NCBI Bookshelf. A service of the National Library of Medicine, National Institutes of Health.
Cockayne S, Pighills A, Adamson J, et al. Home environmental assessments and modification delivered by occupational therapists to reduce falls in people aged 65 years and over: the OTIS RCT. Southampton (UK): NIHR Journals Library; 2021 Jul. (Health Technology Assessment, No. 25.46.)
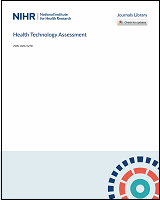
Home environmental assessments and modification delivered by occupational therapists to reduce falls in people aged 65 years and over: the OTIS RCT.
Show detailsIntroduction and aim
This chapter reports the methods and results of the economic evaluation that was undertaken as part of OTIS. The aim was to establish the cost-effectiveness of OT-delivered environmental assessment and modification (the intervention) compared with usual care, incorporating the impact of the intervention on both participants’ quality of life and falls prevention.
Methods
The within-trial economic evaluation involved:
- a cost–utility analysis, which evaluated the intervention compared with usual care in terms of the incremental cost per quality-adjusted life-year (QALY)
- a cost-effectiveness analysis, which assessed the intervention in terms of the cost per fall averted, thereby using the primary outcome measure of the trial.
The analyses included all costs related to health-care services used by the participant over the course of the trial, plus the cost of the intervention itself.
Within-trial economic evaluation
For both cost–utility and cost-effectiveness analyses, the base-case analysis was undertaken on an ITT basis from the perspective of the UK NHS and Personal Social Services (PSS), which included only health-care services and social services used by the participant during the trial that were related to falls. The base-case analysis used a multiply imputed data set, with the complete-case scenario also explored via sensitivity analysis. A secondary analysis was conducted from the societal perspective, which incorporated indirect costs in addition to the direct costs captured in the base-case analysis. A 12-month time horizon was used for the analyses, in line with the 12-month trial follow-up, and hence discounting of costs and health benefits was not required.
Economic data collection
Data regarding health-care resource use and health outcomes were collected during the 12-month follow-up period of the study. Health service utilisation and health-related quality of life data were collected using the questionnaires administered to participants at baseline and at 4, 8 and 12 months post randomisation. Falls data were collected via monthly falls calendars which were self-completed by participants. The equipment recommended by the OTs was documented using an equipment list that they completed at the home visit: confirmation that the equipment had been issued/installed was collected during the 4- to 6-week follow-up telephone call.
Health-related quality of life
The outcome for the cost–utility analysis was the QALY, with utilities used to represent health-related quality of life. QALYs provide a generic metric for the measurement of health that combines the impact on both longevity and quality of life, with one QALY equivalent to 1 year in full health.41 Utility values for the different health states experienced by participants were estimated using the EQ-5D-5L, following NICE appraisal guidance.42
The EQ-5D-5L43 was utilised to obtain utilities from participants at the four time points via participant questionnaires. The EQ-5D-5L comprises five domains (mobility, self-care, usual activities, pain/discomfort and anxiety/depression), each of which has five possible response levels: no problems, slight problems, moderate problems, severe problems and unable to/extreme problems. Based on the five responses across the five domains of the EQ-5D-5L classification system, a specific health state is defined. A utility score of one represents full health and a score of zero represents death, with it also being possible to have states worse than death as indicated by negative scores. Utility values were calculated using the mapping function developed by van Hout et al.,44 following the advice provided by NICE in its most recent position statement regarding analyses that use data gathered using the EQ-5D-5L.45
The raw EQ-5D-5L scores by domain are presented to examine the movements between levels for each domain according to study group. Descriptive statistics of the utilities for both groups are presented. The difference in utilities between the two groups for each time point have also been estimated. The overall difference in EQ-5D-5L index scores between the two groups were examined through regression methods, consistent with the model selected in the primary statistical analysis.
The cost–utility analysis was based on the difference in QALYs generated between both groups over the 12 months of the trial. For this, the utilities derived from the EQ-5D-5L were converted into QALYs for each participant using the area under the curve method, following the trapezium rule, which assumes linear interpolation between follow-up points.46,47 The difference in QALYs gained between the two groups were adjusted for baseline utility weights48 to allow for any differences between the two groups at baseline.
Health benefits in terms of falls
The mean number of falls participants experienced during the trial was also incorporated into the economic evaluation, thereby corresponding to the trial’s primary outcome, the number of falls per participant over the 12 months post randomisation. Estimates of the mean number of falls for the two groups were used to estimate the number of falls averted as a result of the OT intervention. This was based on the negative binomial regression model that was used for the primary statistical analysis; this estimated the number of falls per participant per year, adjusting for fixed effects of sex, age at randomisation, history of falling and the allocation ratio used for randomising participants, plus centre as a random effect.
