Included under terms of UK Non-commercial Government License.
NCBI Bookshelf. A service of the National Library of Medicine, National Institutes of Health.
Auguste P, Tsertsvadze A, Pink J, et al. Accurate diagnosis of latent tuberculosis in children, people who are immunocompromised or at risk from immunosuppression and recent arrivals from countries with a high incidence of tuberculosis: systematic review and economic evaluation. Southampton (UK): NIHR Journals Library; 2016 May. (Health Technology Assessment, No. 20.38.)
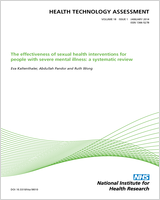
Accurate diagnosis of latent tuberculosis in children, people who are immunocompromised or at risk from immunosuppression and recent arrivals from countries with a high incidence of tuberculosis: systematic review and economic evaluation.
Show detailsObjective
The objective of the economic evaluation was to compare the cost-effectiveness of various screening strategies for the diagnosis of LTBI in immunocompetent children, people who are immunocompromised or at risk of immunosuppression and people who are recent arrivals from countries with a high incidence of active TB.
Currently in the UK, the following strategies are recommended to diagnose people with LTBI:10
- Children. Offer a Mantoux test to children aged 2–15 years. If positive, follow up with an IGRA.
- Immunocompromised. For people who are HIV negative, offer an IGRA alone or an IGRA with a concurrent Mantoux test. If either test is positive perform a clinical assessment to exclude active TB and treat.
- Recently arrived immigrants. Offer an IGRA alone or a dual strategy for people aged 16–35 years. If either test is positive, refer to a TB specialist to exclude active TB and treat.
- General population. Offer an IGRA alone or IGRA testing for people whose Mantoux test shows positive results.
Developing the model structure
To assess the cost-effectiveness of various strategies for the diagnosis of LTBI, we developed an economic model using R (version 3.1.1; The R Foundation for Statistical Computing, Vienna, Austria).
The model was developed with clinical input and represents, as far as possible, the clinical pathways that people would take while being screened for LTBI. The model structure for the child population is presented in Figure 49. The model was structured in two stages: diagnosis of LTBI and disease progression to active TB. The first stage of the model represents the clinical pathway that people would take in a 1-year time period before entering the infectious disease model. For this stage we used a decision tree structure for the diagnosis of LTBI. Four diagnostic strategies were examined in the model for each population:
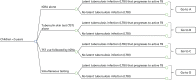
FIGURE 49
Decision tree structure for the child population.
- TST alone
- IGRA alone
- combinations of TST and IGRA
- simultaneous testing.
These strategies being compared were derived based on the strategies outlined in CG11710 and with input from the TB Guideline Development Group. The model begins with people receiving one of these diagnostic strategies (see Figure 49). The branches to the right of the decision node (square symbol) represent the strategies being compared (see Figures 50–54 for the child population and Appendix 15, Figures 60–74, for all other populations). People begin in one of the possible health states to the right of the chance nodes (circle symbols). The decision tree is modelled from individuals who have LTBI that progresses to active TB/no LTBI, followed by the probability of test results. However, in clinical practice the test result is known before LTBI is diagnosed. Modelling the test result first followed by disease category or vice versa makes no mathematical difference in terms of the expected values calculated for each diagnostic strategy.205 In the following sections we describe each strategy in detail.
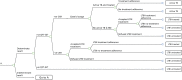
FIGURE 50
Pathway for the TST-alone diagnostic strategy in children. –ve, negative; +ve, positive; CXR, chest radiography.

FIGURE 54
Pathway for the simultaneous testing strategy in children. –ve, negative; +ve, positive; CXR, chest radiography.
Tuberculin skin test-alone strategy (Figure 50)
When screening with a TST, an individual may or may not return to have the test results interpreted (TST not read). Adults with positive TST results (induration ≥ 5 mm/10 mm) are assessed for initial active TB by chest radiography and sputum examination. Children with positive TST results are assessed for active TB by chest radiography and, if that is positive, a gastric lavage procedure. Those who have a positive result on chest radiography and sputum examination are treated for active TB. We assumed here that chest radiography and sputum examination are 100% accurate at diagnosing people who have initial active TB. People who adhere to TB treatment in the immunocompromised or recently arrived population may develop hepatitis and can survive or die from this adverse event. In the model with a cohort of children, we assumed that they would not develop hepatitis because it is a rare adverse event in this population.203 People who have a negative result on chest radiography and sputum examination (LTBI) can either accept or refuse to be treated for LTBI. Those who accept LTBI treatment may adhere/not adhere to treatment. If the TST is not read or the TST results are negative, the individual is not followed up.
Interferon gamma release assay-alone strategy (Figure 51)
When screening with an IGRA alone, an individual may have a determinate or an indeterminate result. Adults with a determinate result and who are IGRA positive are assessed for initial active TB by chest radiography and sputum examination. Children with positive IGRA results are assessed for active TB by chest radiography and, if that is positive, a gastric lavage procedure. Those who have a positive result on chest radiography and sputum examination are treated for active TB. Those who have a negative result on chest radiography and sputum examination (LTBI) can either accept or refuse to be treated for LTBI. People who accept LTBI treatment can adhere or not adhere to treatment. People with an indeterminate IGRA result receive a second IGRA test, which is the same as the initial IGRA test. If the IGRA result is negative or both IGRA tests are indeterminate, the individual is not followed up.

