NCBI Bookshelf. A service of the National Library of Medicine, National Institutes of Health.
Allotey J, Snell KIE, Smuk M, et al. Validation and development of models using clinical, biochemical and ultrasound markers for predicting pre-eclampsia: an individual participant data meta-analysis. Southampton (UK): NIHR Journals Library; 2020 Dec. (Health Technology Assessment, No. 24.72.)
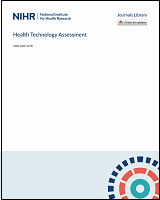
Validation and development of models using clinical, biochemical and ultrasound markers for predicting pre-eclampsia: an individual participant data meta-analysis.
Show detailsSummary of the findings
Existing prediction models for any-, early- and late-onset pre-eclampsia IPD have poor to average predictive performance when externally validated in the combined IPPIC-UK data sets. All models that could be validated showed suboptimal predictive performance across data sets. The clinical utility of the published models was poor.
Most of the IPPIC pre-eclampsia models showed good to average discrimination across data sets; all had good to average calibration across data sets. The models varied in the predictive performance between data sets. The clinical characteristics only and the clinical and biochemical first- and second-trimester models to predict any pre-eclampsia showed consistent net benefit over a strategy of considering all women to have pre-eclampsia for a wide range of probability thresholds beyond 5% in cohort of nulliparous women with singleton pregnancies in the UK. Very few risk factors were associated with pre-eclampsia, with significance in both the CIs and the prediction intervals including BMI, SBP and DBP and MAP for any-, early- and late-onset pre-eclampsia; and urine dipstick, uterine artery pulsatility index and umbilical artery pulsatility index for any- and early-onset pre-eclampsia.
Strengths and limitations
We used the largest data set to date to validate existing prediction models for pre-eclampsia, and to further develop models when required. The IPPIC data set consists of information on predictor variables at various trimesters. We used raw data to determine the presence or absence of pre-eclampsia and the type of onset, ensuring the reliability of the findings. Our comprehensive search identified all published models. By validating them in UK data sets, we were able to assess the extent of transportability of existing models to women managed in the NHS. We assessed the quality of the data sets and studies using robust tools. Rather than develop further models, we ensured that the performances of the existing models were robustly evaluated. The models were validated not only using evidence synthesis, but also within individual data sets. The large sample size provided us with sufficient events for the rare but important outcome of early-onset pre-eclampsia. In addition to reporting the performances of the models in terms of discrimination and calibration, we determined their clinical utility using decision curve analysis.
Prior to the development of the IPPIC pre-eclampsia models, we prioritised the predictors for importance to clinical practice by consensus to ensure face validity. We used multiple imputation to deal with missing values for both predictors and outcomes to avoid the loss of useful information84,240 and explored complex associations such as the non-linearity of predictor effects. We were able to report the association between individual clinical, biochemical and ultrasound predictors, measured in the first, second or third trimester, and rates of early-, late- and any-onset pre-eclampsia with very precise estimates. We pooled data from a very large sample size using IPD meta-analysis, and explored a considerable number of risk factors thought to be predictors of pre-eclampsia. We did not dichotomise any of the continuous predictive factors and we also considered the predictive accuracy of these risk factors along with their association.
Our findings were limited by the variations in population mix, the definitions of the predictors in each study and the outcomes reported. Some studies included only nulliparous women, some strictly included low-risk pregnancies and some included all pregnancies. The prioritisation of predictors of pre-eclampsia by members of the collaborative network who contributed data to the project could also be considered a limitation in the identification of predictors to be considered for model development. It was possible that participants in the survey would rank predictors as important based on their particular research interest, and this method could potentially hamper the identification of new candidate predictors. However, we had a good representation of responses to the survey, and respondents were able to suggest possible factors not already assessed in the survey to be considered as predictors. There was also good consensus among respondents about the importance of predictors assessed, and no new candidate predictors were identified. The individual UK data sets measured different sets of variables (potential predictors) and measured them at different times (e.g. first, second or third trimester). Our validation was carried out considering only UK data sets to reduce the heterogeneity in the outcome definition and to allow existing models’ predictive performance to be assessed in the UK health-care system context. However, this limited our ability to validate many of the existing prediction models and meant that we could validate models across the studies only if all of them reported the same variables. We were, therefore, also unable to validate all existing models in our IPD because of the unavailability of predictors in the models in our UK IPD. It is possible that a significant predictor may not have been evaluated if it was not provided across varied data sets. Some studies used data from the same cohort of women to report various prediction models in multiple combinations. The sources of the data also differed across data sets. Some were collected prospectively with the explicit purpose of predicting pre-eclampsia, whereas others were routine registry data. All of the above accounted for the heterogeneity observed in the performance of the models across the data sets. We validated the performances of published models across only UK data sets, but included all data sets for model development. It is likely that the transportability performances may have differed if all available data had been included. Furthermore, some models, such as the North et al. model42 for any-onset pre-eclampsia, the Poon 2009 model234 for early-onset pre-eclampsia and the Akolekar et al. model241 for late-onset pre-eclampsia, could not be validated as the predictor variables in the models were not available in any of the IPPIC-UK data sets.