Health-care resource use
Data regarding the use of health-care services during the 12-month duration of the study were collected for each participant, specifically health-care resource use in primary care and the community (i.e. GP, nurse, physiotherapist, OT visits) and the hospital setting (i.e. outpatient attendances, day-case attendances, number of nights spent as an inpatient and A&E attendances). The resource use data were collected from self-reported participant questionnaires at baseline and at 4, 8 and 12 months. Participants were asked to record their resource use according to that which was falls-related and that which was not related to falls. In the base-case analysis, only resource use items relating to falls were utilised, with non-falls-related items incorporated as part of a sensitivity analysis.
Participants were contacted after a reported fall so that further information could be collected regarding the type, location and cause of the fall. This information was recorded on a falls data collection sheet. In addition, participants were asked whether they had been injured by the fall; if they answered ‘yes’, they were asked whether they had spent time in hospital overnight as an inpatient. This method of data collection provided an alternative way of gathering data regarding participants’ falls-related hospital stays to the participant follow-up questionnaires. The inpatient stay data collected via participants’ falls data collection sheets were used for a sensitivity analysis, to investigate how the findings differed from the base case (i.e. which used inpatient stay data obtained from participant questionnaires). Based on the falls data collection sheets, a total of 56 participants (intervention, n = 13; usual care, n = 43) stated that they stayed in hospital overnight because of a fall, with some participants recording multiple occasions for overnight hospital stays due to a fall. Where participants had reported no hospital stay and had not given an answer to the question regarding how many nights they had spent in hospital (i.e. the box was left blank), this was assumed to indicate no use of services and, thus, no nights spent in hospital. Where participants reported that they had stayed overnight in hospital due to a fall but left the question about the number of nights spent in hospital blank, it was assumed that these participants had stayed in hospital for one night.
In the 12-month questionnaire, participants were asked whether or not they had any attendances at a falls service/clinic and whether or not they had received a falls prevention visit that was not part of OTIS, in the last 12 months. As part of the falls prevention home visit question, participants were asked to select who had undertaken the visit from the following options: OT, home carer or helper, district nurse, social worker, physiotherapist or ‘other’.
The secondary analysis conducted from a societal perspective included additional costs relating to participants’ private/personal expenses; participants were asked to state how much they had paid for any equipment or modifications made to their house, and for travel costs for health-care attendances. A question was also included in the questionnaires regarding whether or not participants had received any visits from paid care workers. Individuals may need to pay for all, some or none of the cost of paid care workers, depending on their circumstances.49 However, Age UK points out that it is rare for social care to be free.49 Where individuals do not pay for their care, social services can fund the visits, and how much individuals pay depends on capital, income, benefits and/or expenses.50 Paid care worker visits were assumed to be paid for by the participant/relatives, and therefore this fed into the societal perspective analysis rather than the base-case analysis, with this assumption explored via sensitivity analysis.
Resource use data are presented for both groups in terms of mean value, SD and mean difference (with 95% CIs) between the groups.
Costs
Costs of health-care services
Unit costs for health-care resource use items were obtained from established costing sources such as NHS Reference Costs 2017/1851 and the Unit Costs of Health and Social Care 2018 from the Personal Social Services Research Unit (PSSRU).52 The unit cost items used in the analysis are summarised and presented in Table 8. The year of pricing was set as the mid-year of the trial (i.e. 2017–18), based on the trial period of June 2016–19. All costs were evaluated in Great British pounds for 2017–18. Unit costs were multiplied by the corresponding resource use data to give an estimation of the total cost per participant.
TABLE 8
Unit costs of health and social care services
The total cost for each participant comprised the following elements: health-care resource utilisation (i.e. primary, community and secondary care visits and attendances) and the cost of the OT intervention (for those in the intervention group only). Further details of the intervention cost are provided in Costing the occupational therapist intervention section. Participants in the usual-care group received usual care from their GP and health-care professionals, and hence the cost of usual care incorporates the cost of this resource use. As the falls prevention advice leaflet produced by Age UK27 was provided to participants in both groups, it has been excluded from the analysis.
Costing the occupational therapist intervention
The cost of implementing and delivering the intervention was estimated based on information obtained from the OT home assessment visit that participants in the intervention group received, plus information regarding the cost of training the OTs to undertake the home visits. The intervention cost therefore comprised (1) the cost of OTs’ time spent on the home visit, including travel for the visit, and follow-up telephone call(s); (2) the cost of training the OTs; and (3) the cost of the equipment provided to the participant following recommendations from the OTs at the home visit. The three elements of the intervention cost are described in detail in the sections below, and the corresponding unit costs are summarised in Table 9.
TABLE 9
Unit costs of the intervention
Time spent by occupational therapists on the home visit and follow-up calls
The duration of the intervention home visit was recorded and used to cost the visit. When visit duration was missing, an average visit duration was applied. The OTs who were trained to undertake the home visits ranged from band 6 to band 8a; the duration of the visit in minutes was multiplied by a cost per minute, using the unit costs of time, for the particular OT who visited. That is, a unit cost of £0.73 per minute of band 6 time was applied when a band 6 OT undertook the home visit, and, similarly, a cost of £0.88 per minute was applied for band 7 OTs. One visit occurred where the OT name was not recorded, and hence an average unit cost per minute was applied. No band 8a OTs undertook the visits, but they were involved in the intervention training, including the running of cascade training. A travel cost of £5.60 was applied for each visit, based on a cost of 56 pence for each mile52 for an assumed 10 miles of travel. The duration of the follow-up telephone call and associated administration time was not recorded for each participant, but instead it was assumed to be 15 minutes based on feedback from study OTs who undertook the follow-up calls. This call duration was then also multiplied by the OT unit cost per minute.