FIGURE 51
Pathway for the IGRA-alone diagnostic strategy in children. –ve, negative; +ve, positive; CXR, chest radiography.
Combined strategy (Figure 52)
For children and the recently arrived population, those who had their TST results interpreted, and whose results are positive, receive an IGRA test. Children with determinate, positive IGRA results receive chest radiography and, if positive, the gastric lavage procedure before a sputum examination for the assessment of active TB. Children with negative chest radiography/sputum examination results are either treated or not treated for LTBI. Children with indeterminate results receive a second IGRA test, which is the same as the initial IGRA test. If the TST is not read or the TST is negative, the individual is not followed up. Recent arrivals with determinate, positive IGRA results are assessed for active TB by chest radiography and sputum examination. If there is a positive result on chest radiography and sputum examination, they are treated for active TB. Those who have a negative result on chest radiography and sputum examination (LTBI) can either accept or refuse to be treated for LTBI. If people accept LTBI treatment, they may adhere/not adhere to treatment. People with an indeterminate IGRA result receive a second IGRA test, which is the same as the initial IGRA test. These people follow similar pathways to those who received one IGRA test. At most, people will receive two IGRA tests. If the TST result is not read, the TST result is negative, the IGRA result is negative or both IGRA tests are indeterminate, the individual is not followed up.

FIGURE 52
Pathway for the diagnostic strategy TST positive followed by IGRA in children. –ve, negative; +ve, positive; CXR, chest radiography.
Conversely, in the immunocompromised group, people receive an IGRA test first. Those who have a positive result on the IGRA test receive chest radiography and sputum examination to detect initial active TB. Those with a positive result on chest radiography and sputum examination are treated for active TB. Those who have a negative result can accept or refuse treatment for LTBI. People who have accepted and adhered to LTBI treatment may develop hepatitis and can survive or die from this adverse event.
Individuals with a negative IGRA result undergo a TST test. People here follow similar pathways as those who received the TST-alone strategy. Those with an indeterminate IGRA result receive a second IGRA test, which is the same as the initial IGRA test. These people follow similar pathways to those who received one IGRA test. At most, people will receive two IGRA tests. If the IGRA result is negative, both IGRA tests are indeterminate, the TST result is negative or the TST result has not been read, the individual is not followed up.
Simultaneous testing strategy (Figures 53 and 54)
When screening with an IGRA and a TST, people can have a combination of test results: a determinate result on the IGRA and the TST read, a determinate result on the IGRA and the TST not read, an indeterminate result on the IGRA and the TST read or an indeterminate result on the IGRA and the TST not read. Children with a positive result on either test receive chest radiography and, if positive, the gastric lavage procedure and sputum examination to detect initial active TB. For the other populations, those with a positive result on either test receive chest radiography and, if positive, a sputum examination to detect active TB. If the IGRA result is indeterminate and the TST is not read, the individual is not followed up.

FIGURE 53
Decision tree structure for the child population receiving the simultaneous testing strategy. –ve, negative; +ve, positive.
Stage 2 of the model is a disease progression model looking at progression between no TB/LTBI, LTBI that will progress to active TB and active TB, as well as secondary infections in other individuals caused by people with active TB. The basic model structure is shown in Figure 55. This structure is the same for people who are/are not being treated for LTBI/active TB, although the transmission probabilities are different in each of these cases. The outputs of the decision tree are used to determine the proportions of people who start in each state, specifically:
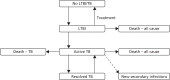
FIGURE 55
Dynamic transmission model.
- active TB
- LTBI – treated for LTBI
- LTBI – untreated
- no TB/LTBI – treated for LTBI
- no TB/LTBI – untreated.
The model used was a discrete event simulation, modelling individual patients, built using R (version 3.1.1). An initial simulation, starting with an identical cohort of 500,000 individuals in each arm, was run using the mean values of each parameter. To account for parameter uncertainty, we also ran a Monte Carlo simulation, consisting of 2000 different sampled parameter sets, each run on a starting sample of 100,000 individuals. An individual’s event risks at any time point are determined by their age, TB status and current treatment and remain constant until one of these factors changes.
People who begin the model with LTBI and who are not treated will develop active TB at a later point (from the definition of LTBI in our model as LTBI that progresses to active TB). The mean delay between the diagnostic test and progression to active TB was estimated from the systematic review, with individual activation times simulated assuming a constant activation rate over time. People who begin the model with LTBI and who are treated for LTBI have a certain probability of not developing active TB in the future (the effectiveness of the treatment – assumed to be 6 months of isoniazid), with activation times for those whose treatment is unsuccessful sampled as above.
