs 1 and 2, such as orthopedics, gastroenterology, and pain medicine. While we did not explore whether any of the excluded studies explicitly included race-based input variables within algorithms, algorithms clearly could affect health and healthcare disparities can be found in every medical specialty, healthcare setting, and patient population.
Our findings are reinforced by websites such as MDCalc, a widely used repository for healthcare algorithms, formulas, and calculators. MDCalc, which does not develop algorithms but aims to make them readily available to clinicians, has more than 700 entries. Despite this scope, as of the writing of this report, only 14 included race and ethnicity as an input variable. It is unknown how many algorithms include input variables that might be proxies for race and ethnicity.
We were able to ascertain the original source behind the development of many but not all the algorithms in our review and found that clinical research teams accounted for at least 12 of the algorithms. These were typically investigators managing clinical trials or large observational studies who then promulgated an algorithm derived from the data they collected. At least nine were developed by medical specialty societies or other organizations tasked with setting healthcare policy, such as the United Network for Organ Sharing and USPSTF. At least five of the algorithms were published by academic researchers using machine learning, artificial intelligence (AI), or other data-mining strategies to develop and validate risk prediction algorithms. Three algorithms were created by health plans using large member datasets, and two algorithms examined in CQ 4 were built by EHR vendors.
Our review was limited in scope; therefore, the algorithms we examined do not fully represent the larger environment. For example, we evaluated only two EHR algorithms, but our KIs, TEP, and SMEs indicated that there are probably hundreds of clinical algorithms embedded in the systems used by many academic medical centers. Obermeyer et al.5 demonstrated how there may be unforeseen effects of algorithms that can influence patient care on a broad scale, but little has been published about these algorithms. Meanwhile, larger health systems are increasingly devoting resources to develop homegrown algorithms for managing healthcare delivery, often aimed at reducing readmissions or predicting which patients are at highest risk for sepsis or death. Smaller hospitals, although unlikely to have internal capacity for such efforts, may be likely to use algorithms already embedded by vendors in their EHRs.116
We also found that at least 18 of the algorithms we reviewed are or were previously endorsed by medical specialty societies, included in clinical practice guidelines, and/or used by quasi-regulatory agencies such as United Network for Organ Sharing. Such designations are important mechanisms to disseminate clinical algorithms, although it is difficult to evaluate the extent of their use.
Finally, AI might dramatically alter how algorithms are developed and used and how healthcare is delivered in countless ways. A recent journalistic investigation117 revealed that AI-informed algorithms used by Medicare Advantage plans resulted in widespread denial of care to seniors. While AI and machine learning tools are recognized as a source of significant concern,118–121 rigorous, real-world research is lacking. We identified only five studies meeting our inclusion criteria that evaluated algorithms that were derived or tested with the use of AI or machine learning tools, and these studies focused on AI tools used during development and validation of algorithms, rather than implementation. Nevertheless, research on AI is growing rapidly, and 36 of the 278 studies we excluded during full-text review (13 percent) presented research related to AI or machine learning in the context of algorithms. CQ2 highlights several efforts to address the challenges of new AI tools.
3.5. Contextual Question 2. What are existing and emerging national or international standards or guidance for how algorithms should be developed, validated, implemented, and updated to avoid introducing bias that could lead to health and healthcare disparities?
