NCBI Bookshelf. A service of the National Library of Medicine, National Institutes of Health.
Crawford F, Chappell FM, Lewsey J, et al. Risk assessments and structured care interventions for prevention of foot ulceration in diabetes: development and validation of a prognostic model. Southampton (UK): NIHR Journals Library; 2020 Nov. (Health Technology Assessment, No. 24.62.)
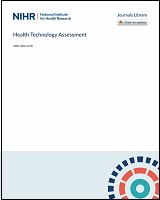
Risk assessments and structured care interventions for prevention of foot ulceration in diabetes: development and validation of a prognostic model.
Show detailsBackground
Diabetes-related foot ulcers give rise to considerable morbidity and generate a high monetary cost for health and social care services. They precede 80% of diabetes-related LEA; hence, the clinical need to identify patients at risk of DFUs as early as possible is clear.
We have developed and validated a CPR suitable for routine practice that can help to identify a patient’s risk status for future diabetic foot ulceration (see Chapter 4). In addition, our SR on the prevention of DFU (see Chapter 6) has identified three interventions that have the potential to reduce the risk of DFUs in patients at risk. Drawing together the CPR and the results of the SR allows us to develop an evidence-based care pathway for the treatment of patients at risk of DFUs.
In this chapter, we report an economic evaluation of this evidence-based care pathway. The purpose of our analysis was to provide an economic rationale for the choice of preventative treatment and optimal monitoring frequency for patients at risk of DFU. We then assess the value of further research in this area and identify particular areas of uncertainty around which future research should be focused.
Objectives
- Question 1: what is the potential cost-effectiveness of the use of a validated CPR as part of structured care to reduce the incidence of DFU?
- Question 2: what is the potential cost-effectiveness of alternative strategies, including monitoring intervals?
- Question 3: is there potential worth in undertaking further research, particularly a RCT?
To address these questions, we first conducted a SR of published health economic models used to estimate the cost-effectiveness of DFU prevention. Informed by the review of the literature, we then developed a de novo health economic model capable of estimating the potential cost-effectiveness of alternative care pathways for the prevention of DFUs. This model was informed by epidemiological modelling of clinical data from a diabetes register in Fife (NHS Fife SCI-Diabetes), Scotland, between 2010 and 2018. Value-of-information analysis was then used to quantify the value of conducting further research and to suggest where such research should be focused.
Literature review of cost–utility analyses
Aim
The aim was to undertake a review of published cost–utility analyses of the prevention of DFUs.
Methods
We undertook a review to identify (1) costs and outcomes of relevant interventions for the prevention of diabetic foot ulceration and (2) published cost–utility analyses of such interventions. The review methods are provided in Appendix 5.
Results
Our search returned 11 relevant papers to be reviewed. Four other relevant papers were identified by ‘hand-searching’ the references of the 11 papers identified. Of these 15 papers, five were cost–utility analyses of the prevention of DFUs.121–125 The remaining papers either were concerned with the cost–utility of treatments for patients who had already experienced DFUs or were papers relating to the cost burden of DFUs.126–134 Further hand-searching did identify other studies in which an economic analysis was undertaken (i.e. some assessment of costs and outcomes); however, as these papers were not cost-effectiveness papers and were beyond the scope of this review, they were excluded.
We review here the five papers that were cost–utility analyses of the prevention of DFUs. Full details of the papers reviewed are given in Appendix 6, Table 52.
Quality of economic evaluations
All five cost–utility papers were quality assessed using the Drummond checklist135 for economic evaluations. All studies were deemed to be of high quality.
Review of papers
Eastman et al.121 used a Markov model to simulate the natural history of diabetic patients and the rate of complications (including DFUs and LEA) and to evaluate the impact of preventative treatments. Information on age, sex, ethnicity and incidence and prevalence rates were based on US community and population studies. The authors then estimated the cost-effectiveness of glycaemic control for reducing diabetes-related complications. Costs and outcomes were estimated for patients aged between 25 and 74 years. The authors found that treatment that maintains an HbA1c value of 7.2% is associated with a reduction in LEA of 67%. They found the cost per additional quality-adjusted life-year (QALY) of treatment was £16,002.
Ragnarson Tennvall and Apelqvist122 used a Markov model to assess the cost–utility of an ‘optimal’ prevention programme for patients at risk of DFUs and amputation. The prevention programme included foot inspection, appropriate footwear and education. Patients were stratified in the model according to their risk of ulceration. The treatment effect of the preventative programme was based on RCTs and observational studies reported in the literature (see Table 21). Costs and outcomes were assessed over a 5-year time horizon. The study found that optimal preventative treatment of high-risk patients was cost saving based on the achievement of a 25% lower incidence of DFUs and extremity amputation than in baseline prevention scenarios. Similarly, Ortegon et al.123 used a Markov model to assess the cost–utility of optimal prevention and treatment of diabetic foot. The preventative programme included protective foot care, education, regular inspection, risk identification and the involvement of a multidisciplinary team. Treatment effect was based on a study that reported a reduction in LEA of between 49% and 85%.135 Patients were stratified in the model according to risk. Costs and outcomes were measured over the lifetime of a patient. The study found that, based on the achievement of a reduction in ulceration incidence of 10%, preventative treatment was cost-effective, with a cost per QALY gained of < US$25,000 (2003 prices).