To ensure that the relevant data were included in the analysis, we dealt with missing data by imputing both within and between studies. We also made assumptions such as using early second-trimester values of BMI and MAP if the first-trimester values were missing. Our analysis of the association of risk factors and the different pre-eclampsia outcomes was limited to complete records only. Our assumption that data missing were missing completely at random is unlikely to be true. Applying multilevel multiple imputation would reduce this bias, and also allow estimates to be adjusted for potential confounders, giving a better evaluation of associations. However, the complexity of modelling the missing data mechanism would make this demanding (or impossible). We therefore present this as the most robustly available assessment of risk factors for pre-eclampsia.
Comparison with existing evidence
Current guidelines such as those by the National Institute for Health and Care Excellence242 in the UK and by the American College of Obstetricians and Gynecologists73 in the USA provide a list of risk factors rather than a prediction model to determine an individual’s risk of pre-eclampsia. The predictive performance of both approaches has been shown to be inferior to that of multivariable prediction models.236,243,244 However, these models had not been externally validated in multiple data sets until now, with resulting suboptimal predictive performance.
Until now, only a small fraction of the 131 pre-eclampsia prediction models identified have been externally validated (11%, 15/131), and an even smaller proportion (4%, 5/131) have been evaluated for their clinical utility.44,108,160,245,246 Studies reporting on the external validation of these models often have not reported performance measures in terms of calibration, which has more value in assessing the predictive performance of the model than discrimination estimates or detection rates.247 Some existing models also used multiples of the mean to standardise biochemical and ultrasound markers, but this is of limited use in real-world settings as the estimates of adjustment factors used to standardise these measurements to multiple of the mean values in the models are not always known in the population in which the model is to be used. For some of the models that we could validate, the summary performance measures have a lot of uncertainty (wide CIs), reflecting the small numbers of events and/or the heterogeneity. Even with larger numbers of events, CIs can be wide, especially for calibration, so it is possible that, for some of the models, miscalibration is due to chance. However, we must also look at the broader picture emerging across all of the validations. For most models, the majority of summary results for calibration, and the study-specific results for calibration, are suggestive of overfitting (slopes < 1). This is something that the field needs to address as whole. In our IPPIC models, we have examined overfitting in our model development, and adjusted for it.
Recently, the ASPRE trial34 showed that women at high risk of preterm pre-eclampsia who were started on 150 mg of aspirin early in pregnancy had their risk lowered, and their high-risk status was determined using a prediction model. However, a few questions need to be answered before this approach is implemented. First, the extent to which the model over- or underpredicted women’s pre-eclampsia risk is not reported in the study, because women assessed as being at low risk of pre-eclampsia using the model were not followed up further. This is also shown by the significant difference in the incidence of preterm pre-eclampsia between the placebo group and the population used to develop the model. Second, it is likely that some women in the group categorised as ‘low risk’ using the model may have benefited from the intervention. In the absence of follow-up of this group for pre-eclampsia outcomes, we cannot robustly confirm that they would not have benefited from the intervention. Third, the clinical utility of the prediction model has not been assessed, limiting our ability to recommend its routine use in clinical practice. We were unable to validate the exact model used in the ASPRE trial because the multiple of the mean predictors were unavailable in our IPD data set. The authors who developed this model recently validated it in three data sets with ‘appropriately trained staff and quality control of measurement’.248 This showed that the model discriminated well, with a large C-statistic in all three validation data sets. However, further independent validation of this model is needed to evaluate its performance in ‘real-world’ settings.
