NCBI Bookshelf. A service of the National Library of Medicine, National Institutes of Health.
King C, Llewellyn C, Shahmanesh M, et al. Sexual risk reduction interventions for patients attending sexual health clinics: a mixed-methods feasibility study. Southampton (UK): NIHR Journals Library; 2019 Mar. (Health Technology Assessment, No. 23.12.)

Sexual risk reduction interventions for patients attending sexual health clinics: a mixed-methods feasibility study.
Show detailsBackground
The use of data-driven triage tools, developed using predictive statistical models, is relatively common in both primary and secondary clinical care.89 They are used to target individual care based on key risk characteristics found at the population level, such as the Framingham risk score, which has been widely used to support treatment decisions for cardiovascular disease.90 In SH, triage is commonplace.91,92 Clinics often stratify patients according to symptoms, behavioural risks and demographics to receive different services such as ‘quick checks’ or safeguarding.93,94 These triage processes tend to be a dichotomous decision based on predefined criteria, which may not necessarily take into account risk behaviours or identify patients most in need of interventions.95
Since 2009, SH clinics in England have provided data to a mandated surveillance system for SH episodes, the Genitourinary Medicine Clinic Activity Data Set (GUMCAD).96,97 This data set contains 12 variables that include demographics and any tests and diagnoses related to that episode of care. This has allowed spatial trends in STIs to be monitored over time; however, it lacks information on risk behaviours, which would allow for more detailed risk stratification.
In order to facilitate a more in-depth understanding of STI epidemiology in England, Public Health England (PHE) enhanced the GUMCAD to include numbers of partners, drug and alcohol use, prior GUM clinic visits and partner notifications in GUMCADv3.98,99 The British Association for Sexual Health and HIV (BASHH) recommends that all these variables are recorded as part of a patient consultation, and they are therefore intended to be feasible for collection in routine care.100 The GUMCADv3 reporting system was piloted in two phases, with revisions made in phase 2 based on clinic feedback and data quality issues from phase 1.
A population-level, data-driven approach to triage, based on the risk of a STI diagnosis, has not yet been applied to the UK setting. In order to test a model of delivery of a behavioural intervention that is tailored to the risk profile and characteristics of the target population, we therefore developed a data-driven triage tool that could be integrated into service systems and processes.
Aim
The aim was to develop a triage tool based on clinical data routinely collected within SH clinics in England, in order to stratify patients according to their risk of STI diagnosis and thereby direct service users to tailored behavioural interventions individualised to their needs. Separate models were to be developed for the MSM and young people groups because of the different risk types and relative importance of behavioural and demographic data.
Method
We conducted secondary data analysis of the nationally mandated GUMCADv2 data from 2013 to 2015 and the second phase of the GUMCADv3 pilot, conducted in 2015–16. Analysis of the phase 1 GUMCADv3 pilot is not presented as this version of the surveillance system was superseded by the phase 2 version.
Data sets
Surveillance (Genitourinary Medicine Clinic Activity Data Set v2)
This is a national mandatory reporting data set for England: all level 2 and level 3 SH services are required to submit their SH patient episodes to PHE. It covers an estimated 600 services (including SH services) and reports STI diagnoses. The data set contains 12 variables (Table 4): demographics, attendance information and any episode activity and diagnoses. Data from the reporting periods quarter 1 (2013) to quarter 3 (2016) were used. This data set is referred to as ‘v2’ throughout.
TABLE 4
Genitourinary medicine clinic activity data set variables available for triage tool analysis
Enhanced surveillance (Genitourinary Medicine Clinic Activity Data Set v3 pilot 2)
This data set was generated by PHE during a pilot conducted from July 2015 to June 2016 in five SH clinics: Bedford (Brook), Bristol (GUM), Croydon (GUM), Barnet (GUM) and Southend (GUM). This data set contains the same 12 variables from v2 and an additional 18 questions on recent sexual behaviours, drug and alcohol use, and previous diagnoses and attendance (see Table 4). This data set is referred to as ‘v3p2’ throughout.