Training
The total costs associated with training comprised the time OTs spent attending the training workshop and the associated materials (£8 per training pack and £15 for access to online training modules), plus the cost of the trainers’ time. Information regarding the time spent on the training workshops/modules and the staff grade of the trainees/trainers was collected by the trial team. Capital costs such as room hire, catering and travel costs for the training were not costed separately in the analysis because the intervention was delivered in an NHS setting and these overheads were included already in the OT staff costs. The costs associated with developing the intervention (i.e. preparing the training materials) were also not incorporated as these costs would not be incurred when rolling out the intervention.
Costs were attached to the training that was undertaken to enable the study OTs to conduct the OTIS home visits, with the opportunity cost of each of the 27 trainees included. Training costs were estimated according to whether the training was provided on a face-to-face basis or via cascade training; both trainee and trainer costs differed according to the method used. For the face-to-face training, a cost for the OT attendees’ time spent attending the training (7 hours) was multiplied by their corresponding hourly unit cost, according to their band. The cascade training assumed a similar time spent by trainees (7 hours) plus the time spent on completing the online training modules (3 hours), with the cost of the online training of £15 applied for each trainee. For the trainers who undertook the training, a cost of their time was incorporated: the face-to-face training was conducted over 4 days, with either a grade 7 or a grade 8a trainer cost applied depending on who ran each training day. Similarly, trainer costs were applied for those involved in conducting the cascade training (two grade 7 and one grade 8a), along with the cost of spending an additional 3 hours on the online training modules as a refresher prior to running the cascade training. In addition, the cost of training packs for each of the 27 trained OTs was included.
Equipment
Information regarding the items of equipment that were installed following the home visits was incorporated in the intervention cost. This information was collected during the 4- to 6-week follow-up call.
In England, equipment and home adaptations may be provided and paid for by local councils/social services, or it may be the case that the individual will need to instead pay a proportion or the full amount of the cost.53 The provision of equipment/adaptations depends on the type and cost of the item and the circumstances of the individual receiving them, that is, whether or not the individual meets the eligibility criteria for free provision. This takes into account the individual’s financial situation and whether they have an illness or a disability, with grants being available to apply for.54
The base-case analysis includes equipment that was provided by the NHS and PSS, but not equipment that was provided by the participant/relative; this does, however, feed into the secondary analysis, which takes a wider societal perspective. A sensitivity analysis was undertaken to explore what would happen if all of the equipment that was provided were to be funded by the NHS and PSS.
The unit costs for the various types of equipment were sourced from PSSRU’s Unit Costs of Health and Social Care 201852 and via the Living Made Easy website55 using the AskSARA guide.56 The guide provides information on daily living products and is governed by the NHS’s The Information Standard, a certification scheme for health and social care information. The cost of each equipment item is provided in Appendix 3.
Multiple imputation
Missing data are likely to arise in economic studies that involve the collection of patient-level data.57 Reasons for missing data include participants failing to complete certain items in the questionnaire, and participants failing to return the questionnaire at all. The total mean cost generated as part of an economic analysis often involves the summation of the costs of several resource use items. If responses to any of the items are missing, however, the overall cost would be considered missing if an approach such as complete-case analysis was utilised. This form of analysis would lead to meaningful data being excluded;58 there might be only a small proportion of missing resource use items, but a high proportion of individuals with missing total costs.57 Complete-case analysis is considered a useful starting point, although it is not recommended for use in the base case of a within-trial economic analysis.59 Available case analysis uses data more efficiently, although it also has limitations, for instance relating to the use of different samples for the costs and outcomes.59
Multiple imputation (MI) is an alternative approach to deal with missing data that relies on the assumption that data are missing at random; that is, the probability that data are missing is not dependent on the unobserved data, conditional on the observed data.60 MI estimates a set of plausible values for the missing data using the distribution of the observed data,60 with multiple ‘complete’ data sets created. Owing to the creation of multiple predictions for each missing value, MI incorporates the uncertainty in the imputations and provides accurate standard errors.61
Implementing MI with chained equations when data are not missing at random could result in biased estimates.61 Descriptive analysis of missing economic data was undertaken to determine whether or not the missing at random assumption underpinning MI was plausible. Missing economic data at all follow-up points for each study group and missing data patterns were explored. Logistic regression was used to assess the association between missingness and baseline variables, and the association between missingness and observed costs and EQ-5D-5L scores.