Rauner et al.124 used a Markov model to assess the cost–utility of preventative education programmes to reduce DFUs. Treatment effect was based on the assumption of a 25–50% reduction in DFUs, based on estimates obtained from the literature.82,136 The authors’ model built on previous work by stratifying patients according to not only risk but also age, allowing for the potential for more targeted decision-making. Costs and outcomes were assessed over a 10-year period. Their results suggest that preventative programmes are highly cost-effective when targeted at patients at high risk of DFUs and LEAs.
Barshes et al.125 used a Markov model to assess the cost–utility of primary prevention programmes aimed at reducing the incidence of DFUs. In contrast to the other papers under review, which took the cost and effectiveness of treatment as given and estimated the cost-effectiveness, Barshes et al.125 sought to estimate the effectiveness target necessary for a treatment to be considered cost saving. Costs and outcomes were estimated over a 5-year period. The study found that preventative programmes had a > 90% probability of being cost-saving when the annual prevention costs were < US$50 per person and/or when DFUs are reduced by at least 25%. If patients were deemed to be at high risk, programmes were cost-saving when incidence of DFU reduced by 10%.
All studies, with the exception of Eastman et al.,121 used a Markov model to simulate the clinically relevant states experienced by patients at risk of developing DFUs. All of these studies included clinical states representing pre-ulceration, ulceration and post-ulceration outcomes; however, they varied in terms of which other relevant clinical factors they included in the modelling. The studies by Ortegon et al.123 and Rauner et al.124 were the only ones to stratify patients according to risk state. Three studies123–125 included additional clinical states associated with diabetic foot, such as ischaemia (e.g. neuropathy, peripheral vascular disease). Eastman et al.121 used a Monte Carlo simulation to estimate the rate of complications, including DFUs and LEAs, associated with all patients with T2DM. The disparity in modelling strategies among these studies is a natural consequence of the complexity of the disease and the range of possible complications. Furthermore, the choice of modelling strategy, and the clinical events included, is a function of the specific decision problem addressed in each study; however, the result is that uncertainty about the most appropriate choice of model for estimating the cost-effectiveness of strategies to prevent DFUs.
All of the papers reviewed found that preventative treatment for DFUs was cost-effective or, in some cases, cost saving. However, the evidence on effectiveness in these studies comes from research conducted some time ago and/or studies that have small sample sizes; therefore, significant uncertainty remains about the treatment efficacy of potential interventions.
Only the model of Ortegon et al.123 estimated the lifetime costs and outcomes relating to DFUs. As the costs (in terms of the ongoing care required) and outcomes (in terms of fewer DFU and amputations), and the significant morbidity associated with these, will have an impact over a patient’s full lifespan, it is necessary to take a lifetime perspective when estimating cost-effectiveness.
All the studies reviewed suggest that the effectiveness (and cost-effectiveness) of treatments is likely to vary according to patient risk, and that treatment is most likely to be considered cost-effective in the subgroup of patients at high risk. However, none of the papers reviewed have modelled how often a patient’s risk should be assessed (risk monitoring frequency).
Summary
Our review highlights considerable heterogeneity in how the clinical and cost consequences of treatments to prevent DFUs have been modelled in the literature. Furthermore, at the time these studies were published, there was little consensus about the efficacy of treatments available for preventing DFUs; therefore, existing models of cost-effectiveness rely on assumptions about treatment effect. Another important limitation of current models is that risk monitoring frequency has not been considered.
Development of health economic model
Overview
There is a lack of consensus among clinical guidelines about the monitoring frequency required for patients who are at risk of developing DFUs. NICE currently recommends annual monitoring of patients at low risk, and monitoring as frequently as once every 1 or 2 weeks for patients at high risk. SIGN recommends that the monitoring take place at least annually, but concedes that the optimal monitoring frequency is unknown; hence, there is a need for new economic evaluations to consider the impact of alternative monitoring frequencies on DFU outcomes and the implications for cost-effectiveness. As discussed in Review of papers, the choice of modelling strategy will depend on the specific decision problem at hand and, thus, clinical input into the model choice is crucial from an early stage. The literature review of economic models has shown that interventions that are able to reduce the incidence of DFUs in at-risk patients have the potential to be cost-effective. Based on our review of the literature, and on advice from our clinical colleagues, we developed a de novo health economic model to answer the questions stated in our objectives.
Cost perspective
This economic evaluation was undertaken from the perspective of the UK NHS and Personal Social Services137 that is, the costs relevant to the economic analysis were those incurred by the NHS and Personal Social Services.
Time horizon
This economic evaluation investigated the costs and health outcomes associated with each clinical pathway over a 20-year time horizon.
Discount rate
Future costs and health outcomes were discounted at 3.5%, in line with recommended practice.137
Conceptual model of disease and treatment pathways
Our task was to undertake an economic evaluation of an ‘evidence-based pathway’ for the prevention of DFUs. To do so, we had to understand the important clinical and economic events in the care pathway. The conceptual model in Figure 18 is a visual representation of the events we sought to capture in our model and how these events relate to costs and QALY outcomes.