Age-specific all-cause mortality rates were taken from UK-specific data in the Human Mortality Database206 and applied to all individuals in the model. Age-specific utilities for individuals without TB were calculated using data from the Health Survey for England.207 When an individual develops active TB, they have an immediate, age-specific probability of death, over that of all-cause mortality. Recovery rates from active TB were calculated from the mean length of an active TB episode, assuming a constant probability of recovery over time. Individuals with resolved TB have an annual probability of relapse, with subsequent activations having the same probability as the initial episode.
For each TB activation (primary or relapse), individuals generate a certain number of secondary cases of LTBI that will progress to active TB, sampled from a Poisson distribution. These cases are assumed to occur in the general population; hence, the age of the secondarily infected individuals was simulated from the average age distribution of active TB cases in the UK. These secondary cases were assumed to be identical (in terms of probability of death, average length of active TB episode, utility loss, number of secondary cases generated) to similarly aged individuals in the initial population. We did not simulate secondary cases of LTBI that do not progress to active TB as we have also not considered these in our initial population.
As the model is run, any new cases of LTBI generated are included in the disease progression model from that time forward. Costs and QALYs are accrued by individuals according to the length of time that they spend in each state of the model. Unlike a traditional economic model, it is not possible to continue running the simulation until all individuals have died, as there is a continuous stream of new individuals being added as a result of new infections. Consequently, the simulation was run for 100 years, with discounting meaning that any results over a longer time horizon than this are unlikely to make a meaningful difference to the outcome. The parameters for the discrete event simulation are presented in Table 27 for the child population and in Tables 64 and 65 (see Appendix 16) for the immunocompromised and recently arrived populations respectively.
TABLE 27
Model input parameters required for the child population
Model assumptions
A number of assumptions were required to develop a workable model structure to enable the analyses to be undertaken:
- We assumed that our population is similar to the population in the clinical effectiveness studies, but excluding studies with populations with a high incidence of active TB.
- People being assessed for initial active TB undergo chest radiography and, if positive, receive a sputum examination.
- Children being assessed for initial active TB undergo chest radiography and, if positive, undergo a gastric lavage procedure.
- The sputum examination is 100% accurate when diagnosing initial active TB.
- Individuals with a second indeterminate result on the IGRA test are at the same risk of developing active TB as those with a false-negative result.
- People who have been diagnosed with initial TB accept treatment.
- People who do not adhere to LTBI treatment take medication for 1 month.
- People who do not adhere to LTBI treatment are not at risk of developing isoniazid-induced hepatotoxicity.
- People who do not adhere to active TB treatment take medication for 1 month.
- Children are not at risk of developing hepatitis as a result of treatment for active TB or LTBI.
- No health loss is experienced by people with LTBI who do not progress to active TB.
Data required for the model
The model was populated with clinical information from the current clinical effectiveness review and supplemented with information from secondary sources. Information required to parameterise the model included prevalence, sensitivity and specificity, adverse events, resource use, and costs and utilities. We acknowledge here that there is no gold standard test for a LTBI diagnosis. Hence, we have used clinical information from studies in this review that reported information on confirmed cases of active TB (the proportion of untreated individuals who go on to develop active TB at a later date).
All of the data available for the child population were based on studies in which there was previous contact with an index case. We therefore restricted our analysis to this population both because of the lack of data and because it was thought unlikely that a general screening programme for all children, irrespective of contact, would ever be introduced.
Prevalence
In this analysis, prevalence was defined as the proportion of people who have LTBI that will progress to active TB, assuming that they are not treated. We derived estimates for this LTBI prevalence criterion based on empirical data from the three cohorts separately. We used WinBUGS software (version 1.4.3; MRC Biostatistics Unit, Cambridge, UK) to conduct Bayesian Markov chain Monte Carlo (MCMC) simulation to derive the prevalence of LTBI in each cohort using the following formula:
Rearranging the above equation for prevalence of LTBI:
To avoid overestimating the prevalence of LTBI that progresses to active TB, we excluded studies with populations with a high incidence (≥ 40 cases per 100,000) of active TB. For the recently arrived population, we derived the prevalence from all studies on recent arrivals found in the clinical effectiveness review, which included people with LTBI who progressed to active TB.
Performance of screening tests (sensitivity and specificity)
The sensitivities and specificities of the various strategies were derived based on information obtained from longitudinal studies in people who received testing and developed active TB. Therefore, our calculated sensitivities and specificities represent the sensitivities and specificities of detecting people with LTBI that will progress to active TB, not the sensitivities and specificities of detecting LTBI in general. Bayesian MCMC was used to derive posterior distributions for test performance assuming weakly informative priors to derive the sensitivity and specificity of the diagnostic tests by population. Estimates for sensitivity and specificity were derived for TST (≥ 5 mm), TST (≥ 10 mm), QFT-GIT and T-SPOT.TB.
To synthesise the clinical evidence in WinBUGS, there were three main components of the model: the statistical model, priors and data. Appendix 17 provides the WinBUGS code for the child population.
Statistical model
In our models we have used distributions to represent the unknown variables in the model. For the evidence synthesis for children, immunocompromised people and recent arrivals we used the binomial distribution to derive the sensitivities and specificities of TST, QFT-G, QFT-GIT and T-SPOT.TB. We chose the binomial distribution because we were interested in the probability p of the number of successes (people with positive/negative results that progressed to active TB) from n number of longitudinal studies.