Definitions
Young person: any attendance among all women, and men who have no report of sex with men and self-report as heterosexual, aged 16–25 years.
Men who have sex with men: any attendance among men who have any report of sex with men, or self-report as bisexual or homosexual, of any age.
Attendance: any first attendance within an episode of care.
Outcome: any new diagnosis of HIV, syphilis, gonorrhoea, chlamydia, hepatitis, lymphogranuloma venereum, trichomonas or herpes. Recurrent herpes and warts infections and non-specific genital infections were excluded.
Data management
The v2 data undergo routine data cleaning processes by PHE; details of this process are available on request from PHE (‘GUMCADv2 Specifications Manual_v3_23_09_2014’) (Hamish Mohammed, Public Health England, 23 September 2014). The v3 data were cleaned for inconsistencies between demographic and reported sexual behaviours (e.g. a female heterosexual reported as having female sex partners), drugs reports (e.g. no reported drug use and sharing injecting equipment) and previous SH attendances and diagnoses. During the cleaning, a positive response to any question about high-risk behaviour was given more weight than any contradictory negative response; for example, if an individual reported ‘no’ to drug use in the previous 3 months but gave a positive response to subsequent questions about the use of cannabis, then the answer to ‘any drug use’ would be changed to ‘yes’ and the answer to questions about cannabis use would be left unchanged. In the case of discrepancies between gender, sexual orientation and types of partners, gender and partner type were prioritised. For example, a heterosexual male reporting male partners would be classified as MSM within the model. Cases with multiple items of conflicting data were excluded.
The core v2 variables were still reported through the routine v2 system for the pilot clinics; the v3 pilot data were submitted separately to PHE. The clinic code, patient identification (ID) and attendance date were used to merge the two data sets. Checks for discrepancies in demographic information between v2 and v3 data sets were conducted and resolved on a case-by-case basis; cases with inconclusive cleaning were excluded from analysis. If patients from the v3p2 data set were merged with a v2 record, demographic variables were compared to test for possible biases in the subset of patients available for analysis. All cleaning, merging and data management was carried out using Stata® (StataCorp LP College Station, TX, USA) version 13.
Selection of candidate predictors
The predictor variables investigated were those available in the data set. The behavioural and risk variables included in the v3p2 data set were based on those recommended for sexual history taken by BASHH in 2013 and are well supported in the literature as being indicators of STI risk.100 The variables were split into demographic and behavioural variables. Demographic variables included age, deprivation, prior GUM visits, prior STI diagnosis (including specific infections), ethnicity, country of birth, sexual orientation, gender and HIV status. Behavioural variables included number of sexual partners, new partners, condom use, problematic alcohol use and drug use, and unprotected anal intercourse and sex with known HIV-positive partners in MSM. Depending on the number of observations and degrees of freedom in the models, variables were recategorised between models.
All of these variables were considered in the model development; however, exclusion for reasons of missing data or low prevalence (e.g. < 5%) was undertaken following the initial description. Variables with missing data may introduce bias if the data are not missing at random (e.g. if patients are less likely to disclose risky behaviours, or there are differences in reporting quality between clinics), and if they are not frequently available then including them in a triage tool might be impracticable.101 There are several approaches to dealing with missing data. For variables with limited missing data (< 25%), which are assumed to be missing at random, multiple imputation is recommended, as it preserves sample size.102 However, including missing data as a distinct category may be a more pragmatic approach, as complete data collection within a routine clinical setting may not be realistic, and missing data are unlikely to be missing completely at random. This was our primary analysis approach.
To protect against overfitting, a general rule is to have 10 outcome events (i.e. STI diagnoses) per degree of freedom in the development model (i.e. predictor variable).103 Lower priority or highly correlated candidate predictors were removed to reduce the number of degrees of freedom when possible and necessary.103
Developing the prediction model
The primary outcome was the binary composite variable of STI diagnosis. Multivariable logistic regression was used to develop the triage tool. The primary models were developed in the v3p2 data set, one for MSM and one for young people.