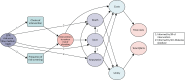
FIGURE 18
Conceptual model of decision problem.
Conceptual model implemented as a Markov model
The model was implemented as a semi-Markov model, as the survival analyses used to estimate transitions indicated that transition probabilities varied according to time in state. Submodels were developed to estimate total discounted costs and QALYs for ulceration and amputation events to simplify the implementation of the model. Figure 19 illustrates graphically the transitions we require to estimate this conceptual model as a Markov model.
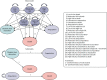
FIGURE 19
Schematic of semi-Markov model and submodel.
NHS Fife Scottish Care Information – Diabetes Collaboration data set
NHS Fife SCI-Diabetes is an electronic patient record used in the routine management of NHS patients with diabetes mellitus. The data set we obtained contained information about individual patients who received foot care in NHS Fife foot monitoring clinics over a 12-year period. The data contained the results of risk assessments of the risk factors recommended by the SIGN guideline,12 only three of which are required in our validated CPR as reported in Chapter 4: monofilament sensitivity, pedal pulses and history of ulceration (all binary variables). Data were available from the beginning of 2005 until the end of 2017. A separate data set from NHS Fife SCI-Diabetes provided death data that were merged with the SCI-Diabetes foot monitoring data. No death data were recorded before 1 March 2009 in the data set; therefore, this date was used as the entry point into the defined cohort as we would not know whether or not patients with records that stopped before that date had died. The use of the SCI-Diabetes anonymised data set was approved by the information governance department of NHS Fife.
Clinical prediction rule definition of risk status
The economic model includes the use of the CPR, which defines a patient’s DFU risk status as follows:
- Low risk [CPR score of 0 or 1; probability of ulcer at 2 years ≤ 0.05 (see Table 10)] – negative for history and also negative for at least one of monofilament sensitivity and pedal pulses.
- Moderate risk (CPR score of 2; probability of ulcer at 2 years = 0.12) – positive for history but negative for both monofilament sensitivity and pedal pulses, or negative for history but positive for both monofilament sensitivity and pedal pulses.
- High risk (CPR score of 3 or 4; probability of ulcer at 2 years ≥ 0.25) – positive for history and also positive for at least one of monofilament sensitivity or pedal pulses.
This categorisation, although arbitrary, was chosen for ease of use in a clinical setting (just three categories of risk) and was decided on following group discussions at project team meetings.
NHS Fife Scottish Care Information – Diabetes Collaboration population
Patients who were recorded in the data before 1 March 2009 and were attending foot monitoring clinics on or after 1 March 2009 (prevalent patients) or who started to attend foot monitoring clinics after this date (incident patients) were defined as eligible for analysis. Having a mix of prevalent and incident patients is appropriate as any changes in the monitoring policy would apply to all patients. In the case of patients whose first recorded appointment was before 1 March 2009, the date of entry to the analysis cohort was considered to be 1 March 2009 and their CPR status was the last recorded CPR status before 1 March 2009. In the case of those patients whose first recorded appointment was on or after 1 March 2009, the date of entry to the cohort was taken as that date, alongside the patient’s CPR status at that date. Use of these criteria resulted in 26,154 patients being included in the cohort used to inform important parameters of the economic evaluation model. Table 22 summarises the key demographics of this cohort.
TABLE 22
Descriptive statistics for cohort used in economic evaluation model
Figure 20 shows the total number of clinical visits per patient in the cohort over the period of approximately 8 years. The follow-up time varied considerably between each patient depending on whether they were a prevalent or an incident patient (and when they became an incident patient) and also if the patient died. As can be seen from Figure 20, almost 80% of patients in the data set had more than one visit to the foot assessment clinic, and it was not uncommon for a patient to have more than 10 clinical encounters.

FIGURE 20
Histogram of total number of clinical visits per patient in the cohort.
Table 23 presents the number of key events of interest for the economic model that occurred during the follow-up of the identified cohort. Among the 26,154 patients, approximately 4% developed an ulcer, 1% required an amputation and almost 24% died.
TABLE 23
Number of key events observed in the cohort used in the economic evaluation model
If the only record for a patient in the data set was of their death (and hence there were no risk assessment data), we excluded that patient from the cohort used for analysis (analysis cohort, n = 26,086).
Missing data
There were significant numbers of missing data in the NHS Fife SCI-Diabetes data set. This was particularly an issue with regard to records for patients’ test results for the three CPR predictors (monofilament sensitivity, pedal pulses and history of ulceration) that were used to estimate risk status in the cohort. To determine the most likely mechanism for the occurrence of missing data, regression modelling was used to investigate the association between patient demographics and missingness. Patients’ characteristics were found to be associated with missingness, ruling out the possibility that the missing data mechanism was missing completely at random.138 After consultations with clinicians and data controllers, we considered that MAR was also unlikely and, therefore, ruled out a multiple imputation approach to account for missing data. After consultating with NHS colleagues, we proceeded with the following rules.
When a patient had a missing record for one of their CPR predictor variables, the patient was recorded as not being at risk from this predictor (i.e. if the record for monofilaments was left blank, we assumed that the patient was sensitive to monofilaments). When a patient had a valid recording (positive or negative) followed by missing data in subsequent visits, we assumed that the patient did not change risk status for this variable unless indicated otherwise. Full details of the missing data approach are given in Appendix 6, Table 54.