First, we were interested in the probability ppos of the number of positive test results from n longitudinal studies and, second, we were interested in the probability papos of the number of positive results that progressed to active TB from n number of positive test results. Likewise, we were interested in the probability paneg of a negative result progressing to active TB.
Logical expressions were built into the model to represent the relationship between the probability of a positive result, the prevalence of LTBI, test sensitivity and test specificity (see Appendix 17).
We initially explored both fixed- and random-effects models. However, for two of the populations (children and immunocompromised people) the random-effects models did not converge (most likely because in a number of studies either no individuals or only a very small number of individuals progressed to active TB). Hence, for consistency, we used the fixed-effects model for the three populations.
Priors
We stated in the WinBUGS model the prior distribution to be used. We chose the uniform distribution because all possible combinations of positive and negative test results have an equal a priori probability of occurring. In our WinBUGS code we added a logic expression to inform the model that the sensitivity of TST (≥ 5 mm) is greater than the sensitivity of TST (≥ 10 mm), which is greater than the sensitivity of TST (≥ 15 mm). Likewise, the specificity of TST (< 5 mm) is lower than the specificity of TST (< 10 mm), which is lower than the specificity of TST (< 15 mm). We included this logic expression because the TST is a single test with various cut-off thresholds for a positive result and, by definition, TST (≥ 5 mm) would be more sensitive and less specific than TST (≥ 10/15 mm).
Data
Observed data from longitudinal studies identified in the clinical effectiveness review were entered into the model in a list format. Data included the number of people being tested, the number of people with positive results, the number of people with positive results who were untreated and who developed active TB and the number of people with negative results who developed active TB. Tables 67–72 (see Appendix 18) show the information obtained from the clinical effectiveness studies. The term ‘not applicable’ was used to represent any missing values. After compiling the model, we specified distributions from which to sample initial values for the model.
To obtain accurate posterior probabilities we used 60,000 simulations; a burn-in period of 30,000 simulations was used. Output from the remaining 30,000 simulations represented the posterior mean, along with its posterior standard deviation, posterior median and 95% credible intervals. Convergence of the model was assessed using a visual inspection of the sample trace plots (see Appendix 17).
The results of the meta-analysis are presented in Table 28. The sensitivity and specificity of TST (≥ 5 mm) for the diagnosis of LTBI in children were estimated at 72.80% and 49.03%, respectively. In the immunocompromised group we derived estimates of 32.42% and 74.22% for the sensitivity and specificity of TST (≥ 5 mm), respectively. In the recently arrived immigrants group we derived estimates of 93.56% and 50.11% for the sensitivity and specificity of TST (≥ 5 mm), respectively. In the models we have not stratified by BCG status and hence we used a cut-off of ≥ 5 mm to define a positive TST.
TABLE 28
Diagnostic accuracy of various tests for diagnosing LTBI that progresses to active TB
Similar methods were used to derive the sensitivity and specificity of TST (≥ 10 mm) in these populations. The sensitivity and specificity of QFT-GIT for the diagnosis of LTBI in children were estimated at 68.84% and 61.03%, respectively. In the immunocompromised group we derived estimates of 55.48% and 82.27% for sensitivity and specificity, respectively, and in the recently arrived group we derived estimates of 59.15% and 79.29% for sensitivity and specificity, respectively. In the models we used QFT-GIT values as the base-case values for the analysis because the majority of the studies compared QFT-GIT with the TST.
Resource use and costs
The resource use and costs included were those directly incurred by the NHS. Costs for diagnostic tests, chest radiography, gastric lavage, sputum examination, treatment of LTBI/TB and isoniazid-induced hepatitis were all included in the analysis. Societal costs (indirect costs, loss of productivity or cost of death) were not included. The unit costs are presented in Table 27. The majority of the cost information used in the analyses was obtained from secondary sources. The costs for QFT-GIT (testing kit, consumables, processing and phlebotomy) and the TST (disposables, administration and reading) were obtained from Pooran et al.209 Estimated costs for chest radiography, the gastric lavage procedure and sputum examination were obtained from NHS reference costs 2012/13.214 Estimated costs for the treatment of LTBI were obtained from the NHS drug tariff 2015216 and in consultation with a clinical expert (see Appendix 16). Costs for the treatment of TB were obtained from Bothamley et al.215 (see Appendix 16). Management of LTBI included further blood tests (full blood count and liver function tests), doctor and nurse outpatient visits, and treatment with 300 mg of isoniazid daily for 6 months. Estimated costs for treating isoniazid-induced hepatitis were obtained from Pareek et al.77 All costs were adjusted to 2012/13 prices using the Hospital and Community Health Services pay and price index217 and discounted at a rate of 3.5% per annum, as recommended by NICE.91
Outcomes
Two different outcome measures were used in the analysis, QALYs and diagnostic error avoided. To calculate QALYs, age-related utility weights for the general population were obtained from the Health Survey for England207 and the utility decrement of 0.15 for people who received treatment for active TB was derived from the published literature.197 With respect to the diagnostic error avoided, we did not require any effectiveness information; the true-positive and true-negative cases were given the value of 1 and we reserved the value of 0 for an error (false positives and false negatives) in the diagnosis.