Although some of the published models showed a promising ability to discriminate between women who had a pre-eclampsia outcome and those who did not, calibration performance was generally poor across the data sets and there was large heterogeneity in the calibration performance across different IPPIC data sets. Although the CIs (e.g. for calibration slope) were sometimes very wide, a general picture is that most models demonstrated overfitting at model development with predictions that were too extreme compared with the observed risk in the data sets (calibration slope < 1). Model predictions were also systematically too low or too high depending on the data set used to validate the model (calibration-in-the-large ≠ 0). The models were validated in a rather heterogeneous group of data sets with different eligibility criteria. These findings suggest that the differences between women in the data sets were not adequately captured by the set of predictors included in the models. There was also little difference in predictive performance when biochemical markers or ultrasound markers were combined with maternal and clinical characteristics, compared with models with only maternal and clinical characteristics. Some of the heterogeneity in predictive performance of the models is likely to be due to different methods and timing of measurement, for example in blood pressure and biochemical marker values. Going forward, standardisation of measurement methods, for example across laboratories and hospitals, might reduce heterogeneity in calibration performance. A related point is that prediction models in this field need to be clearer with regard to how and exactly when included predictors should be measured.
For IPPIC models, summary predictive performance was promising, and net benefit was demonstrated in some data sets across clinically relevant thresholds of predicted risk. However, large heterogeneity remained in all performance statistics across data sets. Heterogeneity in calibration performance could be reduced if, when applying the models in practice, model parameters (e.g. intercept) could be recalibrated to each population and setting. This would require local data for recalibration and model updating.
Relevance to clinical practice
Existing models that were externally validated had poor calibration performance and their utility was limited, with no model identified that can be recommended for clinical use. The IPPIC models showed promising performance for predicting pre-eclampsia, in particular in both low- and middle-income countries where only clinical characteristics may be available, and in high-income countries where there is access to additional biochemical markers. However, on application, the predictive performance of the models needs to be improved by recalibration to particular settings and populations; this would require local data. Ultrasound markers did not add any additional information or improve the performance of the prediction models beyond the clinical characteristic only models. This suggests a lack of need for additional time or resources in carrying out these assessments for screening of women at risk of pre-eclampsia. The thresholds of risk on which decision-making is based are likely to vary with the planned intervention (aspirin or calcium), as well as following shared decision-making through discussions between the clinician and the woman.
Relevance to research
Validation, including examination of calibration heterogeneity, is still required for the models we could not validate. For these and the IPPIC models, we need validation in multiple large data sets across different settings and populations to properly assess their transportability.249 The impact of using the models in clinical practice needs to be evaluated beyond predicting pre-eclampsia, but also in the identification of women with pre-eclampsia who are also most likely to have severe complications such as HELLP syndrome, eclampsia, abruption or renal failure. The acceptability of the models to both women and health-care professionals needs to be assessed, including elucidation of their preferred threshold probability for treatment decisions. A decision-analytic model of resource implications, including the cost utilities of consequences of decisions for various false-positive and false-negative cases, is also needed. Updated models may be needed in local populations, for example using recalibration of the IPPIC models in local data sets, to improve calibration performance. Furthermore, additional strong predictors need to be identified to improve model performance and consistency. New cohorts need to standardise the predictors and outcomes measured, including their timing and measurement methods, to enable more homogenous data sets to be combined in IPD meta-analyses.
Conclusion
Among the 24 existing prediction models that could be validated in the IPD meta-analysis, generally their predictive performance was poor across data sets. To address this, IPPIC models were developed with adjustment for overfitting, which show good predictive performance on average across data sets and may have net benefit in singleton nulliparous populations in the UK. However, heterogeneity across settings is likely in calibration performance, and thus the models need to be recalibrated in local settings and populations of application. Ultrasound markers did not improve the predictive performance of the developed IPPIC clinical characteristic-only models. We did not identify any new predictors for our model development that were not considered previously in existing models. Further work is therefore needed to validate other models, identify new predictors and improve calibration performance in all settings of intended use.
- Discussion - Validation and development of models using clinical, biochemical an...Discussion - Validation and development of models using clinical, biochemical and ultrasound markers for predicting pre-eclampsia: an individual participant data meta-analysis
- hypothetical protein AeMF1_009603 [Aphanomyces euteiches]hypothetical protein AeMF1_009603 [Aphanomyces euteiches]gi|2180795211|gb|KAH9116461.1||gnl| AJNMJ|cds.AeMF1_9647.1Protein
- A systematic review and economic evaluation of diagnostic strategies for Lynch s...A systematic review and economic evaluation of diagnostic strategies for Lynch syndrome
- Dynlrb2 dynein light chain roadblock-type 2 [Mus musculus]Dynlrb2 dynein light chain roadblock-type 2 [Mus musculus]Gene ID:75465Gene
Your browsing activity is empty.
Activity recording is turned off.
See more...