The findings of our investigations into missing economic data indicated that the missing at random assumption is plausible, as presented in more detail in Results. Therefore, the base-case analysis was conducted using MI for the handling of missing data. The model used MI with chained equations, with predicted mean matching on utilities at 4, 8 and 12 months and the cost estimates, and hence ensured that only plausible values were imputed. The imputation model included age, sex, centre, history of falling at baseline, utilities (at baseline and at 4, 8 and 12 months) and total costs at the resource use level (e.g. total cost of GP visits over 12 months). Three imputations are suggested to be sufficient for a data set that has 20% of total data missing.62 Owing to the extent of missing data in the present study, MI by chained equations was undertaken for a total of 10 imputations, with graphical plots used to compare the distributions of the observed data with the imputed data, thereby depicting whether or not the imputed data resemble the observed data. Mean cost and QALY estimates were generated using the combined imputed data sets following Rubin’s rules.63 The multiply imputed data set was used for the base-case economic analysis, with incremental cost-effectiveness ratios (ICERs) generated as described in Incremental analysis.
For comparison purposes, we conducted a complete-case analysis as a separate sensitivity analysis, in which only participants with observed data for all costs and utilities were included. As complete-case analysis relies on the data being not missing at random, the sensitivity analysis allows us to explore the impact of this alternative assumption around missing data. Available case analysis has been used for initial exploration of the data.
For participants who died during the study, usual imputation methods were applied when these participants had missing data before their death. For resource use and QALY data that would have been obtained via questionnaires received at any follow-up time point after their death, zero resource use and zero QALYs were assumed.
Incremental analysis
Costs and health outcomes (i.e. QALYs and falls) are summarised for both groups in terms of mean value and SD, and mean difference (with 95% CIs) between the groups. The analyses used conventional decision rules when comparing the mean costs and outcomes for both groups, with the results presented in terms of ICERs (i.e. the additional cost per extra unit of benefit), where appropriate. The ICERs of (1) the cost per fall averted and (2) the cost per QALY gained were estimated based on Equation 1:
where incremental costs and incremental effects are shown by ΔC and ΔE, respectively.
The ICER is compared with the willingness-to-pay threshold, i.e. the amount that a decision-maker is willing to pay for an additional QALY. A willingness-to-pay threshold of £20,000–30,000 has been used for the analyses, in line with NICE recommendations;42 that is, if the estimated cost per QALY is below the threshold, the intervention will be considered cost-effective. Interventions with an ICER < £20,000 would generally be considered cost-effective.
The findings are also presented in terms of the net monetary benefit (NMB),64 which involves translating the health benefits associated with the intervention into monetary terms and use of the cost-effectiveness threshold λ. If the mean incremental NMB (i.e. the difference in NMB between the intervention and usual care) is greater than zero, then the intervention is considered to be cost-effective at the threshold under consideration:
The NMB was estimated at a range of willingness-to-pay thresholds, in addition to the uncertainty around the net benefit estimates that is presented graphically using cost-effectiveness acceptability curves (CEACs), as detailed in Analysis of uncertainty.
QALY data were adjusted for baseline EQ-5D-5L scores48 to allow for any differences at baseline between the two groups. Adjustment was made for covariates consistent with those used in the primary statistical analysis. Differences between the groups were found to be statistically significant if the p-value was < 0.05 and are presented alongside CIs around the differences in costs and outcomes.
The cost-effectiveness analysis produced estimates of the mean differences in costs and outcomes using seemingly unrelated regression equations, with 95% CIs estimated using bias-corrected and accelerated bootstrap methods. Bootstrapping was undertaken for 10,000 replications, thereby generating 10,000 estimates of incremental costs and incremental effects, and presented on the cost-effectiveness plane. Regression methods have been used to take into account differences in stratification or prognostic variables, and other sources of heterogeneity. Analyses were conducted using Stata release 16 (StataCorp LP, College Station, TX, USA).
Analysis of uncertainty
The decision uncertainty around the cost-effectiveness estimates has been explored by investigating the probability that the OT intervention is cost-effective relative to usual care at a range of values that decision-makers are willing to pay to gain one additional QALY. As mentioned above, CEACs have been used to illustrate this uncertainty.65 Sensitivity analyses have been undertaken to explore the variability in estimating cost-effectiveness, as follows:
- complete-case analysis as an alternative to the use of MI for dealing with missing data
- inclusion of non-falls-related health-care resource use in addition to the falls-related resource use
- inpatient stay data from falls data sheets, rather than from participant-completed questionnaires
- exploration of the assumption that all equipment provided as part of the intervention is funded by the NHS and PSS (rather than in the base case, which attaches costs only to the items that were paid for by the NHS and PSS in the study and not to the items that were reported as funded by participants themselves, i.e. out-of-pocket expenditure)
- paid care worker visits being paid for by the NHS and PSS (rather than by the participant/relative as in the base case).
Extrapolation to the longer term
A further analysis using an exploratory decision model was also due to be conducted to explore the possible long-term impact of the trial assuming that a falls reduction leads to a reduction in fractures. However, owing to the study findings regarding falls (i.e. that the intervention was associated with an increased falls rate), it was not possible to extrapolate to the future in terms of a fracture reduction, and hence a long-term model has not been included in the economic evaluation.