Analysis
The models were constructed to assess the cost-effectiveness of various strategies for the diagnosis of LTBI in three populations (children, immunocompromised people and recently arrived immigrants). The models estimated the mean costs and effects associated with each diagnostic strategy. For children, we began with a hypothetical cohort of children aged 5 years, whereas for the recently arrived and immunocompromised populations the starting distributions were representative of the UK recent arrival and UK general populations respectively.218 The analysis was undertaken from a NHS perspective in a primary care setting and outcomes were reported as ICERs, expressed in terms of cost per diagnostic error avoided and cost per QALY gained. Because using QALYs allows trade-offs between the harms of false negatives and the harms of false positives, which are treated as equal in a cost per error avoided analysis, our primary conclusions are drawn from the ICERs expressed as cost per QALY gained. Univariate sensitivity analyses and PSAs were undertaken to assess the impact of the uncertainty of model input parameters.
Probabilistic sensitivity analysis
A PSA was undertaken to determine the joint uncertainty in the key model input parameters of prevalence, sensitivity and specificity, and expected QALYs. We undertook the PSA based on an outcome of cost per QALY only. In the PSA, each model parameter is assigned a distribution, reflecting the amount and pattern of its variation, and cost-effectiveness results are calculated by simultaneously selecting random values from each distribution. In total, 2000 sets of parameters were simulated, each of which was run on a starting cohort of 100,000 individuals. Because of the considerable heterogeneity of the studies included in our meta-analysis, the results from the PSA, which explicitly includes the impact of that uncertainty, were considered to provide more plausible estimates of costs and outcomes than our single simulation based on mean parameter values. Therefore, costs and outcomes used to produce ICERs were calculated as the means of the costs and outcomes in each of the 2000 PSA simulations. The distributions used in the PSA are presented in Table 27. We also calculated the probability that each strategy is the most cost-effective at a willingness to pay of £20,000 per QALY.
Results of the cost-effectiveness modelling
The results of the cost-effectiveness modelling of various strategies for the diagnosis of LTBI in the three populations, based on the outcomes of cost per diagnostic error avoided and cost per QALY gained, are presented in the following sections.
Model 1: children
The results from the 250,000 patient simulations, based on the mean value of each parameter, are presented in Tables 29 and 30. Table 29 shows the mean per patient cost (including both the initial cohort and subsequent secondary cases) for each of the six strategies as well as a breakdown of the total cost into diagnosis, LTBI treatment, active TB and hepatitis costs. Table 30 shows the incidence rates of active TB in the initial cohort, the numbers of secondary infections, mean life-years and mean QALYs for each of the strategies.
TABLE 29
Mean costs and cost breakdown based on a single simulation using mean parameter values (2012/13 prices)
TABLE 30
Mean QALYs and life-years gained (discounted), incidence of active TB and numbers of secondary infections
The primary results, based on the 2000 Monte Carlo simulations, are presented in Tables 31 (diagnostic accuracy) and 32 (QALYs). Considering diagnostic accuracy, the TST (≥ 10 mm)-alone strategy dominated the TST (≥ 5 mm) negative followed by QFT-GIT, TST (≥ 5 mm), QFT-GIT and TST (≥ 5 mm) positive followed by QFT-GIT strategies. The TST (≥ 10 mm) strategy had a mean cost of approximately £272 with a corresponding diagnostic error of 0.2449 compared with a mean cost of approximately £306 and a diagnostic error of 0.2322 for the T-SPOT.TB-alone strategy. The ICER for T-SPOT.TB compared with TST (≥ 10 mm) indicates the additional cost required to avoid one diagnostic error. The results for the simultaneous testing strategy and the TST (≥ 10 mm) followed by QFT-GIT are not presented because these results were dominated by the sequential testing strategy and TST (≥ 5 mm) followed by QFT-GIT, respectively.
TABLE 31
Results from the analysis based on cost per diagnostic error avoided (2012/13 prices)
TABLE 32
Results from the analysis based on cost per QALY (2012/13 prices)
The QALY outcomes of the Monte Carlo simulations showed that the TST (≥ 10 mm) diagnostic strategy alone was the least costly strategy and the TST (≥ 5 mm) negative followed by QFT-GIT was the most effective strategy for the diagnosis of LTBI in this population. The QFT-GIT-alone diagnostic strategy had a mean cost of £361 with corresponding QALYs of 23.095, whereas the TST (≥ 5 mm)-alone strategy had a mean cost of £371 and 23.096 QALYs. The ICER of £11,255 indicates the additional cost required to gain an extra QALY. In terms of the joint uncertainty in the expected mean costs and QALYs, the results show that TST (≥ 5 mm) negative followed by QFT-GIT is the most cost-effective strategy at a willingness to pay of £20,000 per QALY in 32% of the simulations followed by TST (≥ 5 mm) (27%) and QFT-GIT (21%).