The recent evolution of AI as a major source of clinical algorithms has led to the emergence of nascent standards, principles, and frameworks to address growing concerns about AI’s ethical, legal, and social impacts.122–124 Discussions with our KIs and TEP revealed that every relevant sector of healthcare and health technology – from EHR vendors and medical device manufacturers to medical specialty societies and clinical guideline panels, from academic medical centers and community health centers to health plans and employers, from researchers and patient advocacy groups to federal and state agencies – all recognize both the value and inevitability of new standards for healthcare algorithms. In recent years, multiple federal agencies have grappled with the broad challenges of healthcare AI and the specific difficulties posed by algorithms.125,126 In August 2022, California’s Attorney General launched an investigation into potential racial and ethnic biases in healthcare algorithms used across the state.127 In November 2021, the New York City Department of Health and Mental Hygiene launched a Coalition to End Racism in Clinical Algorithms (CERCA), designed in part “to end race adjustment, monitor the impact on racial health inequities, and engage patients whose care was negatively impacted by it”.128 Simultaneously, health technology companies, and the technology sector more generally, have sought to design self-regulatory strategies to reassure consumers and policymakers that they are acting responsibly.129,130 Concurrent with these efforts has been the rise of a specialized field of research focused on strategies to identify, mitigate, and prevent harms associated with AI and healthcare algorithms.49,118,120,121,131,132 Moreover, these trends are not unique to the United States but are highly visible in other countries as well.133,134
In Table 17, we summarize 10 policy briefs, white papers, and research articles that provide principles or frameworks that could help guide future development and evaluation of clinical algorithms. All have been published in the past 3 years and primarily reflect expert and consensus opinion. Several of these resources are not specific to healthcare or medicine but provide guidance that readily translates across disciplines. Two documents represent U.S. federal regulatory agencies: the Food and Drug Administration (FDA)135 and the National Institute of Standards and Technology.136 Our KIs and TEP repeatedly discussed FDA as a potential home for formal regulation of algorithms, similar to the agency’s role with medical devices. We also identified two resources developed by Google129 and Microsoft.130 While not focused exclusively on healthcare, both documents include widely applicable recommendations. 137
Another publication reflected the work of the Algorithmic Justice League,137 a research and advocacy organization that promotes awareness of AI-fueled bias and designs algorithms to mitigate harms. Finally, we include manuscripts and material prepared by five academic or nonprofit research institutions, most prominently the Algorithmic Bias Playbook123 published by the Chicago Booth Center for Applied Artificial Intelligence. The Playbook lays out a step-by-step process for organizations to mitigate harmful consequences of biased algorithms.
Several themes, including fairness, transparency, representativeness, and accountability, are major principles cited throughout the guidance we reviewed. Multidisciplinary and diverse teams that include representatives of populations that may be most at risk for the harms caused by algorithmic bias are a key element as well. It is noteworthy that all these resources focus on developing algorithms using AI capabilities, although most of the algorithms we examined in KQ 1 and 2 were derived and validated outside that context. Nevertheless, the principles and guidance presented here may be applicable to many types of clinical algorithms.
Table 17
Guidance, standards, and recommendations.
3.6. Contextual Question 3. To what extent are patients, providers (e.g., clinicians, hospitals, health systems), payers (e.g., insurers, employers), and policymakers (e.g., healthcare and insurance regulators, State Medicaid directors) aware of the inclusion of input variables based on race and ethnicity in healthcare algorithms?
Patients, providers, payers, and policymakers all have vital roles in addressing the challenges inherent at the intersection of race, healthcare, technology, and society. Recently published research33 and discussions with our KIs, TEP, and SMEs explored the perspectives of these key stakeholder groups and highlighted several important considerations.
The KIs and TEP were in consensus that patients are generally unaware of healthcare algorithms and how they might lead to racial and ethnic disparities in health and healthcare. People typically view healthcare through the lens of their own experiences as patients (or as family members and friends of patients); their perspectives may be shaped strongly by their interactions with doctors and nurses, hospital and health clinics, pharmacies, and insurers. Low health literacy is also a barrier to understanding health and healthcare. Moreover, our KIs and TEP suggested that many Americans (including BIPOC communities) do not conceptualize race as a social construct or understand the mechanisms and effects of structural racism. Not surprisingly, algorithms, often complex and embedded in EHRs and clinical guidelines, are not on patients’ minds. Recent controversies about eGFR and other algorithms may have attracted broad attention, but our KIs and TEP did not believe that this has significantly affected public opinion or patient awareness. These perspectives were reinforced in a recent qualitative study that interviewed patients about race and healthcare algorithms.138 The authors reported that few participants were aware that race may be included in common algorithms, and patients were almost universally opposed to the concept of using race to modify clinical equations.
