Included under terms of UK Non-commercial Government License.
NCBI Bookshelf. A service of the National Library of Medicine, National Institutes of Health.
Farmer AJ, Stevens R, Hirst J, et al. Optimal strategies for identifying kidney disease in diabetes: properties of screening tests, progression of renal dysfunction and impact of treatment – systematic review and modelling of progression and cost-effectiveness. Southampton (UK): NIHR Journals Library; 2014 Feb. (Health Technology Assessment, No. 18.14.)
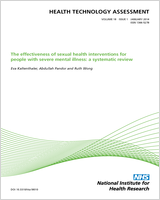
Optimal strategies for identifying kidney disease in diabetes: properties of screening tests, progression of renal dysfunction and impact of treatment – systematic review and modelling of progression and cost-effectiveness.
Show detailsIntroduction
Despite strong evidence that the development of impaired renal function in patients with diabetes is associated with poor disease outcomes, evidence supporting current practice to detect the onset of kidney disease is limited. Annual screening for adults with diabetes to detect the onset of raised levels of urinary albumin (microalbuminuria), and thus identify development of early kidney disease, is recommended in clinical guidelines, with suggestions of more frequent screening for those at increased risk of developing kidney disease. Although the existence of a beneficial treatment, in the form of angiotensin-converting enzyme inhibitor/angiotensin 2 receptor blocker (ACEi/A2RB), is recognised, the extent to which there is benefit for subgroups defined by type of diabetes and baseline presence or absence of microalbuminuria is uncertain. Similarly, there is limited evidence to support recommendations of the frequency with which both serum creatinine and urinary albumin, most commonly measured as a ‘spot’ albumin-to-creatinine ratio (ACR), should be measured. Average rates of progression are slow but highly variable, and, in addition, there is wide variation in the results obtained from a single individual over a short period of time. There is also debate about the extent to which clinical management should differ between those with and without early kidney disease.
Previous studies to determine an optimal screening interval for identifying kidney disease have used average rates of urine albumin level progression to inform their estimates. However, when the rate of disease progression is low compared with the variability of the measure used to monitor progression, the majority of positive tests (in this case, diagnosis of diabetic kidney disease, also known as nephropathy) are false positive (attributable to biological and assay variability rather than underlying disease). For these false-positive individuals, the adverse effects of treatment may outweigh the potential benefits. From a wider perspective, substantial resources may be diverted to providing treatment of minimal benefit. To establish the optimal strategy for screening, we therefore drew together data about the effectiveness of treatment and changes in albumin excretion level to carry out a cost-effectiveness modelling study.
Objectives
Our objective was to determine the clinical value and cost-effectiveness of screening programmes for kidney disease in people with type 1 and type 2 diabetes, including current practice of annual screening. Specifically, we aimed to:
- determine whether the renal benefit of ACEi or A2RB treatment varies by presence or absence of early kidney disease (baseline renal status) and type of diabetes
- quantify trends and variability in markers of early kidney disease, including estimated glomerular filtration rate (eGFR) and ACR
- estimate the proportion of diagnoses of diabetic kidney disease that are likely to be a result of measurement error and biovariability, rather than true change in underlying renal function; and hence
- estimate cost-effectiveness ratios for annual and other screening programmes for kidney disease in type 1 and type 2 diabetes.
Methods
We performed a systematic review of randomised controlled trials of ACEi and A2RB for the prevention or treatment of diabetic kidney disease. We restricted analyses to trials that clearly identified participants’ baseline renal status and type of diabetes. We pooled data for the primary outcome, comparing the ratio of urinary albumin levels at the end of each trial in those treated with ACEi or A2RB with comparator groups not treated with ACEi or A2RB, using a random effects model to estimate heterogeneity of outcomes (I2). We also carried out further limited reviews to inform our estimates of renal progression rates in patients with microalbuminuria and their variation in specified subgroups of patients.
To estimate progression of albuminuria in patients with type 1 and type 2 diabetes, the variability of the initial estimates of albumin levels, the variability of individual follow-up measurements and the variability of rates of progression, we used data from two large cohorts: the Oxford Regional Prospective Diabetes Study (ORPS) in type 1 diabetes and the Collaborative Atorvastatin Diabetes Study (CARDS) in type 2 diabetes. These estimates were derived using a hierarchical linear model for log-ACR. A similar approach was used to explore the data sets for eGFR measurements. The diagnostic strategies on which the clinical pathways were modelled were those recommended by the National Institute for Health and Care Excellence. A simulation model was used to express these results in the form of numbers of individuals identified from screening, together with estimates of the proportion identified who would have ‘false-positive’ tests and, therefore, might be unnecessarily treated.
For type 1 diabetes, we found no health-economic model that we could use to predict the impact of screening for kidney disease and, therefore, needed to develop and validate a new model. Hence, we combined our simulation model based on ORPS data with mortality, morbidity and cost data identified in the literature review to produce a cost-effectiveness model. Risk of cardiovascular disease (CVD) estimates were obtained from results of the Diabetes Control and Complications Trial; estimates of mortality post CVD events were based on a study using linked administrative data on people with diabetes from Western Australia; and information on the incidence of kidney disease and progression to CVD was obtained from studies in Finland and Italy. When diabetes-specific data were unavailable, information from other populations was used.