We used a full model approach, with all predefined variables included regardless of statistical association in univariate analysis.101,104 We conducted a sensitivity analysis using a forward stepwise approach to explore whether or not a more parsimonious model could be used. All variables were binary or categorical, except age and deprivation score (derived from the patient’s postcode). Continuous variables were investigated for non-linear relationships with the outcome and categorised if appropriate. Data reduction within the categorical variables (e.g. ethnicity) was undertaken based on data patterns and substantive knowledge.
The regression coefficients were used to calculate an individual’s probability of STI diagnosis using the following equations (Box 3 presents a worked example):
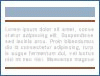
BOX 3
Worked example of the triage tool
Model performance
Model performance was evaluated using several statistical tests. The Hosmer–Lemeshow goodness of fit test was carried out to measure model calibration,105 despite its limitations.106 Model discrimination was tested using the c-statistic [area under the receiver operating characteristic curve (AUROC)].101,107 The c-statistic and the pseudo-R2 were the main parameters for determining if the model was effective at predicting the outcome of interest. A c-statistic of > 0.7 is generally considered reasonable model discrimination for a clinical tool, and one of > 0.8 is considered strong discrimination; 0.5 indicates that the model is no better than chance at predicting the outcome.108 The Bayesian information criterion (BIC) was used to determine the most parsimonious model in sensitivity analyses, with lower values favouring model selection.
We compared different probability thresholds with the patient’s true outcome to give sensitivity, specificity, positive predictive values (PPVs) and negative predictive values (NPVs). External validation, when the regression equation is tested in a district data set, is recommended as an independent assessment of the model performance to assess the extent of overfitting and the resulting optimism of its performance.109 External validation was not conducted because of the limited sample size of the v3p2 pilot; however, it was discussed that external validation could be done as part of the WP5 (see Chapter 6) pilot implementation.
Sensitivity analyses
We conducted sensitivity analyses in order to test assumptions about our primary modelling approach. We assessed a model that included only demographic data to determine how much added value the additional behavioural information provides; this also allowed us to investigate whether or not demographics at the national level had different relationship directions and magnitudes of effect to the smaller v3p2 data set. Missing data, which were included as a distinct category in the primary model, were compared with imputed models to give us more information on pragmatic implementation. A categorised missing approach was adopted to reflect the real-world nature of routine data, and because we made the assumption that data were not missing at random and, therefore, may contain predictive value in themselves. Finally, a full model where all a priori defined variables were included was compared with a forward stepwise regression approach.
Results
Data description
During the pilot period from July 2015 to June 2016, a total of 28,514 episodes of care were reported. Table 5 describes the key demographic variables between those with and those without enhanced behavioural data. The patients recorded in the v3p2 data set were similar in terms of ethnicity, age and gender to those with only basic surveillance for the same time period. There were considerably higher levels of missing sexual orientation information in the enhanced data set (16% vs. 7%), and lower numbers of homosexual or bisexual patients (6% vs. 13%). This probably reflects the fact that the pilot sites do not include any of the clinics with higher proportions of MSM clients, such as Dean Street or Brighton.
TABLE 5
Description of demographic variables in the GUMCAD surveillance and enhanced surveillance data sets
Following cleaning of the merged data set, there were 9530 non-MSM young people recorded in the v3p2 pilot, of whom 1005 had a STI diagnosis (10.6%). This is very similar to the STI diagnosis rate seen in the national surveillance data set during the same time period (10.8%). There were 1448 MSM records in the v3p2 data set, with 318 STI diagnoses (22.0%). This was higher than the nationally reported rate of 14.9%. This allows up to 100 and 32 degrees of freedom in the young person and MSM models, respectively, to avoid overfitting.