Patient perspectives on AI-informed tools in healthcare, construed broadly, have also been studied recently. A survey of 926 people, conducted in 2019 and published in 2022 by researchers at the Yale School of Medicine and Weill Cornell Medical College, found that 55 percent believed that AI will eventually make healthcare somewhat or much better.139 However, 91 percent of respondents were somewhat or very concerned about AI’s potential to result in misdiagnosis, and 71 percent expressed privacy concerns. Additionally, while White and non-White patients did not differ in their overarching opinions regarding AI in healthcare, non-White participants were more likely to be very concerned about potentially negative consequences. Another survey, conducted in December 2022 by the Pew Research Center,140 attracted attention for reporting that 60 percent of patients were uncomfortable with their healthcare provider relying on AI tools to support care. Thirty-eight percent of respondents believed that AI would lead to better care overall, while 33 percent thought outcomes will worsen, and 27 percent expected no change. Seventy-five percent worried that healthcare will adopt AI too quickly, without fully understanding the risks that patients may face. However, 70 percent of people thought that racially biased treatment is a major or minor problem in healthcare, and 51 percent of these respondents were optimistic that AI can reduce bias (33 percent thought things were unlikely to change, and 15 percent expected AI to lead to more biased care).
Two current healthcare trends may have the potential to further shape patient perspectives on healthcare algorithms, in the view of our KIs and TEP. First, increased emphasis on patient-centered care and shared decision making has begun to expand the types of conversations that patients have with their providers, ideally leading to greater trust and transparency. Second, scientific advances continue to pave the way for personalized medicine and, along with broad public interest in personal genetic profiles, may lead to patients (and providers) asking more questions about interactions between genetics, ancestry, race, ethnicity, and health. Indeed, some of our KIs reported that BIPOC patients are increasingly seeking information about treatments that might have unique benefits, or harms, for specific populations. Taken together, these conditions may enable patients to better understand the role of algorithms in guiding their care and to initiate important conversations about how algorithms are developed and used.
Compared with patients, providers (e.g., clinicians, hospitals, health systems) have greater familiarity with healthcare algorithms, but their understanding is also limited in significant ways. Our discussions revealed that front-line clinicians routinely use algorithms in much the same way as imaging devices or pharmaceuticals: they learn how and when to incorporate these algorithms or treatments into medical practice (typically during their medical training or, later in their careers, from colleagues or vendors) without needing to know every component or ingredient or understanding in-depth how such items are developed, tested, or manufactured. Clinicians generally defer to the trusted institutions of their field, such as regulatory agencies, specialty societies, and academic medical centers, to vet the safety and assess the utility of the drugs they prescribe and devices they use. Algorithms are largely viewed in a similar manner— as tools that can be used effectively without knowing how their input variables are selected, defined, or adjusted, or what patient populations were used to develop and test their efficacy. Hospitals and health systems increasingly deploy algorithms that are embedded in EHR systems but rarely seek to review algorithmic formulas or review underlying evidence of effectiveness or possible algorithmic bias.
At the same time, many hospitals and health systems adapt existing algorithms or develop and test homegrown algorithms, but this does not necessarily lead to greater scrutiny of potential bias. Healthcare institutions have only recently begun to recognize the potentially harmful role of algorithms. Regulatory or professional guidance on these concerns is only beginning to emerge (as demonstrated in CQ 2). Unfortunately, as with American society broadly, many healthcare professionals view race as a biological concept rather than a social construct, potentially reinforcing common biases.