We examined the degree and source of parametric uncertainty and used the model to estimate lifetime outcomes of using intensive and conventional blood glucose control to illustrate its potential application. The type 1 model was validated by comparing simulated life expectancies with those calculated from a UK life table, scaled to reflect a higher mortality risk among people with type 1 diabetes. For type 2 diabetes, an existing health economic simulation model [the United Kingdom Prospective Diabetes Study (UKPDS) outcomes model] was extended to incorporate our simulation model of ACR progression based on CARDS data and the analysis of type 2 diabetes trials in our systematic review.
The current and alternative screening programmes with different screening intervals were evaluated using these simulation models to determine whether current UK guidelines for annual screening are cost-effective. Hospitalisation costs were obtained from previously published UKPDS data and adjusted to current prices using the Hospital and Community Services pay and price index. The costs of kidney disease and the costs of administering the screening test were obtained from the Chronic Kidney Disease Costing Report. Treatment and clinical costs were also included using the Prescription Cost Analysis England and Unit Costs of Health and Social Care reports. Utility values were obtained from a meta-analysis of diabetes studies and, where some diabetic complications were not reported, further published data from the UKPDS.
Results
Our systematic review identified a total of 49 trials (34,082 patients). Urinary albumin excretion (UAE) for patients with type 1 diabetes and microalbuminuria treated with ACEi or A2RB was 67% [95% confidence intervals (CI) 54% to 77%; I2 = 83%] lower on average at the end of the trial than in those patients not treated with ACEi or A2RB. There was no effect of treatment in patients with normoalbuminuria (absence of microalbuminuria). For patients with type 2 diabetes, UAE was 21% (95% CI 7% to 32%; I2 = 85%) and 27% (95% CI 15% to 38%; I2 = 87%) lower, on average, after treatment with ACEi or A2RB for those with normoalbuminuria and microalbuminuria, respectively.
Simulation modelling of the proportion of patients with true- and false-positive tests for development of impaired renal function measured with eGFR was not possible in the ORPS (type 1 diabetes) cohort, where the occurrence of a low glomerular filtration rate in the absence of microalbuminuria was so infrequent that there were no data from which to derive parameters. In the CARDS (type 2 diabetes), simulation modelling to identify progression to stage 3b kidney disease with an eGFR < 45 ml/minute in patients with normoalbuminuria suggested that, with annual eGFR measurement, 10 in 1000 patients would be identified at 6 years post diagnosis of type 2 diabetes, 80% of whom might have been either inaccurately or prematurely identified.
For type 1 diabetes, the mean increase in albumin excretion at 1 year for males with normoalbuminuria was estimated at 3% (95% CI 2% to 5%) and for females 4% (95% CI 3% to 6%). The average ACR at baseline (diagnosis of diabetes) was 0.77 mg/mmol (95% CI 0.71 to 0.83 mg/mmol) for males and 0.95 mg/mmol (95% CI 0.87 to 1.04 mg/mmol) for females. In the simulation model, the proportion of the male population diagnosed with microalbuminuria with annual testing increased from 7.7% at baseline to 20.4% at 3 years and 30.7% at 6 years. With 3-yearly testing, the proportions at 3 and 6 years, respectively, were 14.7% and 22.7%. In men and women with type 1 diabetes, 48% (95% CI 43% to 53%) and 55% (95% CI 48% to 61%) of patients, respectively, tested annually for microalbuminuria over 6 years would be inaccurately identified with microalbuminuria, and in type 2 diabetes the proportions are 36% (95% CI 32% to 42%) and 48% (95% CI 41% to 55%), respectively. Reducing the screening interval to 3-yearly would reduce this to a false-positive rate of 38% (95% CI 33% to 44%) for men with type 1 diabetes, with an increase in those individuals not identified over the 6-year period from 1.5% (95% CI 1% to 2%) to 4% (95% CI 3% to 5%).
Using the simulation models for type 1 diabetes, the incremental cost per quality-adjusted life-year (QALY) (standard deviation) of a 5-yearly compared with a 4-yearly screening interval was £3612 (£6586), increasing to £9601 (£34,112) for annual compared with 2-yearly screening. The probability the intervention is cost-saving is around 25% and it has around an 80% chance of being below the cost-effectiveness threshold of £30,000. For type 2 diabetes, the incremental cost per QALY of annual screening compared with a 2-yearly interval was £606 (£1782), making the intervention highly cost-effective. Screening at intervals less frequent than every 5 years has little impact on QALYs. The intervention appears to have a 100% probability of being below a cost-effectiveness threshold of £5000.