Young people and MSM differed from the general surveillance population and from each other (Table 6). The proportion of young women in the data set was higher than in the general clinic population (69% vs. 59%) and the MSM population in the data set was older than in the overall clinic population and more likely to be of white ethnicity (82% vs. 70%). The number of partners reported by young people generally reflected the general population, but MSM reported a higher proportion of multiple partners, with 15% reporting five or more partners in the previous 3 months compared with 3% of the general pilot clinic population. They also had a lower number of missing data for this variable. MSM reported double the rate of drug use of young people (14% vs. 7%) and considerably lower rates of missing data for this variable (31% vs. 52%). This supports the assumption that data were unlikely to be missing at random, with either MSM being more likely to disclose drug use or providers being more likely to ask MSM patients about drug use.
TABLE 6
Description of GUMCAD enhanced surveillance data
Young person model
Variable selection
Deprivation was included as quintiles, based on the UK indices of multiple deprivation111 derived from the patient’s postcode. Age was included in the model as a categorical variable; plotting the relationship between age and STI diagnosis showed that the association was not linear. We described the number of prior STI diagnoses reported, both longitudinally and from patient report. Within this cohort of young people, there were very few non-chlamydia prior diagnoses, and we therefore included prior chlamydia infection only in the model. Ethnicity and location of birth contained a large number of categories, 15 and 9, respectively, adding 23 degrees of freedom to the model. Many of the categories contained < 5% of the patient population; therefore, these variable categories were collapsed to ensure that there were more balanced categories for modelling. Drug use and problematic alcohol use were excluded because of high numbers of missing data, and sexual orientation was excluded for having too little heterogeneity.
Table 7 describes the variables and categories that were included in the primary analysis.
TABLE 7
Variables and their definitions in the primary young person’s model
Primary model
The primary model categorised missing data, retaining all records in the model (Table 8). The model included 34 degrees of freedom and therefore met the required 10 outcomes per degree of freedom. Among young people, females were less likely to have a STI diagnosis [odds ratio (OR) 0.71, 95% CI 0.62 to 0.83] than males, and being older was associated with lower odds of STI diagnosis. Being of black or mixed white and black ethnicity was associated with higher odds of STI diagnosis than being white British.
TABLE 8
Full multivariable logistic regression model for STI diagnosis in the current visit in young people
Behavioural risks included prior chlamydia diagnosis (OR 3.66, 95% CI 2.88 to 4.65), multiple partners in the prior 3 months and having a new partner. Condom use at last sex was protective (OR 0.50, 95% CI 0.41 to 0.62).
The model had reasonable performance, with a pseudo-R2 of 7.8% and a c-statistic of 0.703. The Hosmer–Lemeshow test showed good model fit (p-value = 0.1602). The model predicted probabilities range from 1% to 75%, with a mean of 12%. Using a risk cut-off point of 15%, one would refer 19% of patients, with a sensitivity of 42% and specificity of 84% (Table 9 and Figure 3).
TABLE 9
Sensitivity, specificity, PPV and NPV values for different risk prediction thresholds in the young person’s model
Sensitivity analyses
A model fitted using a forward stepwise approach, using a p-value threshold of 0.2, did not exclude any of the variables and therefore had the same model performance.
A model was fitted using multiple imputation. The following variables underwent 10 imputation rounds using chained equations: location of birth, ethnicity, deprivation, number of partners, new partners and condom use. The model had a pseudo-R2 of 6.6% and a c-statistic of 0.688; the predicted risks ranged from 1% to 68%. Overall, this showed worse discrimination than the model that included categorised missing values.
A model including demographic data only, and fitted using the v2 data set (1,045,373 observations), showed considerably poorer model performance, with a pseudo-R2 of 1.4% and a c-statistic of 0.590. The predicted risk of STI diagnosis was limited, ranging from 2% to 24%, reflecting poor discrimination. A typical high-risk individual based on demographics alone would be an 18- to 19-year-old black Caribbean male, born in Europe and living in an area of high deprivation (predicted risk 23%).