Our KIs and TEP identified medical education as a vital locus for changing these dynamics. Medical school curricula and graduate medical education can begin to emphasize critical thinking about algorithms (and clinical practice guidelines and EHRs that often embed them in practice). More attention can be given to teaching human genetics. And medical schools and academic medical centers could endeavor to debunk historical stereotypes about race and biology. Efforts already underway by the Association of American Medical Colleges and American Medical Association to address entrenched institutional racism in medicine and medical education are major steps in this direction.141–143
Healthcare payers, especially insurers and government-funded healthcare programs, represent a key sector responsible for developing algorithms that often focus on cost reduction and resource allocation. However, they may also tend to lack a sophisticated understanding of how algorithms may contribute to bias and disparities. They often rely on the data they collect to lead them in the right direction, perhaps assuming that patterns linking patient characteristics, healthcare use, and outcomes sufficiently reflect real healthcare needs, without considering the complex social systems that influence access and barriers to care. The recent revelations about potential harms associated with AI-informed algorithms used by Medicare Advantage plans underscores such concerns. Additionally, payers may not be ideal settings for driving change. Health insurers must grapple with the decentralized nature of their operations and the challenges of conducting business across each state’s varied regulatory system. When we sought input on this report from commercial insurers, we found deeply held concerns about the proprietary nature of their operations and data. We also discovered that state-level entities of large, national insurers are substantially autonomous and may not always coordinate or align innovations.
Finally, our discussions addressed the roles of policymakers in confronting the challenges of healthcare algorithms. As described above in CQ 2, FDA and the National Institute of Standards and Technology have taken on critical roles in leading federal activity. Our KIs and TEP agreed that both agencies, especially FDA given its specific role in the healthcare sector, are well-positioned to address these issues. The Center for Medicare & Medicaid Services and the Office of the National Coordinator for Healthcare Information Technology were also suggested as sources of leadership and innovation. Our experts agreed strongly that the federal government will inevitably need to play a key role in setting standards and guidance to ensure that healthcare algorithms do good without exacerbating disparities. Moreover, we heard that all sectors of healthcare – including algorithm developers, commercial vendors, and end-users – anticipate federal guidance, and would generally prefer a stable regulatory environment to the current state of uncertainty.
3.7. Contextual Question 4. Select a sample of approximately 5–10 healthcare algorithms that have the potential to impact racial and ethnic disparities in access to care, quality of care, or health outcomes and are not included in KQs 1 or 2. For each algorithm, describe the type of algorithm, its purpose (e.g., screening, risk prediction, diagnosis), its developer and intended end-users, affected patient population, clinical condition or process of care, healthcare setting, and information on outcomes, if available.
We identified six algorithms to explore for CQ 4, which are described in detail in Tables 18–24. Selected algorithms encompassed four conditions: heart failure, end-stage renal disease, cardiac surgery, and HIV. Two focused on EHR vendor-developed algorithms (Cerner Corp. and Epic Systems Corp.) and were selected based on topics of critical relevance to healthcare inpatient settings and patients: 30-day hospital readmissions (Cerner)144 and Pediatric Hospital Admissions and ED visits (Epic Systems).145 We reviewed each algorithm to understand the extent of racial and ethnic biases that may have been introduced during the problem formulation and variable inclusion justification, algorithm development, and translation, dissemination, and implementation phases. In general, while algorithm developers did provide information on the rationale for the algorithm and the intended use for clinical practice, most developers did not provide an adequate justification for included variables (e.g., literature support, expert panel). Related to the development phase (Table 3, stage 3), all identified studies consistently reported on internal and external validation. However, several elements related to data selection and management and model training and development (Table 3, stage 1 and 2) were inconsistently addressed. For instance, for missing data, some developers simply stated that missing data were omitted and did not describe trends observed in missing data (less desirable for reproducibility and transparency), whereas other developers provided information on the distribution of missing data and how missing data were imputed (more desirable for reproducibility and transparency). In one case (Cerner, 30-day hospital readmission risk prediction model), the developers provided no information for missing data. The impact on race and ethnic biases for this algorithm may be compounded depending on the number of hospitals implementing this algorithm and whether these hospitals perform their own external validation. Furthermore, algorithm developers did not list input variables used and instead referred to categories of variables (e.g., “demographic,” “lab”). This precluded extensive analysis, which was completed for the other described algorithms. We performed limited searches of the literature to assess race and ethnic biases introduced during the algorithm translation, dissemination, and implementation phase. While several calculators were developed to support widespread use of the algorithms (Appendix Table E-1 through E-3), these calculators lack detail to understand how the algorithm may be used in a clinical setting. We found only one instance (unpublished) that described how the algorithm was implemented in a clinical setting (including in an EHR decision support design) (Appendix Figure E-4); the authors of this work did not describe outcomes by race or ethnicity. Lack of published data prevented us from assessing race and ethnic biases that may result from provider interpretation or lack of action (i.e., interaction bias). Overall, vendor-developed algorithms (Cerner or Epic) had the least information available in the published literature, likely due to the work’s proprietary nature, which hampered our assessment of race and ethnic biases at any phase.