Clinical prediction rule risk status over time
Table 24 shows that, at the first clinic appointment, 25,003 patients (96%) were classified as at low risk. This decreased to 23,867 patients (91%) by the final clinic appointment. Over time, there was an increase in patients classified as at moderate risk (first visit, 3%; final visit, 4%) and at high risk (first visit, 1%; final visit, 4%). Overall, 1397 (5%) of the cohort changed their risk status between their first visit and their final visit. The numbers in Table 24 that indicate a change in risk category are shown in italics.
TABLE 24
Frequency of patients by CPR risk status at first clinical appointment and final clinical appointment
Estimation of transition probabilities
Transition probabilities required for the model were estimated based on a set of parametric survival models built on the aforementioned analysis cohort (Table 25). We tested the following parametric models: exponential, Weibull, Gompertz, log-logistic, log-normal and generalised gamma. The choices of distribution were determined by a visual examination of Kaplan–Meier plots and log-cumulative hazard plots and Akaike information criterion (AIC)/Bayesian information criterion (BIC) tests of model fit, as recommended by NICE Decision Support Unit Technical Support Document on survival analysis methodology (see Appendix 6).139 Kaplan–Meier survival plots for the key transitions in the economic model are presented in Appendix 6.
TABLE 25
Numbers of events and estimated survival probabilities (from which transition probabilities are obtained) for all economic model transitions
Results
Estimating costs and utilities
Costs
We calculated total costs by attaching unit costs to resource use per patient with the relevant clinical event. Unit cost estimates were identified from the literature and from microcosting based on input from clinical experts.131 The inclusion and exclusion of resource use items were validated with the project team and diabetic foot clinicians. All unit costs were presented in Great British pounds for the price year 2017. Table 26 provides a list of the unit cost estimates, unit of measurement and source for each resource included in the model. Further details regarding costs are given in Appendix 6.
TABLE 26
Unit costs
Health utilities
Health utilities were identified from the SR of economic models of the prevention of DFU. Utility estimates from Redekop et al.132 were obtained from the general population (the Netherlands), making them particularly suitable for economic evaluation, which requires the relative preferences for health states across multiple disease areas (Table 27).
TABLE 27
Health utilities
Cost-effectiveness analysis
Costs and effects were measured for each potential treatment strategy. The cost-effectiveness of the alternative treatment pathways were evaluated based on their incremental cost-effectiveness ratio (ICER) and their net monetary benefit (NMB). ICERs are calculated as follows:
where ΔCosts is the difference in total costs between interventions and ΔQALYs is the difference in QALY gain between interventions.
If a new treatment is found to be more costly and less effective than the current treatment, the new treatment is said to be ‘dominated’. Conversely, if the new treatment is more effective and less costly than the current treatment, the new treatment is said to dominate the current treatment.
The NMB is a measure of the health benefit, expressed in monetary terms, which incorporates the cost of the new strategy, the health gain obtained and the societal willingness to pay for health gains. The NMB is expressed using the following formula:
where E is effectiveness, WTP is the willingness-to-pay threshold (£20,000 in the UK) and C is cost.
The NMB approach is recommended when comparing more than one intervention and provides a clear decision rule (i.e. if NMB > 0, the new strategy is cost-effective).
Comparators
The base-case treatment strategy in all scenarios is current practice. Current practice is defined as the natural history of the disease, with no CPR to monitor risk, and will involve whatever interventions are currently offered in practice and hence captured in the health outcomes of the current analysis cohort. Current practice is taken as the base case and is compared with the treatment strategies outlined in Table 28.
TABLE 28
Clinical pathways considered in the health economic model
This modelling strategy allows us to incorporate the recommendations of clinical guideline bodies such as NICE and SIGN. Our model included the use of a CPR that is based on a patient’s response to monofilaments, presence or absence of pedal pulses and history of ulceration. These risk factors are recommended, although not exclusively, by NICE and SIGN. There is no evidence-based consensus on the most appropriate strategy for managing patients at risk of DFUs. Although both NICE and SIGN recommend that specialist footwear be considered, only SIGN recommends the use of multidisciplinary care teams. The use of digital thermometry is not recommended in clinical guidelines and is currently the focus of RCTs. SIGN notes that there is no evidence on the ideal monitoring frequency for patients at risk of DFUs; however, it advises annual monitoring. NICE, on the other hand, recommends monitoring annually for low-risk patients, every 3–6 months for patients at moderate risk, every 1–2 months for patients at high risk and every 1–2 weeks for patients for whom there is an immediate, pressing risk of DFUs. By investigating the use of the CPR at 2 years, 1 year and 6 months, our model allows the costs and outcomes associated with a range of preventative strategies recommended by clinical guideline bodies to be explored.
Probabilistic sensitivity analysis
Probabilistic sensitivity analysis was used to explore uncertainty in the model input parameters and to describe the impact that this uncertainty has on the model outcomes, namely costs and QALYs gained. A 1000-iteration Monte Carlo simulation was undertaken. Gamma distributions were used to represent uncertainty in the cost parameters; multivariate, normal distributions were used for (log-)survival regression parameters; beta distributions were used for health utility parameters; and a log-normal distribution was used to represent treatment effect parameters.