Results
Participant population and missing data
A total of 35 participants died during the study period: 13 out of 430 (3.0%) in the intervention group and 22 out of 901 (2.4%) in the usual-care group.
The complete-case analysis (sensitivity analysis 1) included participants who had complete data for all costs and utilities over the entire study duration. A total of 412 participants had complete economic data: 121 intervention participants and 291 usual-care participants. The proportion of participants who had complete economic data available remained at similar levels over the course of the study; 54.4% and 55.5% of participants had complete data for the intervention and usual-care groups at baseline, respectively, compared to 55.6% and 56.8% at 12-month follow-up. Further details on the remaining time points are available in Appendix 5. For both groups, there was a higher number of complete cases at 4 months than at any of the other time points. The missing data patterns displayed for EQ-5D-5L and cost data are not monotonic; there are participants with intermittent missing data. For example, some participants were lost to follow-up at 4 months (i.e. had missing data), but had observed data at 8 months and/or 12 months. The use of complete-case analysis would therefore be inefficient due to discarding data from these individuals altogether, on the basis of having one or more missing data items.
The findings of the logistic regressions of indicators of missing cost and QALY data on study group allocation, several baseline variables and observed outcomes are presented in Appendix 6. Age, sex and lower EQ-5D-5L scores at baseline were significantly associated with missing cost data at 12 months. Baseline EQ-5D-5L score, age and history of falling were found to be significant predictors of missing QALY data at 12 months. Observed values for QALYs at both 4 months and 8 months were significantly associated with missingness of QALY data at 12 months, and also with missingness of cost data at 12 months. Observed costs at 4 months and 8 months were not found to be significant predictors of missing QALY data at 12 months or of missing cost data at 8 months. Observed costs at 4 months were, however, significantly associated with missingness of QALY data at 12 months. These findings suggest that the data are unlikely to be missing completely at random and support our assumption that data are missing at random.
Economic data were, therefore, assumed to be missing at random, with MI by chained equations used to deal with the missing data for the health economic analysis. Missingness was assumed to depend on baseline covariates (sex, age, history of falling and EQ-5D-5L at baseline) and observed costs and QALYs. The distributions of the imputed data were compared with the distributions of the observed data, as a means of checking the fit of the imputation model, with the distributions found to be similar for both costs and utilities (see Appendix 6).
Health-related quality of life
The EQ-5D-5L is categorised as complete if each of its five dimensions contains a response. The completion rates for the EQ-5D-5L were 93% for both groups at baseline (see Appendix 7), and, although the completion rates decreased by 12 months, they remained high, at 91% and 86% for the usual-care and intervention groups, respectively. Full details of the missing dimensions for the incomplete EQ-5D-5L questionnaires are provided in Appendix 8.
A breakdown of the different EQ-5D-5L levels (1–5) reported by participants, according to dimension, time point and group, can be seen in Appendix 9, for all available cases. Over 80% of participants reported having problems in terms of pain/discomfort at all time points in both groups, and problems with mobility and usual activities were experienced by at least 62% of participants. Fewer participants reported problems with the dimensions of self-care and anxiety/depression, with over 25% and 37% experiencing these at the study follow-up points.
The mean EQ-5D-5L visual analogue scale scores were found to be similar at baseline: 73.3 in the intervention group and 73.7 in the usual-care group (see Appendix 10). Scores reduced slightly in both groups over time, with 12-month scores being 71.5 for the intervention group and 72.1 for the usual-care group. Median visual analogue scale scores remained at 75 throughout the study for both groups at all time points.
Participants’ mean utility scores at each time point are summarised in Table 10 for all available cases, alongside the mean difference between the groups, both unadjusted and adjusted for baseline utility. At baseline, utilities were higher, on average, for usual-care participants (0.69) than for participants in the intervention group (0.68). After adjusting the mean difference for baseline utility, a marginally greater health-related quality of life gain (of 0.0003) was seen in the intervention group than in the usual-care group at 12 months. However, the difference in mean utility score was not statistically significant at any time point, whether unadjusted or adjusted for baseline utility (see Table 10).
TABLE 10
Summary of EQ-5D-5L utility scores and unadjusted and adjusted mean difference (95% CI) at each time point (all available cases)
In terms of the total QALYs gained by participants over the trial duration for all available cases (Table 11), no statistically significant differences were found between the groups, when adjusting for baseline utility only or when adjusting for baseline utility and all covariates. QALYs were found to be marginally lower for the intervention group (reduction of 0.0015 QALYs; p = 0.814) when adjusting for baseline utility and all covariates.
TABLE 11
Total QALYs for all available cases by allocation over 12-month trial duration and difference in mean QALYs (95% CI) (estimated using ordinary least squares)
Falls
At the primary end point of 12 months, participants in the intervention group experienced additional falls compared with those in the usual-care group (IRR 1.17, 95% CI 0.99 to 1.38; p = 0.07), as summarised in Chapter 3. As the intervention was associated with a higher falls rate (i.e. no falls were averted) and an additional cost, it was not clinically meaningful to calculate the ICER in terms of the cost per fall averted.