The results of the univariate sensitivity analyses are presented in Table 33. In each scenario we present costs and QALYs for each of the three most effective strategies [QFT-GIT, TST (≥ 5 mm) and TST (≥ 5 mm) negative followed by QFT-GIT]. We also show which of the three strategies was the most cost-effective in each scenario, assuming a willingness to pay of £20,000 per QALY. In the majority of scenarios, as in the base case, the TST (≥ 5 mm) negative followed by QFT-GIT strategy was the most cost-effective strategy. However, decreases in prevalence, the sensitivity of the TST, the effectiveness of LTBI treatment or the disutility associated with active TB, as well as increases in the sensitivity of QFT-GIT, all led to QFT-GIT being the most cost-effective option. Conversely, decreases in the sensitivity of QFT-GIT led to the TST (≥ 5 mm) being selected as the most cost-effective option.
TABLE 33
Univariate sensitivity analyses
Finally, Figure 56 presents CEACs for each of the same three strategies, showing the proportion of simulations in which each has the highest net benefit at different willingness-to-pay thresholds.

FIGURE 56
Cost-effectiveness acceptability curves for the child population, showing the proportion of simulations in which each strategy is the most cost-effective at different willingness-to-pay thresholds.
Model 2: immunocompromised people
The results from our 250,000 patient simulations, based on the mean value of each parameter, are presented in Tables 34 and 35. Table 34 shows the mean per patient cost (including both the initial cohort and subsequent secondary cases) for each of the six strategies as well as a breakdown of the total cost into diagnosis, LTBI treatment, active TB and hepatitis costs. Table 35 shows the incidence rates of active TB in the initial cohort, the numbers of secondary infections, mean life-years and mean QALYs for each of the strategies.
TABLE 34
Mean costs and cost breakdown based on a single simulation using mean parameter values (2012/13 prices)
TABLE 35
Mean QALYs and life-years gained (discounted), incidence of active TB and numbers of secondary infections
The primary results, based on the 2000 Monte Carlo simulations, are presented in Tables 36 (diagnostic accuracy) and 37 (QALYs). Considering diagnostic accuracy, QFT-GIT dominated the QFT-GIT negative followed by TST (≥ 5 mm), T-SPOT.TB and TST (≥ 5 mm) strategies. The TST (≥ 10 mm) strategy had a mean cost of approximately £236 with a corresponding diagnostic error of 0.1641 whereas the QFT-GIT positive followed by TST (≥ 5 mm) strategy had a mean cost of approximately £253 and a diagnostic error of 0.1047. The ICER of £297 per diagnostic error avoided for the QFT-GIT positive followed by TST (≥ 5 mm) strategy compared with the TST (≥ 10 mm) strategy shows the additional cost required to avoid a diagnostic error. We have not presented the results for the simultaneous testing strategies because these strategies were dominated by the equivalent sequential strategies.
TABLE 36
Results from the analysis based on cost per diagnostic error avoided (2012/13 prices)
TABLE 37
Results from the analysis based on cost per QALY (2012/13 prices)
The QALY outcomes of our Monte Carlo simulations showed that TST (≥ 10 mm), QFT-GIT positive followed by TST (≥ 5 mm) and TST (≥ 5 mm) were dominated by the QFT-GIT-alone strategy, which had a mean cost of £259 with corresponding QALYs of 15.523. The ICER reported for the T-SPOT.TB-alone strategy shows the additional cost required to gain 1 extra QALY compared with the QFT-GIT strategy. At a willingness to pay of £20,000 per QALY, the QFT-GIT negative followed by TST (≥ 5 mm) had the highest net benefit in the largest proportion of simulations (40%), followed by the T-SPOT.TB (25%) and QFT-GIT-alone (19%) strategies. All other strategies had the largest net benefit in < 7% of the simulations.
The results of the univariate sensitivity analyses are presented in Table 38. In each scenario we present costs and QALYs for each of the three strategies that were not strictly dominated by another strategy in the primary results. We also show which of the three strategies was the most cost-effective in each scenario, assuming a willingness to pay of £20,000 per QALY. In the scenarios in which the importance of test sensitivity was equal to or higher than that in the base case, the QFT-GIT negative followed by TST (≥ 5 mm) strategy was consistently the most cost-effective strategy at a willingness to pay of £20,000 per QALY. In the scenarios in which the relative importance of test specificity was increased (by decreasing LTBI prevalence, decreasing the effectiveness of LTBI treatment, increasing the cost of LTBI treatment, decreasing the cost of active TB or ignoring the impact of secondary TB cases), QFT-GIT often became the most cost-effective strategy.
TABLE 38
Univariate sensitivity analyses
Finally, Figure 57 presents CEACs for each of the three non-dominated treatment strategies, showing the proportion of simulations in which each has the highest net benefit at different willingness-to-pay thresholds.
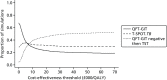
FIGURE 57
Cost-effectiveness acceptability curves for the immunocompromised population, showing the proportion of simulations in which each strategy is the most cost-effective at different willingness-to-pay thresholds.