The distribution of ICERs produced by the Monte Carlo simulation was presented on the cost-effectiveness plane. Cost-effectiveness acceptability curves (CEACs) were used to present the uncertainty in the decision regarding the most cost-effective option, over a variety of monetary willingness-to-pay thresholds.140
Value-of-information analysis
We conducted a value-of-information (VOI) analysis to determine the value to society of collecting further information on the effectiveness, costs and cost-effectiveness of alternative clinical pathway strategies.
To order to establish whether or not future research would be worthwhile, we calculated the expected value of perfect information (EVPI), which is the difference in net benefit of a decision based on our current information (evidence) and a decision with perfect information (i.e. no uncertainty). The situation of perfect information can be thought of as reducing the width of CIs around all of our model parameters to zero. We estimated the EVPI summed across the potential DFU population in NHS Fife and for a time period during which we expect this treatment strategy to remain the ‘gold standard’. In our analysis, we assumed that a reasonable time period for estimating the VOI would be 10 years. We also assumed that the number of patients in the NHS Fife SCI-Diabetes data set would be the number of eligible patients over a 10-year period (26,086 patients over 8 years in NHS Fife SCI-Diabetes, and hence 3261 patients per year). This equates to an ‘effective population’ (e.g. discounted population) of 15,239 patients, or 1524 patients per year, eligible in Fife. If the EVPI for the population is greater than the costs of carrying out the additional research, then carriyng out this research is potentially cost-effective. To determine what type of new evidence would be most valuable, we calculated the expected value of partial perfect information (EVPPI) for parameters of interest. Owing to the non-normal distribution of NMB, we estimated the VOI using a non-parametric simulation approach.141
Results
Custom-made footwear and offloading
Table 29 presents the results of the economic model in which patients are monitored with the CPR and treated with custom-made footwear and offloading. The results suggest that, in this scenario, treating all patients with special footwear is the most cost-effective strategy.
TABLE 29
Base-case results for patients treated with custom-made footwear and offloading
Digital infrared thermometry
Table 30 presents the results of the economic model in which patients are monitored with the CPR and treated with digital infrared thermometry. The results suggest that, in this scenario, treating all patients with digital thermometry is the most cost-effective strategy.
TABLE 30
Base-case results for patients treated with digital infrared thermometry
Complex interventions
Table 31 presents the results of the economic model in which patients are monitored with the CPR and treated with complex interventions. It can be seen that the expected costs of the different strategies with complex interventions are higher than for the others, but with no advantage in QALYs. As a result, at a willingness to pay of £20,000, current practice is the preferred option.
TABLE 31
Base-case results for patients treated with complex interventions
Probabilistic sensitivity analysis
Cost-effectiveness planes
Figures 21–23 are graphical illustrations of the uncertainty surrounding the ICERs produced from a 1000-iteration of a Monte Carlo simulation. All three simulations suggest that there is considerable uncertainty surrounding the QALYs associated with each treatment strategy; however, there is comparatively less uncertainty around the total costs. Figure 23 shows that treating all with complex interventions is likely to have a significantly higher total cost than other treatment strategies.

FIGURE 21
Distribution of incremental costs and QALYs associated with custom-made footwear and offloading.
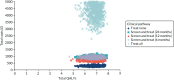
FIGURE 23
Distribution of incremental costs and QALYs associated with complex intervention.

FIGURE 22
Distribution of incremental costs and QALYs associated with digital infrared thermometry.
Cost-effectiveness acceptability curves
Figures 24–26 show the probability that each treatment strategy is considered cost-effective, compared with the alternatives, for a range of thresholds of willingness to pay for a single QALY gain. Figures 24 and 26 show considerable uncertainty surrounding which intervention is most likely to be deemed cost-effective, with no clear strategy producing the greatest probability at a willingness-to-pay threshold of £20,000 per QALY gained. Only in the case of digital infrared thermometry (see Figure 25) does the treat-all strategy emerge as having the greatest probability of being cost-effective, although, even for this intervention, the CEAC suggests a probability of just over 30% that this strategy is the most cost-effective at a willingness-to-pay threshold of £20,000 per QALY.

FIGURE 24
The CEAC for custom-made footwear and offloading.
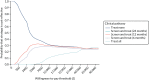
FIGURE 26
The CEAC for complex intervention.

FIGURE 25
The CEAC for digital infrared thermometry.
One-way sensitivity analysis
One-way sensitivity analysis assessed the impact on cost-effectiveness of varying our chosen willingness-to-pay threshold from £20,000 per QALY gained (as recommended by NICE) to £13,000 per QALY gained (Claxton et al.142). This had the effect of reducing the cost-effectiveness of custom-made footwear and offloading (from an incremental NMB of £766 to £250) and of infrared thermometry (from an incremental NMB of £1802 to £1139). The use of complex interventions is still considered not to be cost-effective. There was no change in the relative position of the cost-effectiveness of screening intervals.