Health-care resource use and costs
The falls-related health-care services utilised by participants during the study period are summarised in Table 12. The resources participants used most commonly in relation to falls were visits to the GP, nurse and physiotherapist, in addition to hospital outpatient visits and inpatient overnight stays. The most notable difference between the groups was seen for inpatient hospital stays; participants in the intervention group stayed for an average of 0.05 nights over the past 4 months, when asked in the 12-month questionnaire, whereas participants in the usual-care group stayed for an average of 0.28 nights. Further resource use due to ‘other’ reasons is provided in Appendix 11. A greater proportion of missing data occurred for resource use related to falls than for ‘other’ resource use (i.e. non-falls-related). This applied for each resource use item, that is, for GP visits, nurse visits and all remaining resource use items, as shown in Table 12 and Appendix 11.
TABLE 12
Mean resource use, based on all available cases (falls-related only)
The proportion who attended a falls service or clinic over the 12 months was slightly higher in the intervention group than in the usual-care group, at 6% and 5%, respectively. A higher proportion of participants in the intervention group (19%) received a (non-study) falls prevention visit over the 12 months of the study duration, than in the usual-care group (9%). A possible reason for this could be participants misunderstanding the question and instead recording their OTIS visit. Based on the possible confusion by participants, we explored the impact of excluding the non-study falls prevention visits from the analysis, as summarised in Cost–utility analysis and uncertainty.
Unit costs have been attached to the resource utilisation from Table 12 to indicate the cost differences for each resource. The largest differences can be seen in the costs of inpatient hospital stays and day-case hospital visits, with the mean costs being lower for both of these for participants in the intervention group. The cost of physiotherapist and OT visits was higher for the intervention group, with only small differences seen for the remaining items. However, it is worth pointing out the high proportion of missing data for the majority of these items. Table 13 indicates the total mean costs for each resource use item over the 12-month follow-up period for all available cases. The corresponding mean costs for the multiply imputed data set is provided in Table 14. Total out-of-pocket expenditure that features in the societal perspective analysis can be seen in Appendix 12.
TABLE 13
Total mean costs of health and social care services (falls-related) and intervention cost based on all available cases, up to 12-month follow-up
TABLE 14
Total mean costs of health and social care services (falls-related) and intervention cost based on multiply imputed data set, up to 12-month follow-up
Costing the occupational therapist intervention
A total of 382 participants received the home visit as part of the intervention; the visit duration was recorded for 374 of these participants, with an average duration applied for the remaining eight participants for whom duration was not recorded. The mean intervention cost was found to be £137 for the 382 participants who received the home visit, which comprised the costs outlined in Table 15.
TABLE 15
Cost of the OT home visit intervention
The training cost per participant was estimated by dividing the total cost of training the OTs involved in the study by the number of participants who received the intervention. Of the 27 trained OTs, 23 conducted the 382 visits, seeing 16 or 17 participants on average. However, if the intervention were to be rolled out in practice, an OT is likely to see more people than this, and hence the cost of their training will be spread over more people.
The average equipment cost was relatively low; a high proportion of 71% (273/382) of participants did not receive any equipment/adaptations. Participants had a mean of 0.48 (SD 0.94) items installed, with this ranging from 0 to a maximum of 6 items installed per participant (and the median being 0). A breakdown of the mean number of the 31 possible equipment items installed is provided in Chapter 5 (see Table 22). In addition to these items, OTs had space to enter five ‘other’ items. An average cost for ‘other’ equipment was applied based on the five most commonly occurring equipment items (full details are in Appendix 4).
Cost–utility analysis and uncertainty
The OT intervention was associated with an additional cost for the base-case analysis, shown in Table 16, of approximately £19 per participant, on average. In terms of the effect of the intervention on health-related quality of life, only marginal differences in QALYs were demonstrated. The base-case analysis found 0.004 fewer QALYs for participants in the intervention group than for those in the usual-care group, on average, over the 12 months. It was not appropriate to report the base-case results in terms of the cost per QALY gained owing to the finding that usual care was the dominant option; that is, it was less expensive than and generated additional QALYs to the intervention, although this QALY gain was marginal.
TABLE 16
Summary results for incremental analysis, cost-effectiveness and uncertainty: for the base-case analysis and sensitivity analyses
The NMB at £30,000 per QALY was –£145.31 (95% CI –£149.90 to –£140.72), which also indicates that the intervention is not a cost-effective option when compared with usual care. The NMB at £20,000 per QALY of –£103.13 (95% CI –£106.73 to –£99.53) similarly shows that the intervention is not cost-effective when this lower threshold is considered.