Men who have sex with men model
Variable selection
Similarly to the young person’s model, within the MSM model, age and deprivation were included as categorical variables, and ethnicity and country of birth were reduced to fewer categories because of the lack of heterogeneity within the sample. Within this cohort of MSM, a variety of prior STI diagnoses were reported, including HIV, syphilis, chlamydia and gonorrhoea. Many of these contained too few records to be included as individual predictors; therefore, a single binary variable indicating STI in the prior 12 months was used. Problematic alcohol use was excluded for having too many missing data.
Table 10 summarises the variables in all the models from this point forward.
TABLE 10
Variables and their definitions in the primary MSM model
Primary analysis
The model was fitted, using categorised missing values, with 36 degrees of freedom and may therefore be overfitted (Table 11). In the MSM model, the only significant demographic predictors of STI diagnosis were being of South Asian ethnicity (OR 2.53, 95% CI 1.05 to 6.10) and being born in Europe (OR 2.46, 95% CI 1.26 to 4.78). Significant behavioural risks included having had condomless anal sex in the previous 3 months (OR 1.95, 95% CI 1.39 to 2.73) and any drug use in the prior 3 months (OR 1.89, 95% CI 1.31 to 2.74).
TABLE 11
Full multivariable logistic regression model for STI diagnosis in the current visit in MSM
The model had reasonable performance, with a pseudo-R2 of 7.0% and a c-statistic of 0.676. The Hosmer–Lemeshow test showed good model fit (p-value = 0.224). The model predicted probabilities range from 3% to 71%, with a mean of 16%. Using a risk score threshold of 30% would result in one in five patients being classified as being at high risk of STI diagnosis, with a sensitivity of 38.7% and specificity of 84.8% (Table 12 and Figure 4).
TABLE 12
Sensitivity, specificity, PPV and NPV values for different risk prediction thresholds in the MSM model
Sensitivity analyses
Using a forward stepwise approach to the model, with a p-value threshold of 0.2, excluded age, deprivation quintile, number of partners and ethnicity. This model was favoured according to the BIC statistic, but had a poorer discrimination (c-statistic = 0.658) and model fit (pseudo-R2 = 5.8%); BIC tends to favour parsimonious models, that include fewer explanatory variables.
A model was fitted using multiple imputation, which underwent 10 imputation rounds using chained equations of location of birth, ethnicity, deprivation, number of partners, sex with a known HIV-positive partner, condomless anal sex and drug use in the prior 3 months. The model had a pseudo-R2 of 6.8% and a c-statistic of 0.676; the predicted risks ranged from 4% to 71%. This model showed very similar performance and discrimination to the model that included categorised missing data, and similar direction and magnitude of relationships with the outcome.
A model including demographic data with only the v2 data set (245,863 observations) showed very poor model performance, with a pseudo-R2 of 0.5% and a c-statistic of 0.553. The range of predicted risk of STI diagnosis was limited (7–23%), reflecting poor discrimination. A typical low-risk individual based on demographics alone would be a South Asian aged > 65 years living in an area of low deprivation who was born in Asia (predicted risk 7%). This is contradictory to the v3p2 model, in which being South Asian was one of the main risks for STI diagnosis.
Discussion
We developed two triage tools, one each for young people and MSM groups, based on routinely collected demographic and limited behavioural data as part of a pilot implementation of GUMCADv3. Overall, both models showed borderline reasonable, but not good, performance, with the young person’s model (c-statistic = 0.706) having slightly better performance than the MSM model (c-statistic = 0.676). A c-statistic of > 0.7 is generally considered the threshold for a diagnostic to be clinically reasonable. The inclusion of STI history and behavioural data was crucial to model performance, with models based on demographic data showing very poor performance (c-statistic = 0.590 and 0.553 for young people and MSM, respectively).