We did not identify any prospective clinical validations for any models, let alone a subgroup analysis by race, which limits our understanding of how these models actually affect care. This leaves a gap related to establishing effectiveness for any group, especially marginalized subgroups. None of these models are FDA-approved and likely do not qualify for regulation under current federal standards. Results are organized into seven sections, including tables:
- Table 18. Overview of Algorithms Included to Address CQ 4
- Table 19. Potential Scale and Reach of Algorithm Impact on Populations
- Table 20. Race and Ethnicity Definitions and Standards
- Table 21. Algorithm Model Performance
- Table 22. Evidence, Evidence Quality, Data Sources, and Study Populations Used for Algorithm Development and Validation
- Table 23. Bias Mitigation Strategies Completed by Algorithm Developers
- Table 24. Approaches and Practices for Implementing, Adapting, or Updating Algorithms as Specified by Algorithm Developers
Table 18 summarizes key information about the algorithms: developer, year, clinical setting, intended user, key outcome, race variables included, whether algorithm developers included definitions for race and ethnicity, and the algorithm’s potential impact on racial and ethnic disparities when translated for use in clinical practice. See Appendix E for calculator input variables and results by race.
Table 18
Overview of algorithms included to address Contextual Question 4.
Results in Table 19 assessed the scale of potential impact of the algorithms on patient populations. A rubric was developed that accounted for condition prevalence, whether the algorithm was recommended by a professional clinical society or government organization, whether the algorithm was implemented within an EHR system, publication metrics (e.g., citations, downloads), and whether the algorithm had been implemented in a widely used online point-of-care clinical resource. All algorithms, except for the Dialysis Mortality Risk calculator,147 were rated as having large potential patient impact. The impact scale for the Dialysis Mortality Risk calculator and the two EHR vendor algorithms was unable to be assessed due to several unknown key elements.
Table 19
Potential scale and reach of impact on populations.
3.7.1. Contextual Question 4a
- a.
If race and ethnicity is included as an input variable, how is it defined? Are definitions consistent with available standards, guidance, or important considerations identified in CQ 2?
Table 20 below contains information on how each algorithm developer defined race and ethnicity and whether those definitions or categories were consistent with available standards. The last column provides context as to how data were captured at the time of algorithm development and relevant data quality. Except for the Society of Thoracic Surgeons’ (STS) algorithm for CABG, algorithm developers did not specify race and ethnicity definitions, nor were race and ethnicity consistent with available standards for race categories. Four algorithms were developed or validated using data from datasets containing data abstracted from multiple EHR systems or from multiple clinical settings.146–148,152 In three cases, developers did not specify how data were collected or whether participants were directly asked to provide race and ethnicity responses.146,147,152
Table 20
Race and ethnicity definitions and standards.
- b.
For healthcare algorithms that include other input variables in place of or associated with race and ethnicity, how were these other variables defined? Are these definitions consistent with available standards, guidance, or important considerations as identified in CQ 2? Were racial and ethnic variables considered during initial development or validation?
All non-EHR vendor sample algorithms included race or ethnicity. Inclusion criteria for the Pediatric Hospital Admission and ED Visit risk algorithm145 limit data to patients with previous healthcare utilization (one in-person ambulatory encounter and at least one additional ambulatory encounter, ED visit, or hospitalization in the 2 years before the prediction). The algorithm includes Medicaid status as an input variable. The Cerner Hospital Readmission Risk algorithm144 developers describe analysis of prior hospital utilization data but do not elaborate on how these data were used in the derivation process.