Value of information
The VOI analysis is conducted for the three potential interventions separately, as these are mutually exclusive. In all VOI analyses that follow, the most cost-effective option for each intervention is considered against current practice.
The EVPI per patient affected by the decision to recommend treatment based on a strategy of treating all with custom-made footwear and offloading is estimated to be £9226.
Based on our assumptions of an effective population of 1218 eligible patients per year in NHS Fife and at a willingness-to-pay threshold of £20,000 per QALY gained, this equates to an EVPI of £11.2M per year. (Figure 27 shows how this varies with willingness-to-pay threshold.) If we assume that the period of time for which this treatment strategy would be expected to be considered ‘gold standard’ is 10 years, then the estimated total population EVPI is £112.4M over a 10-year period for the NHS Fife diabetes mellitus population.

FIGURE 27
The EVPI (population, £), based on custom-made footwear and offloading.
The EVPPI suggests that the majority of the value of reducing parameter uncertainty in our model would be in reducing uncertainty around the parameters obtained from our survival analysis of NHS Fife SCI-Diabetes data (Figure 28). This suggests that the uncertainty having the greatest impact on our decision problem is related to the number of events (ulceration, amputation, death) in the NHS Fife SCI-Diabetes data set.

FIGURE 28
The EVPPI per patient (£), based on custom-made footwear and offloading.
Digital infrared thermometry
The EVPI per patient affected by the decision to recommend treatment based on a strategy of treating all with digital infrared thermometry is estimated to be £8533.
Based on the same assumptions as above with regard to eligible population, time horizon and willingness to pay for QALY gains, we estimate an EVPI of £10.4M per year and of £104M over 10 years. Figure 29 illustrates how this EVPI varies with willingness-to-pay threshold.
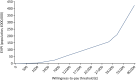
FIGURE 29
The EVPI (population, £), based on infrared digital thermography.
The EVPPI suggests that the majority of the value of reducing parameter uncertainty in our model would be generated from reducing uncertainty around the parameters obtained from our survival analysis of NHS Fife SCI-Diabetes data (Figure 30).

FIGURE 30
The EVPPI per patient (£), based on digital infrared thermometry.
Complex intervention
The EVPI per patient affected by the decision to recommend treatment based on a strategy of treating all with complex interventions is estimated to be £8968.
Based on the same assumptions as above with regard to eligible population, time horizon and willingness to pay for QALY gains, we estimate an EVPI of £10.9M per year and of £109M over 10 years. Figure 31 illustrates how this EVPI varies by willingness-to-pay threshold.
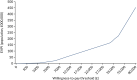
FIGURE 31
The EVPI (population, £), based on complex intervention.
The EVPPI suggests that the majority of the value of reducing parameter uncertainty in our model would be generated from reducing uncertainty around the parameters obtained from our survival analysis of NHS Fife SCI-Diabetes data (Figure 32).
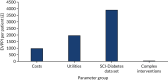
FIGURE 32
The EVPPI per patient (£), based on complex interventions.
Across all three interventions available, the VOI analysis suggests that there is value in reducing the uncertainty related to the decision problem (i.e. whether or not it is cost-effective to use this treatment strategy). In terms of the specific groups of parameters we looked at, there was value in reducing the uncertainty relating to all of them, but reducing the uncertainty relating to the NHS Fife SCI-Diabetes data had the greatest value across all three analyses. This suggests that increasing our knowledge of the epidemiology of DFUs, in terms of how patients move between risk states and how often events occur will allow us to better capture the true cost and outcomes associated with our proposed treatment strategy, and allow us to minimise the health losses from choosing the wrong treatment strategy.
Discussion
Summary of principal findings
- Our analysis of the NHS Fife SCI-Diabetes population that attend foot clinics suggests that patients’ DFU risk does not readily change over time.
- Despite the significant uncertainty, our health economic model suggests that preventative DFU interventions have the potential to be considered cost-effective.
- The CPR developed in this study can be used as a tool for identifying patients who are suitable for treatment.
In this chapter, we have (1) undertaken a review of published cost–utility models for the prevention of DFUs; (2) used this information, alongside input from clinical experts, to develop a health economic model capable of estimating the cost-effectiveness of DFU prevention treatments and alternative monitoring frequencies with a CPR; and (3) estimated the value of further research and suggested where this research should focus. Our results suggest that preventative treatments for DFUs have the potential to be considered cost-effective at a willingness-to-pay threshold £20,000 per QALY gained.
Our model included the use of annual monitoring with the CPR, as recommended by NICE and SIGN. We also looked at the impact of biannual monitoring and monitoring every 6 months; however, our model did not look at the strategy of monitoring only high-risk patients as often as every 1–2 months or monitoring patients of immediate concern every 1–2 weeks. As noted Chapter 3, Methods, our design of the economic model was influenced by the results of the survival analysis of the NHS Fife SCI-Diabetes data. Analysis of these data suggests that patients do not readily change risk status (i.e. move from low to moderate risk or from moderate to high risk). Indeed, over the 8 years for which we had data, just 5% of individuals changed risk status; therefore, it is unlikely that increasing the monitoring frequency from 6-monthly (which is included in the model) to, for example, every 1–2 months or every 1–2 weeks, will capture enough additional events to offset the additional monitoring costs.