Young people
The young person’s model identified several significant predictors of STI diagnosis, as well as protective factors, such as being female, being > 17 years of age and reporting condom use at last sex. This agrees with previously published literature, which has also found older age and condom use to be associated with lower risk of STI diagnosis in other settings.112–114 Similarly, multiple partners and prior diagnoses are established risks for STIs among young people.15,113,115 The finding that young people of black ethnicity (including black Caribbean) or mixed white and black ethnicity are at higher risk of STI diagnosis agrees with previous findings from the UK.116,117 Among young people, possible explanations for this association may be around different levels of SH knowledge, and therefore behaviours, among younger and black ethnic minorities.118
Applying the young person’s model as a triage tool within a clinical setting requires a threshold to be set, with patients having a score above the threshold categorised as being at ‘high risk of STI diagnosis’ and those below the threshold as ‘low risk of STI diagnosis’. The risk predictiveness curve (see Figure 3) shows that most young people were relatively low risk, with predicted risk rising sharply from 20% to 75% in only 10% of the population. Using a predicted risk threshold of > 20%, in which the slope of the curve rises steeply, results in a sensitivity of 25% and specificity of 93%. Applying a lower threshold of > 15% improves the sensitivity to 42% and reduced the specificity to 84%; however, this would double the number of patients classified as being at ‘high risk of STI diagnosis’ (9% vs. 19%). Although this lower threshold increases sensitivity, the feasibility of delivering a brief intervention to one in five young people may not be possible.
Men who have sex with men
The MSM model identified only four significant predictors of STI diagnosis: being of South Asian ethnicity (OR 2.53), being born in mainland Europe (OR 2.46), having had condomless anal sex in the previous 3 months (OR 1.95) and drug use in the prior 3 months (OR 1.89). The use of drugs has been reported as a risk for STI diagnosis by multiple studies,16,20,119 so this finding would be expected. However, the lack of association seen between number of partners and STI diagnosis contradicts multiple studies that have found it to be a significant risk,16,17,120 as was found in young people. In fact, when we used a forward stepwise modelling approach, the number of partners was not retained in the model; nor was age, deprivation or ethnicity. Compared with other reports of risks for different STIs in the UK, the finding that being of South Asian ethnicity is a significant risk was unexpected.121 This may be the result of small numbers of observations (n = 33); a handful of cases in this group could result in a significant relationship.
The risk predictiveness curve for the MSM model (see Figure 4) showed a more consistent increase in risk of STI diagnosis across the population, suggesting that MSM are more likely than young people to be at high risk when the increase is concentrated in a small proportion of the population. Half of MSM have a predicted risk of a STI of > 20%, which explains why the discrimination of this model is poorer than that of the young person’s model. Using a predicted risk threshold of > 30% would result in 20% of the MSM clinic population being classified as having a ‘high risk of STI diagnosis’, with a sensitivity of 39% and specificity of 89%. A threshold of > 20% would give a better balance of sensitivity and specificity (66% and 60%, respectively), but would result in 46% of patients being at ‘high risk of STI diagnosis’.
Implementation challenges
A key challenge of implementing risk scores for triaging in real-world clinical settings is the need to balance sensitivity, specificity and available resources. The aim of this pilot study was to demonstrate the feasibility of triaging patients into different behavioural risk reduction interventions, and crucially, using existing clinical resources. Therefore, the decision about what threshold to use when operationalising the triage is probably driven more by the proportion of patients classified as high risk than by optimising either the sensitivity (identifying more true positives) or specificity (identifying fewer false positives). Based on this being the priority, a risk threshold of 20% for young people and of 30% for MSM may be the best balance between resources, sensitivity and specificity.
A potential challenge for this approach, assuming that all high-risk patients would be referred to an intervention that requires a level of clinic resources, would arise if clinic populations differ dramatically in terms of their demographics and sexual behaviours. A clinic that sees mostly lower-risk patients, for example mostly women of white or Asian ethnicity aged > 18 years, would probably classify less than the expected 9% of high-risk patients. In comparison, a clinic attended by more young black men would probably classify > 9% as high risk, resulting in an unequal burden on resources.