- c.
For each healthcare algorithm, what methods were used for development and validation? What evidence, evidence quality, data sources, and study populations were used for development and validation?
Tables 21 and 22 contain information on algorithm performance metrics and the underlying data used to develop the algorithm. Performance data were mostly consistently reported. Most studied reported on goodness of fit, C-statistics, and predicted versus observed probability plots. Sensitivity and positive predictive value were provided only for the Epic Pediatric Admissions and ED Visit Risk algorithm.145 All studies performed validations.
Table 21
Algorithm model performance.
Table 22
Evidence, evidence quality, data sources, and study populations used for algorithm development and validation.
- d.
Are development and validation methods consistent with available standards, guidance, and strategies to mitigate bias and reduce the potential of healthcare algorithms to contribute to health disparities?
Table 23 summarizes the bias mitigation activities completed by algorithm developers. We defined mitigation strategies as evidence of external validation in a population that differed from the derivation and internal validation sample datasets, evidence of subgroup analysis by race and ethnicity.
Table 23
Bias mitigation strategies completed by algorithm developers.
- e.
What approaches and practices are there to implement, adapt, or update each healthcare algorithm?
Table 24 below summarizes the approaches and practices developed by algorithm developers for implementing, adapting, or updating algorithms. We performed a limited search for each algorithm to identify subsequent publications by algorithm developers that contained guidance for general use, adaptation, or updating. We found examples of developer-suggested input variables to include in subsequent implementation or adaptations.147 Only EHR algorithm authors provided recommended clinical thresholds for use in clinical care.
Table 24
Approaches and practices for implementing, adapting, or updating algorithms as specified by algorithm developers.
- Overview
- Key Question 1. What is the effect of healthcare algorithms on racial and ethnic differences in access to care, quality of care, and health outcomes?
- Key Question 2. What is the effect of interventions, models of interventions, or other approaches to mitigate racial and ethnic bias in the development, validation, dissemination, and implementation of healthcare algorithms?
- Contextual Question 1. How widespread is the inclusion of input variables based on race and ethnicity in healthcare algorithms?
- Contextual Question 2. What are existing and emerging national or international standards or guidance for how algorithms should be developed, validated, implemented, and updated to avoid introducing bias that could lead to health and healthcare disparities?
- Contextual Question 3. To what extent are patients, providers (e.g., clinicians, hospitals, health systems), payers (e.g., insurers, employers), and policymakers (e.g., healthcare and insurance regulators, State Medicaid directors) aware of the inclusion of input variables based on race and ethnicity in healthcare algorithms?
- Contextual Question 4. Select a sample of approximately 5–10 healthcare algorithms that have the potential to impact racial and ethnic disparities in access to care, quality of care, or health outcomes and are not included in KQs 1 or 2. For each algorithm, describe the type of algorithm, its purpose (e.g., screening, risk prediction, diagnosis), its developer and intended end-users, affected patient population, clinical condition or process of care, healthcare setting, and information on outcomes, if available.
- Results - Impact of Healthcare Algorithms on Racial and Ethnic Disparities in He...Results - Impact of Healthcare Algorithms on Racial and Ethnic Disparities in Health and Healthcare
- Introduction - Cervical Degenerative Disease Treatment: A Systematic ReviewIntroduction - Cervical Degenerative Disease Treatment: A Systematic Review
- UI-H-DH1-awq-p-13-0-UI.s1 NCI_CGAP_DH1 Homo sapiens cDNA clone IMAGE:5893116 3',...UI-H-DH1-awq-p-13-0-UI.s1 NCI_CGAP_DH1 Homo sapiens cDNA clone IMAGE:5893116 3', mRNA sequencegi|19753715|gnl|dbEST|11861849|gb|B 38.1|Nucleotide
- Treating Prostate Cancer - Comparative Effectiveness Review Summary Guides for C...Treating Prostate Cancer - Comparative Effectiveness Review Summary Guides for Consumers
Your browsing activity is empty.
Activity recording is turned off.
See more...