Further research aimed at predicting which patients will change risk status is warranted, as these are the patients best placed to benefit from preventative treatment. Further research, for example in the form of developing a prognostic model capable of predicting the patients whose risk status is likely to change, rather than those who are likely to develop ulceration, would be welcome.
In conducting an economic evaluation of the introduction of the CPR, we needed to compare the effect of directing therapy with and without the CPR. In the model, we have assumed that if we do not have the CPR we have two options: give no-one the intervention(s) or give everyone the intervention(s). This is rather simplistic given that some kind of risk assessment currently takes place;28 however, given the multiple combinations of treatment strategies across both the NICE and the SIGN guidelines, in terms of risk factors, methods of assessment, and interventions and monitoring frequencies, it would have been challenging to define a specific strategy including a CPR that could constitute ‘current practice’. Hence, our comparison with ‘no CPR’ allows us to estimate the potential value of the new CPR and the value of further research into the new CPR.
In our analysis, we investigated the impact of introducing a CPR based on three risk factors. Current guidelines on how patients should be assessed for risk involve a greater range of potential risk factors (NICE: insensitivity to a 10-g monofilament, absence of pedal pulses, presence of significant structural abnormality, neuropathy disability score (> 5), history of ulceration, more formal assessments using a biothesiometer, Doppler ultrasound and/or an ABI test; SIGN: insensitivity to a 10-g monofilament, limb ischaemia, ulceration, callus, infection or inflammation, deformity, gangrene, amputation, Charcot arthropathy). Given the range of potential risk factors suggested by NICE and SIGN, and the lack of clarity about the adherence of clinicians to any particular strategy, it was not possible in our study to include the use of a current prognostic model to identify patients at risk of DFUs, with which we could compare the new CPR model developed in this study. Further research aimed at comparing different prognostic models would be welcome. Treating all patients attending a foot screening clinic is not currently recommended by the NICE or the SIGN guidelines; however, considering a ‘treat all patients attending foot screening’ strategy, and hence not using a CPR, allows us to distinguish between the value of introducing the CPR and the value of the interventions themselves.
The meta-analysis and economic modelling of potential preventative treatments suggests that custom-made footwear and offloading and digital thermometry have the potential to be cost-effective; however, given that patient adherence to both of these treatments is unknown and likely to be a critical factor, further research into patient acceptability of and adherence to these treatments would be welcome.
Our VOI analysis suggests that reducing the uncertainty about the epidemiology of DFUs is likely to produce the greatest value. This may be partly explained by the small number of ulceration and amputation events in this data set and, hence, the associated uncertainty. To reduce this uncertainty, a study similar to the one presented in this chapter could be run using diabetes mellitus register data for the whole of Scotland or the UK, and for longer follow-up periods, to reduce the uncertainty related to the number of events.
Our VOI analysis also suggested that there was little value in further research relating to evidence on the treatment effect of interventions; however, it should be stressed that only one form of uncertainty is captured by the VOI analysis, namely the uncertainty related to the parameters estimated and used in the health economic model (i.e. the CIs around the estimate). The analysis does not capture the uncertainty related to issues such as publication bias and heterogeneous populations in the original studies that informed the treatment effect estimates. Hence, the decision uncertainty relating to the treatment effect in our VOI analysis (and the value of reducing this) is likely to be an underestimate. It should also be noted that in our analysis the treatments were regarded as mutually exclusive; however, in reality a combination of these treatments could be offered to patients. Our analysis also assumed that treatment effect remained constant across level of patient risk, as the relative effectiveness of these treatments in patients with different levels of risk is unknown. In addition, the effectiveness of any of these treatments used at alternative monitoring frequencies is also unknown; hence, the effectiveness of combined treatments (for specific risk groups, and used at alternative frequencies) should be explored further. To address the uncertainty surrounding treatment efficacy, a RCT would be required; however, we found that, in an at-risk population of (approximately) 26,000, few patients changed risk status, or developed ulceration, or received amputations over an 8-year follow-up period. Given this, the size, duration and cost of a RCT in this area, and what it would add compared with a large observational study (based on routine data sources), would need to be considered carefully.
Strengths of this study
The NHS Fife SCI-Diabetes data set was obtained from monitoring patients in routine clinical practice. This has given us an insight into the treatment of patients in a ‘real-world’ setting. The data set contained > 26,000 patients followed up over an 8-year period. This allowed us to assess empirically how risk of ulceration changes over time.
As part of this study, we developed a de novo health economic model (guided by the advice of clinicians involved in the prevention of DFUs) capable of including both the use of a CPR and the costs and outcomes associated with alternative clinical pathways.
Limitations of this study
The NHS Fife SCI-Diabetes data set was obtained by monitoring patients in routine clinical practice (i.e. it was not designed to be used for research); therefore, considerable data cleaning and manipulation was required. Despite this, issues of missing data remained in the data set. In particular, patients’ results for the three predictive tests included in the CPR were frequently missing. Furthermore, the project team’s clinicians suggested that amputation events may be under-recorded. This is because patients who progress to the stage of requiring an amputation may no longer be seen by the foot care team and, as a result, their data are not captured by risk assessment monitoring. For each variable in our analysis, we have chosen what we believe to be the most appropriate form of imputation – that is, what we believe is most likely to produce a consistent and plausible record of patient monitoring, reflecting what is most likely to have happened in clinical practice.