Model 3: recent arrivals from countries with a high incidence of tuberculosis
The results from our 250,000 patient simulations, based on the mean value of each parameter, are presented in Tables 39 and 40. Table 39 shows the mean per patient cost (including both the initial cohort and subsequent secondary cases) for each of the six strategies as well as a breakdown of the total cost into diagnosis, LTBI treatment, active TB and hepatitis costs. Table 40 shows the incidence rates of active TB in the initial cohort, the numbers of secondary infections, mean life-years and mean QALYs for each of the strategies.
TABLE 39
Mean costs and cost breakdown based on a single simulation using mean parameter values (2012/13 prices)
TABLE 40
Mean QALYs and life-years gained (discounted), incidence of active TB and number of secondary infections
The primary results, based on the 2000 Monte Carlo simulations, are presented in Tables 41 (diagnostic accuracy) and 42 (QALYs). Considering diagnostic accuracy, the QFT-GIT-alone strategy was the least costly strategy and the TST (≥ 5 mm) positive followed by QFT-GIT strategy was the most effective. The QFT-GIT strategy had a mean cost of approximately £266 with a corresponding diagnostic error of 0.2113, whereas the TST (≥ 5 mm) positive followed by QFT-GIT strategy had a mean cost of approximately £277 and a diagnostic error of 0.1955. The ICER for the TST (≥ 5 mm) positive followed by QFT-GIT strategy compared with the QFT-GIT-alone strategy shows an additional cost of £692 to avoid one diagnostic error. We have not presented the results for the simultaneous testing strategies because these strategies were dominated by the equivalent sequential strategies.
TABLE 41
Results from the analysis based on cost per diagnostic error avoided (2012/13 prices)
TABLE 42
Results from the analysis based on cost per QALY (2012/13 prices)
The QALY outcomes of our Monte Carlo simulations showed that the QFT-GIT strategy dominated the TST (≥ 5 mm) positive followed by QFT-GIT and T-SPOT.TB strategies. TST (≥ 5 mm) had a mean cost of £299 with corresponding QALYs of 19.922. TST (≥ 5 mm) negative followed by QFT-GIT was more expensive than the TST (≥ 5 mm) strategy, with corresponding QALYs of 19.923 and an ICER of £58,720 compared with TST (≥ 5 mm). At a willingness to pay of £20,000 per QALY, the TST (≥ 5 mm) strategy had the highest net benefit in the largest proportion of simulation (47%) followed by the TST (≥ 5 mm) negative then QFT-GIT strategy (28%) and the QFT-GIT-alone strategy (18%). All other strategies had the largest net benefit in < 5% of the simulations.
The results of the univariate sensitivity analyses are presented in Table 43. In each scenario we present costs and QALYs for the three strategies that were not strictly dominated by another strategy in the primary results. We also show which of the three strategies was the most cost-effective in each scenario, assuming a willingness to pay of £20,000 per QALY. In the majority of scenarios, as in our base case, the TST (≥ 5 mm)-alone strategy was the most cost-effective strategy. However, a decrease in the prevalence of LTBI, increase in the sensitivity of QFT-GIT and decrease in the sensitivity of the TST all led to strategies involving QFT-GIT becoming the most cost-effective.
TABLE 43
Univariate sensitivity analyses
Finally, Figure 58 presents CEACs for each of the three non-dominated treatment strategies, showing the proportion of simulations in which each has the highest net benefit at different willingness-to-pay thresholds.
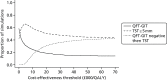
FIGURE 58
Cost-effectiveness acceptability curves for the recently arrived population, showing the proportion of simulations in which each strategy is the most cost-effective at different willingness-to-pay thresholds.
Exploring sensitivity and specificity
Clearly, key drivers of differences between the models are sensitivity and specificity. To illustrate the impact that these parameters have on the outputs of our models, Figure 59 shows graphs of sensitivity and specificity plotted against costs, QALYs and net monetary benefit (at £20,000 per QALY) for each of the six strategies that were simulated in the child population.
These graphs show, at first sight, the counterintuitive result that increased specificity is associated with lower QALYs and lower net monetary benefit whereas higher sensitivity is associated with higher costs. This is because of the high levels of correlation between sensitivity and specificity (specifically, higher sensitivity is associated with lower specificity) in the strategies that were simulated. Therefore, both sets of graphs are in fact showing the same result, namely that, as sensitivity increases and specificity decreases, this leads to higher QALYs, higher costs and, on balance, a higher net monetary benefit.
To try and remove the effect of this sensitivity/specificity correlation, instead of using the different strategies we used the outputs of the PSA simulations for one of these strategies. This gave us 2000 realisations of sensitivity, specificity, costs and QALYs and, as each of these sensitivity/specificity pairs was a sample from the posterior distribution of our MCMC, we would expect lower correlations between sensitivity and specificity than when comparing between different strategies. We then ran a linear regression model for costs and QALYs, with sensitivity and specificity as the predictor variables. The results of this regression model are shown in Table 44.