Figure 9 illustrates the 10,000 bootstrap sample estimates, which are spread across the four quadrants of the cost-effectiveness plane, although the north-west and south-west quadrants are more heavily populated than the remaining two quadrants. The probability of the intervention being cost-effective at different willingness-to-pay thresholds is shown in Figure 10. At the lower NICE threshold of £20,000 per QALY, the probability of the intervention being cost-effective is 29.1%, and is similar at 26.9% for a threshold of £30,000 per QALY (under the base-case scenario).
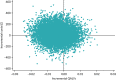
FIGURE 9
Cost-effectiveness plane for the base-case analysis: NHS perspective (MI adjusted for all covariates).

FIGURE 10
Cost-effectiveness acceptability curve for the OT intervention relative to usual care, for the base-case analysis: NHS perspective (MI adjusted for all covariates).
The secondary analysis undertaken from the societal perspective, which also included indirect costs rather than just considering the costs borne by the NHS and PSS under the base case, found an incremental mean cost per participant of £73.15 (95% CI £68.41 to £77.89). A reduction in QALYs (–0.0025, 95% CI –0.0026 to –0.0023) was again demonstrated for the intervention group versus the usual-care group. The cost-effectiveness plane displaying the bootstrap sample estimates for the secondary analysis is provided in Appendix 13. The corresponding CEAC (see Appendix 13) depicts a 34% chance of the intervention being cost-effective at the £30,000 per QALY threshold.
Sensitivity analysis
The findings for each of the sensitivity analyses are shown in Table 16 in terms of the incremental mean costs, the incremental mean QALYs, the ICER and the probability of the intervention being cost-effective. For all sensitivity analyses, the QALYs remained relatively similar, with the exception of sensitivity analysis 1, which comprised the complete-case analysis. We therefore focus on the cost findings here. When only complete cases were included (sensitivity analysis 1), the intervention was found to be the dominant option compared with usual care. Additional QALYs were experienced by the intervention group, on average, although this QALY difference was found to be small (0.0076) and not statistically significant. This sensitivity analysis found a cost saving of £69 for the intervention group, in contrast to the additional costs demonstrated in the base case. However, the complete-case analysis comprised only 412 participants. The corresponding cost-effectiveness plane (Figure 11) demonstrates the QALY gains, with more of the point estimates being situated in the north-east and south-east quadrants. The CEAC for the complete-case analysis can be seen in Figure 12. The cost-effectiveness planes and CEACs for sensitivity analyses 2–5 are provided in Appendices 14 and 15, respectively.
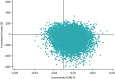
FIGURE 11
Cost-effectiveness plane for the complete-case analysis (NHS perspective, adjusted for all covariates).
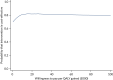
FIGURE 12
Cost-effectiveness acceptability curve for the complete-case analysis (NHS perspective, adjusted for all covariates).
In the base-case analysis, the intervention was dominated by usual care, as the intervention generated additional costs and fewer QALYs. The intervention remained dominated for both sensitivity analysis 3 (alternative source of inpatient stay data) and sensitivity analysis 4 (all equipment funded by NHS and PSS). Sensitivity analysis 3 explored the use of an alternative source of data for the number of nights spent in hospital due to falls. When the falls data sheets were used instead of the follow-up questionnaires to obtain data on falls-related nights spent in hospital, additional costs were found for the intervention group, which in turn led to an additional mean cost of £120 per participant. Sensitivity analysis 4 investigated the findings when all the equipment installed following the OTIS home visit was funded by the NHS and PSS. As expected, because the equipment component of the intervention cost increased, an overall increase in the mean cost of £40 was found. For the analysis exploring the inclusion of non-falls-related resource use (sensitivity analysis 2) and the analysis of paid care worker costs being included (sensitivity analysis 5), the intervention group experienced an average cost saving of £204 and £55, respectively. The corresponding ICER results were presented in terms of the cost per QALY lost, whereby QALYs are being lost but costs are being saved. The ICERs here indicate how much would need to be saved to justify a loss of one QALY.
The effect of removing the non-study falls prevention visits from the analysis, owing to participants’ potential misunderstanding of these, was also explored; the overall cost saving was £68.28 per participant (as opposed to an additional cost of £18.78 in the base case).
Discussion
The within-trial cost–utility analysis findings indicate that the OT intervention was associated with slightly higher costs (£18.78, 95% CI £16.33 to £21.24) and generated marginally fewer QALYs (–0.0042 QALYs, 95% CI –0.0043 to –0.0041 QALYs) and additional falls over the 12 months’ study duration, when compared with usual care. However, the cost and QALY differences between the two groups were small. The cost-effectiveness plane indicated dispersion of the incremental costs and incremental QALYs, with the point estimates featuring in all four quadrants, further highlighting the uncertainty around the findings.
The base-case analysis, which took an NHS and PSS perspective and used MI to take account of the large proportion of missing economic data, found a 27% probability that the intervention was cost-effective, using a willingness-to-pay threshold of £30,000. The finding of additional costs and fewer QALYs for the intervention group indicates that the intervention was dominated by usual care and is, therefore, unlikely to provide a cost-effective use of NHS resources when compared with usual care.