Discussion
The extent of reduction in renal albumin excretion with ACEi and A2RB treatment in patients with type 1 diabetes varies with presence or absence of microalbuminuria: treatment reduced albumin excretion rates by two-thirds in patients with prior microalbuminuria, but there was no evidence of benefit for patients without albumin in their urine. For type 2 diabetes, the relative benefit of treatment was approximately the same regardless of the presence or absence of microalbuminuria.
The ACR remains highly variable as a measure and, despite confirmation with two subsequent tests, has a high rate of false-positive diagnoses of microalbuminuria. In contrast, false-negative rates are low.
The eGFR has a limited role in monitoring renal progression in early diabetic kidney disease. In the current analysis, it adds no additional information in predicting the progression to macroalbuminuria for patients with type 1 and type 2 diabetes with chronic kidney disease stages ≥ 3 (eGFR > 60 ml/minute).
Annual screening with ACR appears to be cost-effective as an intervention to minimise the risk of adverse renal outcomes for both patients with type 1 diabetes and patients with type 2 diabetes, despite a high false-positive rate of screening leading to, at worst, unnecessary or, at best, early therapeutic intervention with ACEi and A2RBs. The source of variability when screening for albuminuria is the physiological variability of the disease marker in the individual. Improvements in test performance are unlikely and, therefore, new disease markers for the detection of risk of diabetic kidney disease are needed.
There are a number of limitations to this work, including the need for further validation of the type 1 and adapted type 2 health economic models, the exploratory nature of this work, which synthesises data from a wide range of sources, the lack of cardiovascular outcome data for the impact of ACEi and A2RB, and the limited range of options for screening considered. Our data for type 1 diabetes is drawn from a younger cohort; a clinical trial is currently under way that will provide more information about treatment of this group with A2RBs, even in the absence of elevated levels of albuminuria. In addition, options for regular monitoring need to consider the practicality of moving away from annual screening intervals and the importance of patients’ perceptions of screening intervals.
Conclusions
The simulated outcomes from both type 1 and type 2 models provide an evidence base to support current UK guidance for annual screening to identify the development of early kidney disease in both patients with type 1 diabetes and patients with type 2 diabetes.
Research priorities
Our type 1 health economic simulation model can be revised as further data become available from large studies of patients with type 1 diabetes to allow our conclusions to be further tested.
The type 1 simulation model will provide a tool with which other health economic questions about type 1 diabetes can be addressed.
Our estimates of cost-effectiveness may be improved by establishing cohorts of individuals at increased risk of developing microalbuminuria and diabetic kidney disease, with sampling at intervals more frequent than annually.
Further research is needed, and is already under way among children with type 1 diabetes, to establish whether individuals at risk of developing kidney disease might benefit from treatment before the occurrence of microalbuminuria. For example, those with an ACR persistently in the upper range of normal may represent a group at increased risk who may benefit from early treatment.
Alternative markers of kidney disease may be helpful to identify the risk of diabetic kidney disease. For example, cystatin C is an alternative to eGFR measurement for those who maintain normoalbuminuria, and might be included as a measure in cohort studies of individuals undergoing long-term monitoring of renal function.
Aspirin was previously recommended for individuals with diabetes, as the condition was viewed as a cardiovascular risk equivalent. Subgroup analyses from trials currently in progress may provide information that could change some of the assumptions around the benefits of screening included in our models.
Qualitative work may be required, along with subsequent surveys and, if appropriate, trials, to establish whether patient knowledge of renal albumin status provides an additional motivational factor sufficient to increase adherence to medication without causing an adverse impact on wellbeing.
Funding
Funding for this study was provided by the Health Technology Assessment programme of the National Institute for Health Research.
- Scientific summary - Optimal strategies for identifying kidney disease in diabet...Scientific summary - Optimal strategies for identifying kidney disease in diabetes: properties of screening tests, progression of renal dysfunction and impact of treatment – systematic review and modelling of progression and cost-effectiveness
- The START manual - START (STrAtegies for RelaTives) study: a pragmatic randomise...The START manual - START (STrAtegies for RelaTives) study: a pragmatic randomised controlled trial to determine the clinical effectiveness and cost-effectiveness of a manual-based coping strategy programme in promoting the mental health of carers of people with dementia
- Regulatory approvals - A high-dose preparation of lactobacilli and bifidobacteri...Regulatory approvals - A high-dose preparation of lactobacilli and bifidobacteria in the prevention of antibiotic-associated and Clostridium difficile diarrhoea in older people admitted to hospital: a multicentre, randomised, double-blind, placebo-controlled, parallel arm trial (PLACIDE)
- Acknowledgements - Placebo comparator group selection and use in surgical trials...Acknowledgements - Placebo comparator group selection and use in surgical trials: the ASPIRE project including expert workshop
- References - Clinical trial metadata: defining and extracting metadata on the de...References - Clinical trial metadata: defining and extracting metadata on the design, conduct, results and costs of 125 randomised clinical trials funded by the National Institute for Health Research Health Technology Assessment programme
Your browsing activity is empty.
Activity recording is turned off.
See more...