TABLE 44
Results of the linear regression model
In this model, in which we jointly estimated the impact of both sensitivity and specificity on outcomes, the results are much more intuitive. Increases in both sensitivity and specificity lead to increases in QALYs and decreases in costs, with increases in sensitivity providing the largest QALY gains and increases in specificity the largest cost reductions. It should be noted that the output data from the PSA simulation very likely do not conform to the necessary assumptions (linearity, additivity, etc.) for linear regression and the models contain a lot of noise because of the impact of varying other parameters and so the actual values of these parameters should be treated with extreme caution. Nevertheless, they do give an indicative picture of what the key drivers of difference between the models are.
Discussion and conclusion
The results based on the outcome of cost per diagnostic error avoided showed that, in the child population, the TST (≥ 10 mm) strategy dominated all strategies except for the T-SPOT.TB strategy alone. T-SPOT.TB compared with TST (≥ 10 mm) was more effective but more expensive, with an ICER of approximately £2711 per diagnostic error avoided. A breakdown of effectiveness showed that the T-SPOT.TB strategy resulted in fewer false-positive cases (0.2172) than the TST (≥ 10 mm) strategy (0.2307), but a larger number of false-negative cases (0.0150 vs. 0.0142). If the T-SPOT.TB strategy were to be used in this population to diagnose LTBI that progresses to active TB, this would lead to a slight reduction in the number of children being overtreated for LTBI. In the immunocompromised population, QFT-GIT dominated QFT-GIT negative followed by TST, T-SPOT.TB and TST (≥ 5 mm) in terms of diagnostic errors avoided. The results showed that QFT-GIT resulted in fewer false positives and fewer false negatives than the other strategies. The use of TST (≥ 10 mm) in this population was more effective, with overall diagnostic errors avoided of 0.1641. A breakdown of this effectiveness showed that TST (≥ 10 mm) resulted in fewer false-positive but more false-negative results. Likewise, the combination strategy QFT-GIT positive followed by TST (≥ 5 mm) produced fewer false-positive results but more false-negative results. In the population of recent arrivals from countries with a high incidence of TB, QFT-GIT dominated the T-SPOT.TB, TST (≥ 5 mm) negative followed by QFT-GIT and TST (≥ 5 mm) strategies. TST (≥ 5 mm) positive followed by QFT-GIT had an ICER of £692 per diagnostic error avoided compared with QFT-GIT, with more false negatives and fewer false positives.
The cost per QALY outcomes are summarised in terms of the probability of each strategy being the most cost-effective (at a given threshold). We used a willingness-to-pay threshold of £20,000 per QALY, a standard threshold that is used in the UK. The results in the child population showed that TST (≥ 5 mm) is marginally more effective than the QFT-GIT-alone strategy, with an ICER of approximately £11,255 per QALY, and has a 27% probability of being the most cost-effective strategy at a willingness to pay of £20,000 per QALY. The most effective strategy was TST (≥ 5 mm) negative followed by QFT-GIT, which was the most cost-effective strategy in 32% of the simulations. The results in the immunocompromised population showed that QFT-GIT negative followed by TST (≥ 5 mm) was the most effective strategy, with an ICER of approximately £18,746 compared with T-SPOT.TB, and is the most cost-effective strategy in 40% of the simulations. In the population of recent arrivals, TST (≥ 5 mm) dominated the TST (≥ 5 mm) positive followed by QFT-GIT, T-SPOT.TB and QFT-GIT strategies, and had a probability of 47% of being cost-effective at a willingness to pay of £20,000 per QALY.
Based on the current clinical evidence on people with LTBI without treatment that progressed to active TB as well as expert opinion used to develop the model structures, the results demonstrate that TST (≥ 5 mm) was slightly more cost-effective than QFT-GIT in the child population. In the immunocompromised population the results based on cost per QALY showed that QFT-GIT negative followed by TST (≥ 5 mm) was the most cost-effective strategy. In the recent arrivals population the results based on cost per QALY showed that TST (≥ 5 mm) dominated the TST (≥ 5 mm) positive followed by QFT-GIT, T-SPOT.TB and QFT-GIT-alone strategies.
- Health economics methods and results - Accurate diagnosis of latent tuberculosis...Health economics methods and results - Accurate diagnosis of latent tuberculosis in children, people who are immunocompromised or at risk from immunosuppression and recent arrivals from countries with a high incidence of tuberculosis: systematic review and economic evaluation
- List of abbreviations - Folate Augmentation of Treatment – Evaluation for Depres...List of abbreviations - Folate Augmentation of Treatment – Evaluation for Depression (FolATED): randomised trial and economic evaluation
- Drosophila arizonae strain AZTUS-31 antares (antr) gene, complete cdsDrosophila arizonae strain AZTUS-31 antares (antr) gene, complete cdsgi|744515636|gb|KP236491.1|Nucleotide
- MP19 [Homo sapiens]MP19 [Homo sapiens]gi|13445660|gb|AAK26328.1|AF340020_Protein
- Sexual risk reduction interventions for patients attending sexual health clinics...Sexual risk reduction interventions for patients attending sexual health clinics: a mixed-methods feasibility study
Your browsing activity is empty.
Activity recording is turned off.
See more...