Costs of ulceration were identified from the literature.131 As patients may be treated either in an inpatient setting or in an outpatient setting, our cost of ulceration is a weighted average of the two costs, assuming that 90% of patients are treated in an outpatient setting (based on clinical expert opinion). Amputation costs were weighted according to which type of amputation was required. Based on the literature, we assumed that two-thirds of amputations that are required are minor and that one-third are major.143 There was also some uncertainty about the costs of interventions. Digital infrared thermometry involves a relatively inexpensive device; however, because of the lack of data on its use in routine practice, it is not clear what other resource may be required to implement this treatment strategy (e.g. contacting nurses to report high temperatures and to seek further advice). The precise elements of a ‘complex intervention’ vary across the UK, with no single agreed-on approach or clarity about the best types of specialist care required; therefore, our estimate of resource use for complex intervention may differ from that delivered in practice across the UK.
The diabetes mellitus population used in our analysis was a mix of an incident and prevalent population. This was justified on the grounds that any new monitoring policy would probably be introduced for all patients, rather than only for new patients; however, it should be noted that we do not explicitly model the process of patient entry and we take the estimates from the survival analysis as representing a static cohort. Although this is not strictly technically correct, we believe that this is a reasonable compromise to simplify the modelling.
Our choice of low, moderate and high risk of DFUs was based on the predicted probabilities of DFUs from the CPR and was agreed in consultation with the wider project team; however, it should be noted that, based on our definitions of risk, the proportion of patients classified as high risk is lower than that found in other studies. This may have implications for our findings regarding the lack of movement between risk states over time. Further work on this model should include investigating the impact on cost-effectiveness of alternative definitions of risk according to the CPR; however, we do not believe that this is likely to significantly alter our overall results owing to the significant uncertainty in the epidemiology of the NHS Fife SCI-Diabetes data, the true costs of interventions and the effectiveness of potential interventions.
Implications of our findings
- The finding that patients’ DFU risk status does not change readily suggests that a move towards less frequent risk monitoring of at-risk patients would be acceptable.
- As the proportion of patients whose DFU risk status changes over time is low (5%), but the costs and health outcomes associated with this group are significant, further work to predict those patients whose risk score is most likely to change would be welcome.
- There is a need for further research into the effectiveness and acceptability of and adherence to potential preventative DFU interventions.
- There is a need to better understand what constitutes ‘current practice’ in DFU prevention across the UK, in terms of risk assessment methods (risk factors and how they are assessed), interventions offered and adherence to clinical guidelines.
Conclusion
Our analysis suggests that DFU treatments have the potential to be cost-effective at a willingness-to-pay threshold of £20,000 per QALY gained. In the case of custom-made footwear and offloading and digital infrared thermometry, our results suggest that treating all patients attending routine foot monitoring is likely to be considered the most cost-effective strategy, with the additional cost of these strategies compensated for by the additional increase in QALYs. In the case of complex interventions, our results suggest that these treatments are not likely to be considered cost-effective, regardless of monitoring frequency. This is because of the significant cost associated with complex interventions.
Our probabilistic sensitivity analysis suggests that there is considerable uncertainty surrounding which treatment strategy is most likely to be considered cost-effective at our willingness-to-pay threshold. This is driven primarily by the uncertainty surrounding the QALY gain associated with each treatment strategy. Our VOI analysis suggests that there is value in conducting further research in this area and, specifically, in reducing the uncertainty about how many patients experience ulcers, require an amputation or die over a given time horizon.
Given our findings, we believe that preventative treatments for patients at risk of DFUs have the potential to be cost-effective, but that significant uncertainty remains surrounding these findings; therefore, further research would need to be undertaken before we could recommend an optimal treatment strategy.
- Economic model: evidence-based pathway - Risk assessments and structured care in...Economic model: evidence-based pathway - Risk assessments and structured care interventions for prevention of foot ulceration in diabetes: development and validation of a prognostic model
- Introduction - Clinical effectiveness and patient perspectives of different trea...Introduction - Clinical effectiveness and patient perspectives of different treatment strategies for tics in children and adolescents with Tourette syndrome: a systematic review and qualitative analysis
- Trial management, governance and conduct - The Age of BLood Evaluation (ABLE) ra...Trial management, governance and conduct - The Age of BLood Evaluation (ABLE) randomised controlled trial: description of the UK-funded arm of the international trial, the UK cost–utility analysis and secondary analyses exploring factors associated with health-related quality of life and health-care costs during the 12-month follow-up
- Assessment of cost-effectiveness - Biomarkers for assessing acute kidney injury ...Assessment of cost-effectiveness - Biomarkers for assessing acute kidney injury for people who are being considered for admission to critical care: a systematic review and cost-effectiveness analysis
- Development and validation of pre-eclampsia prediction models - Validation and d...Development and validation of pre-eclampsia prediction models - Validation and development of models using clinical, biochemical and ultrasound markers for predicting pre-eclampsia: an individual participant data meta-analysis
Your browsing activity is empty.
Activity recording is turned off.
See more...