For the secondary analysis conducted from a societal perspective, the intervention group again experienced higher costs and fewer QALYs than the usual-care group, and hence the intervention was dominated by usual care. It is worth noting that the societal perspective considered the key costs expected to be incurred by participants, and, owing to concerns about overburdening participants, the questionnaires did not provide an exhaustive list of items. We acknowledge that it would have been useful to collect information on whether the paid care worker visits participants reported were paid for by them or by social services.
The results remained robust under some of the sensitivity analyses undertaken to explore the use of different assumptions, although some of the analyses did demonstrate different findings. The complete-case analysis of 412 participants indicated the intervention to be a cost-effective option, as it dominated usual care in terms of finding a reduction in costs and an improvement in QALYs, although these differences were not statistically significant. However, for all other analyses, a mean loss in QALYs was found for the intervention group, although, again, these were not statistically significant. Cost savings arose when non-falls-related resource use was incorporated and when the cost of paid care worker visits was included. Higher costs were seen for the intervention group with the sensitivity analysis that used alternative data regarding hospital inpatient stay, and under the scenario in which all equipment provided as part of the OT intervention was funded by the NHS and PSS.
The breakdown of the EQ-5D-5L data collected for this population for the four time points was useful to investigate. Participants in both groups were found to have a marginal fall in utility over the course of the trial, perhaps indicating that health-related quality of life diminishes slightly over time for the elderly population included in the study.
In addition to potential recall issues for resource use questions in terms of participants possibly not remembering the details of a visit that happened a few months ago, participants may have struggled to decide whether their resource use related to a fall or an ‘other’ reason when completing their study questionnaires. The larger number of missing data for falls-related resource use could indicate that participants missed these questions out because they were not sure whether the visits were in relation to a fall, but instead found it easier to respond regarding visits that were due to ‘other’ reasons. It could, therefore, be considered a limitation to ask participants to assign their service use in this way because of the increased likelihood that data on the falls-related option will be missing.
The costs were heavily driven by the cost of hospital inpatient stays, hospital day-case visits and outpatient attendances, with A&E attendances, physiotherapist visits and GP visits also factoring to a lesser extent. The intervention cost of £137 inevitably was a prominent additional cost incurred by participants in the intervention group. The key cost drivers of the intervention were the OT time cost attached to the home visit and the training cost element. However, the training cost per person who receives a home visit will reduce with the number of people seen by a trained OT, as the initial training cost is then spread over more people. A point to note regarding the intervention cost relates to the possibility of OTs spending further time after the home visit finishing off their paperwork, which has not been included in the costs; the analysis assumes that all study forms were completed at the home visit as outlined to OTs in the initial study training. Another potential source of additional OT time is the time taken to make referrals. We did not collect information on the number of participants who received a referral following the home visit, and therefore this was not incorporated in the intervention cost, but we acknowledge that it would have been useful to capture such information.
The equipment costs were estimated based on the total cost of the equipment items under consideration. We recognise that such an approach will attach higher costs than if the mean annual cost for equipment had been applied. However, we took this approach because this represented the cost incurred by the NHS and PSS during the time horizon of the study. It also removed the need to make assumptions about the lifetimes of equipment and whether some equipment items may, in practice, be used in only a limited timeframe, less than their expected lifetime. A further point to raise around equipment costs relates to the sources used for these costs; we obtained costs for equipment/adaptations from PSSRU’s Unit Costs of Health and Social Care in the first instance. Where information was not available on particular items, we sourced this from AskSARA,56 which includes retail prices paid by individuals. Although we acknowledge that the use of different sources is not ideal, the aim of our approach was to obtain costs representative of those paid in practice, making the best use of the information available.
The original intention in developing a long-term model was to use the results of the trial, supplemented with data taken from the literature, where required, to extrapolate an improvement from the intervention in terms of falls reduction to a reduction in fractures in the longer term. However, given the finding of the trial, namely that intervention effects were not present, an extrapolation analysis using a long-term model was not considered to be meaningful.
In conclusion, the intervention was associated with additional costs, fewer QALYs and additional falls compared with usual care over the 12 months’ study duration. On this basis, the intervention would not be recommended from an economics perspective as it does not provide a cost-effective option when compared with usual care. However, the differences between groups were small, and findings changed when different assumptions were explored via sensitivity analyses.
- Economic evaluation - Home environmental assessments and modification delivered ...Economic evaluation - Home environmental assessments and modification delivered by occupational therapists to reduce falls in people aged 65 years and over: the OTIS RCT
- Danio rerio cereblon, mRNA (cDNA clone MGC:92404 IMAGE:7037986), complete cdsDanio rerio cereblon, mRNA (cDNA clone MGC:92404 IMAGE:7037986), complete cdsgi|51330678|gb|BC080253.1|Nucleotide
- Initiating change locally in bullying and aggression through the school environm...Initiating change locally in bullying and aggression through the school environment (INCLUSIVE): a pilot randomised controlled trial
Your browsing activity is empty.
Activity recording is turned off.
See more...