Strengths and limitations
In general, the young people and MSM populations were representative of the wider clinic populations from the five pilot sites in terms of location of birth, deprivation and ethnicity. MSM patients tended to have lower levels of missing data than young people and general populations; therefore, it is likely that the two populations used in the model development reflect the wider population of these clinics. However, these clinics may not be representative of national GUM clinic attendance. The STI rate among the subsample of MSM specifically was higher than the nationally reported rate for the same time period (22% vs. 15%, respectively), although it does not include data from any of the higher-risk London clinics with large MSM populations.18 The pilot clinics were all located in the south of England, and, therefore, the demographic profile of patients within models is unlikely to be generalisable nationally.
A limitation of the v3p2 data set is the number of missing data within the behavioural variables. Although the behavioural variables are recommended as part of the BASHH guidelines100 and are intended to be feasible for collection in routine care, in practice this may not be the case. The number of missing data differs between young people and MSM, suggesting that clinical staff did not address these questions to patients at random but, rather, selected whom they asked and recorded data for based on personal characteristics. For example, a young woman attending a GUM clinic for contraception may be less likely to have her recent sexual behaviour recorded than one attending for a STI screen. We found that drug use was much more likely to have been recorded in MSM than in the general population (49% vs. 31%, respectively), perhaps reflecting an awareness of chemsex being a common high-risk behaviour in MSM. As it is reasonable to assume that the missingness is not random and that there are several mechanisms that could lead to this missingness, our primary models would not have accounted for this. Improving data completeness for the limited behavioural data across the whole clinic population would probably improve model performance and discrimination. This would also allow for additional variables to be included in the triage tool, such as problematic alcohol use.
We did not conduct any internal validation of either model; therefore, we cannot comment on how well the model would generalise to a different data set. The young person’s model, with 1005 outcomes and 34 degrees of freedom, met the rule of thumb to prevent overfitting that there should be 10 outcome events per degree of freedom. The MSM model, however, was fit with 36 degrees of freedom for 318 outcomes; therefore, it is likely to be overfitted, despite having poorer performance. External validation was planned during the pilot feasibility trial implementation, providing a more robust method of model validation than internal validation.109
Conclusion
Triaging patients into high- or low-risk groups based on routinely collected data within SH clinics showed reasonable discriminatory ability; however, at a minimum, basic behavioural data are needed to improve the discrimination of these models. The ability to include additional, or more complete, behavioural data would probably improve performance further. The models were developed using the only data set available at this time, from a pilot that included a small sample of clinics that were not representative of all clinics in the UK (e.g. larger London clinics with a high proportion of high-risk patients were not included). Although the work demonstrated that developing such a tool was possible to a minimal threshold of clinical utility, further refinement and external validation is needed to improve the performance of the tool and assess the real-world applicability of this approach.
- Work package 2: triage tool development - Sexual risk reduction interventions fo...Work package 2: triage tool development - Sexual risk reduction interventions for patients attending sexual health clinics: a mixed-methods feasibility study
- rhodopsin, partial [Fistularia commersonii]rhodopsin, partial [Fistularia commersonii]gi|756766168|gb|AJM87079.1|Protein
- MAG: hypothetical protein CVU88_00110, partial [Firmicutes bacterium HGW-Firmicu...MAG: hypothetical protein CVU88_00110, partial [Firmicutes bacterium HGW-Firmicutes-13]gi|1308725264|gb|PKM84075.1||gnl|WG C|CVU88_00110Protein
- MAG: phosphodiesterase [Firmicutes bacterium HGW-Firmicutes-13]MAG: phosphodiesterase [Firmicutes bacterium HGW-Firmicutes-13]gi|1308725255|gb|PKM84066.1||gnl|WG C|CVU88_00055Protein
Your browsing activity is empty.
Activity recording is turned off.
See more...